New network model efficiently identifies cancer driver genes
Posted: 16 September 2024 | Drug Target Review | No comments yet
The model enables researchers to expand the number of tumours that they can study, increasing their ability to detect novel interactions.
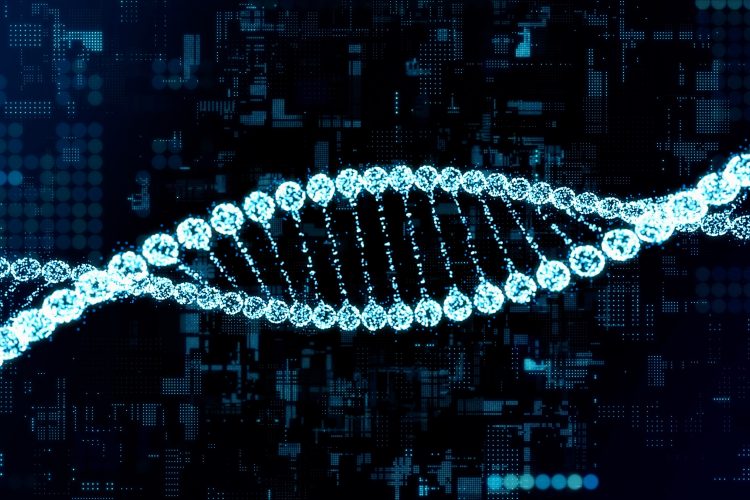
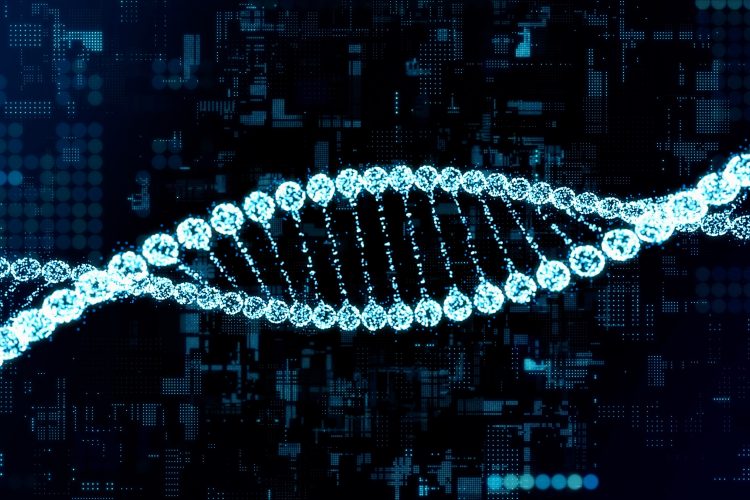
Researchers from Washington State University (WSU) and the University of Vermont have developed a new network model, named DiWANN, to more efficiently identify genetic mutations that work together to drive cancer, compared to existing network models. Significant findings from this could be used to develop novel cancer therapeutics.
The DiWANN model was developed five years ago and has been used by the team to investigate both the spread of COVID-19 and the geographic distribution of tick-borne diseases. For the new study, the scientists added a data reduction step to lessen the amount of required computation, as well as a second computer model to further explore co-occurring genes.
Corresponding author Assefaw Gebremedhin, associate professor at WSU School of Electrical Engineering and Computer Science, stated: “This is a study not of one particular cancer, where you dive in and try to understand it, but rather of many cancers together, looking for patterns and things that could someday be used for drug discovery.”
The team confirmed that two mutations in pancreatic cancer, tumour protein 53 and KRAS, almost always appear together. Also, the scientists found cancer types that are closely connected to each other and that could be susceptible to common drug treatments. Some cancers were homogenous, but other types had a huge variety of different mutations, which are likely to be difficult to treat.
Moreover, the model enables researchers to expand the number of tumours that they can study. Steven Roberts, molecular genetics researcher at the University of Vermont and co-author, explained: “This increases our power to actually detect novel interactions and novel aspects of how these tumours behave…If we can actually screen through large data sets, it’s a much faster way to go about it.”
Improving the understanding of how common different mutations and driver genes are across different cancer types could aid the prioritisation of drug targets. However, this has been challenging due to the large amount of computation needed to study long genetic sequences and a high number of mutations. Gebremedhin highlighted: “Our model tries to understand relationships between sequences in a more effective way, and by effective, I mean they can be computed quickly. With this minimal representation of the network, you can get more information and make computation scalable.”
Now, the team are aiming to develop a web-based tool for public health experts, so that experts can more easily use the model to study complex questions in cancer and other diseases.
This study, supported by the National Science Foundation and the National Cancer Institute, was published in Frontiers in Bioinformatics.
Related topics
Artificial Intelligence, Cancer research, Disease Research, Genetic Analysis, Oncology, Toxicology
Related conditions
Cancer Research, Pancreatic cancer
Related organisations
University of Vermont, Washington State University (WSU)