Overcoming operational challenges in CGT manufacturing with AI
Posted: 18 September 2024 | Dalip Sethi (Terumo Blood and Cell Technologies) | No comments yet
In this Q&A, Dalip Sethi, scientific lead for Terumo Blood and Cell Technologies, Cell Therapy Technologies and Innovation portfolio, discusses the integration of AI into CGT manufacturing processes to enhance operational efficiency and accelerate treatment access. He also elucidates the strategies that are being considered to overcome hurdles when implementing AI solutions.
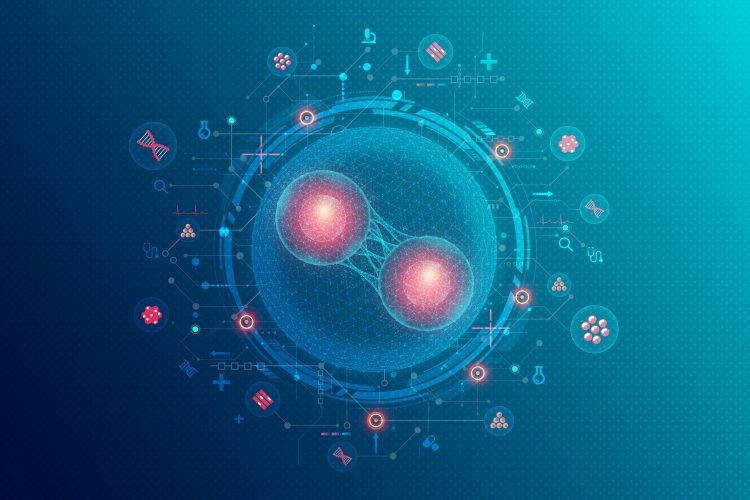
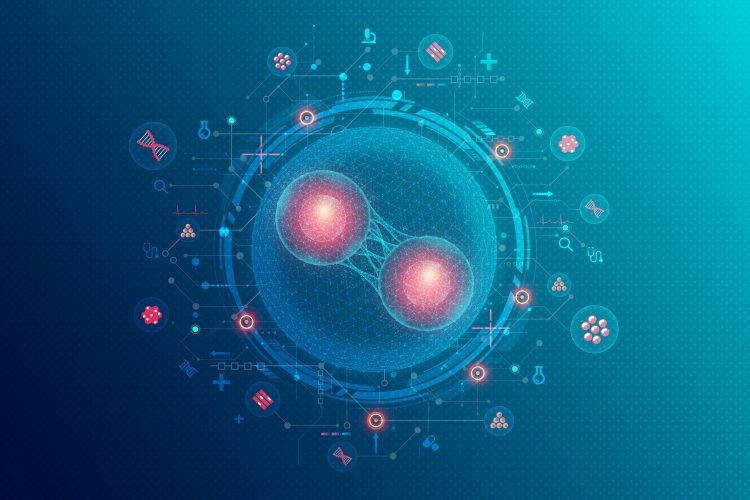
What specific cost-cutting measures can AI provide in the manufacturing process of cell and gene therapy, and how do these compare to traditional methods?
The cell and gene therapy (CGT) space provides a great example of the potential of AI. The future of medicine is being shaped by groundbreaking CGTs, which involve uniquely complex manufacturing steps. Because they are mostly personalised medicines today, production is limited to one patient dose at a time and slowed by cumbersome and largely manual processes. In addition to these inefficiencies, the field is still just learning which manufacturing parameters are most critical to patient outcomes, and how to optimise them.
AI can vastly improve cell culture, a critical step in cell therapy production. It is a tedious and complex process, and each subtype within the diverse set of cell therapy applications utilises cell culture under a unique set of conditions. The data collection and analysis for this step is centered on the utilisation of biosensing tools in order to monitor the cell culture environment. Using the data from cell culture parameters, the industry is beginning to understand the applications of machine learning (ML).
How is AI being integrated into current cell and gene therapy manufacturing processes to enhance operational efficiency and accelerate treatment access?
At the recent ISCT 2024 – Signature Series, researchers from Charles River Laboratories presented a study1 to predict the optimal cell culture parameters using a ML model. The authors were able to achieve high cell densities, exceeding 50 million cells/mL, in a stirred tank bioreactor. In the study, the ML analysis discovered a metabolic model to decrease the use of process reagents, leading to a reduction in the cost of goods of 35 percent to 50 percent, all while keeping T-cell densities high. The study demonstrates the application of these advanced tools when the biosensor data is available. Similar concept can be used with perfusion-based, hollow fibre-based systems such as Quantum Flex Cell Expansion system. In summary, as the utilisation of biosensing tools becomes prevalent, the industry should benefit from enhancement in operational efficiencies, using computational models.
What are the primary data challenges faced by the cell and gene therapy industry when implementing AI solutions, and what strategies are being considered to overcome these hurdles?
Over the last decade, the cell and gene therapy field has moved towards automated unit operations and enhanced digital data capture capabilities. Though advanced automated unit operations are available, adopting these technologies in manufacturing could be faster.
Early-stage developers generally rely on manual processes with limited digital data capture. Manual data needs to be digitised, which may require more than easy transfer and integration among the manufacturing steps. The industry is getting educated on the importance of automated unit operations and availability of digital process data. Companies are planning their drug development strategies with automated unit operations in mind, and how to incorporate them earlier in the development pipeline.
Another challenge is the availability of large data sets for training the models. As more patients are treated, more data would be collected, and bigger data sets would be available.
Today, data tends to be siloed out of an abundance of caution related to intellectual property concerns. It’s a challenge throughout biopharma, but CGT developers in particular think of the process as the product. The concerns are legitimate, and there are also ethical questions related to patient privacy and AI usage, as well as evolving regulatory considerations.
However, the whole field is incentivised to work through these issues because democratisation of data will be crucial. As an enabling technology company, we are in a unique position to have a central role in supporting our partners’ data housing and integration, allowing them to do complex analyses and then leverage that with AI to augment decision making. We are pursuing and encouraging more data partnerships to enable the required openness.
Can you provide examples of efforts within the life sciences sector aimed at overcoming operational bottlenecks in cell and gene therapy manufacturing using AI?
For most companies in the space, AI is a nascent technology and so they have yet to develop the in-house expertise to prepare a transition to the AI future. This puts the emphasis on partnerships. For example, we have sought to boost the data generation capabilities of our Quantum Flex Cell Expansion System by working with companies that specialise in biosensing technology. Connecting the system with biosensors enables on-line monitoring of critical process parameters. The combination allows collection of metabolic data, such as lactate generation and glucose consumption, during the cell culture. The data in turn could be used to predict the number of cells during the culture, and an appropriate time to harvest the cells.
What role do data readiness and quality play in the successful deployment of AI in cell and gene therapy, and what steps are being taken to ensure these prerequisites are met?
Capturing process data and making it available in a consistent format is the key step for enablement of computational analysis and modelling. As a field, we are still determining which of the many parameters we can measure and control during cell culture are most relevant. Researchers need to collect more data on these parameters to train the prediction models, and it needs to be structured in a way that can allow comparisons between platforms and across cell types. As data of a sufficient quality becomes available, it can enable the ML models to optimise cell culturing systems in different bioreactor systems.
Reference
1 Campion, S, et al. Machine learning enables high density T-cell expansion with lower costs while maintaining product quality. Cytotherapy. June 2024; 26(6). Available from: https://www.isct-cytotherapy.org/article/S1465-3249(24)00130-0/abstract
About the author
Dalip Sethi
He holds a doctorate and conducted post-doctoral studies at Thomas Jefferson University, School of Medicine. In his post-doctoral research, Dalip focused on the development of cancer gene-specific RNA and DNA analogs targeted against cancer genes in the signal transduction pathway for use as cancer diagnostics and therapeutics. Throughout his career in the industry, Dalip has been engaged in developing technologies and methods for use in cell therapy applications.
Dalip has authored multiple scientific publications and is a co-inventor on several patents and patent applications. He recently co-authored publications on modular automated systems for CD3+ T-cell manufacturing and monoculture of cord-blood derived CD34+ using an automated, membrane-based dynamic perfusion system. The articles highlighted the benefits of modular automation in cell therapy manufacturing. Dalip is also an ISCT member and participates in committees focused on cold chain, particulates, and process analytical technologies.
Related topics
Artificial Intelligence, Cell Therapy, Gene Therapy
Related organisations
Terumo Blood and Cell Technologies
Related people
Dalip Sethi (Terumo Blood and Cell Technologies)