Computational pipeline for personalised cancer vaccine design
Posted: 16 October 2024 | | No comments yet
Ludwig Cancer Research researchers have designed and validated a computational pipeline which integrates molecular and genetic analyses.
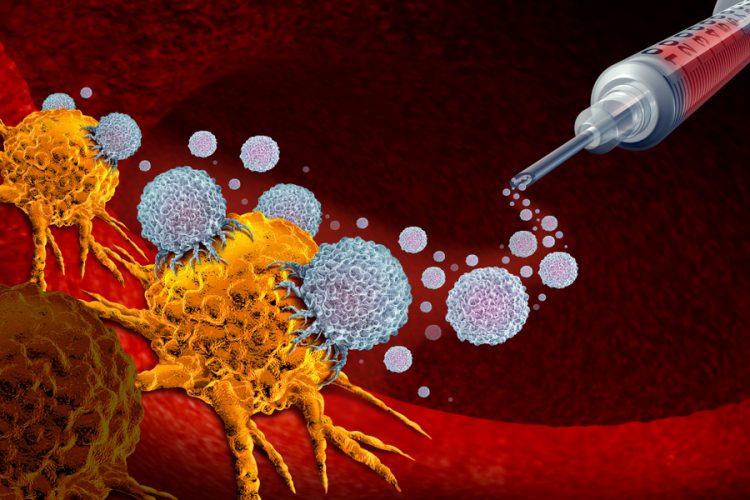
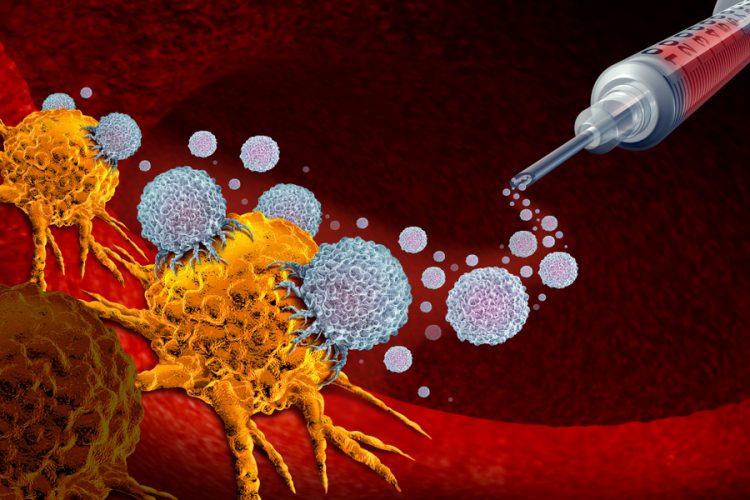
Researchers at Ludwig Cancer Research have developed a full computational pipeline to design personalised cancer vaccines. Led by Florian Huber and Michal Bassani-Sternberg, it integrates molecular and genetic analyses of tumours and specific molecular targets of T cells, using AI algorithms.
Led by Florian Hiber and Michal Bassani-Sternberg, the design, validation and comparative assessment of ‘NeoDisc’ was conducted. Bassani-Sternberg stated: “NeoDisc provides unique insights into the immunobiology of tumours and the mechanisms by which they evade targeting by cytotoxic T cells of the immune system…These insights are invaluable to the design of personalised immunotherapies, and the analytical and computational pipeline at the heart of NeoDisc is already being used here in Lausanne for clinical trials of personalised cancer vaccines and adoptive cell therapies.”
Neoantigens
Several cancers have random mutations that should make them more visible to the immune system. These mutations produce unusual proteins that cells are programmed to cut into peptides and present as antigens.
One of the reasons why patients respond variably to immunotherapies is due to the diversity of neoantigens. Neoantigens can be used to develop vaccines and other types of immunotherapies tailored to target each patient’s tumours. However, these efforts are technically challenging because not all neoantigens are recognised by a given patient’s T cells. Moreover, many that are recognised do not trigger a potent T cell attack. Therefore, one approach to creating personalised vaccines and cell therapies requires the identification of neoantigens most likely to activate a strong T cell attack.
This needs a large-scale analysis of mutations that generate potential neoantigens, the HLA molecules that presents them to T cells and the molecular characteristics that enable recognition by T cell receptors. Bassani-Sternberg is among the pioneers of this “immunopeptidomics” field.
Also, the design of personalised immunotherapies is supported by genomic analysis of the tumour and blood cells that represent the healthy genome of the patient; the large-scale analysis of transcriptomics, and the analysis of the immunopeptidome with mass spectrometry. However, until now, these technologies have never been integrated in a single computational pipeline to predict which neoantigens found in a patient’s tumours should be used as vaccines or harnessed for personalised immunotherapies.
Tumour-specific antigens
Furthermore, tumour-specific antigens such as aberrantly expressed gene products and viral antigens are available for immunotherapeutic targeting. Huber commented: “NeoDisc can detect all these distinct types of tumour-specific antigens along with neoantigens, apply machine learning and rule-based algorithms to prioritise those most likely to elicit a T cell response, and then use that information to design a personalised cancer vaccine for the relevant patient.”
“Notably, NeoDisc can also detect potential defects in the machinery of antigen presentation, alerting vaccine designers and clinicians to a key mechanism of immune evasion in tumours that can compromise the efficacy of immunotherapy,” added Bassani-Sternberg. “This can help them select patients for clinical studies who are likely to benefit from personalised immunotherapy, a capability that is also of great importance to optimising patient care.”
Moreover, the study demonstrates that NeoDisc provides a more accurate selection of effective cancer antigens for vaccines and adoptive cell therapies compared to other computational tools currently used for that purpose.
Moving forwards, the team aim to improve NeoDisc’s training and predictive accuracy by feeding it data obtained from multiple tumours and integrate additional machine-learning algorithms to the software suite.
This study was published in Nature Biotechnology.
Related topics
Artificial Intelligence, Cancer research, Computational techniques, Immunotherapy, Personalised Medicine, T cells, Vaccine, Vaccine development
Related conditions
Cancer
Related organisations
Ludwig Cancer Research