The paradox of data in precision medicine
Posted: 28 November 2024 | Stavros Papadopoulos | No comments yet
The path to faster breakthroughs in precision medicine begins with overcoming the complexities of multi-modal data. Discover how innovative solutions are enabling more personalised treatments.
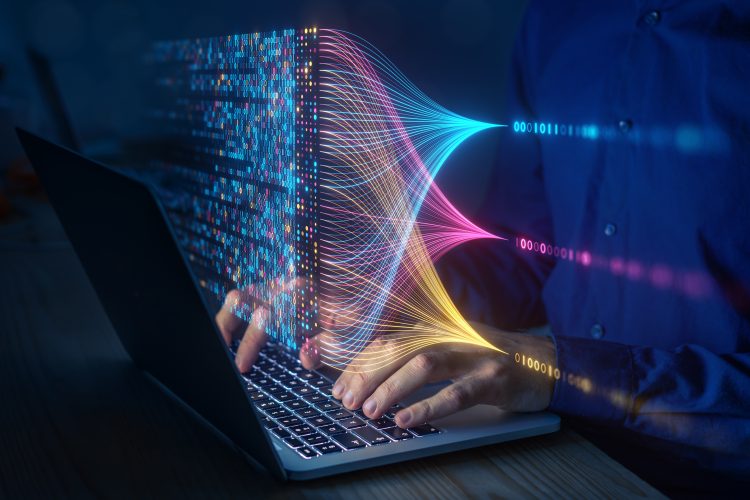
As the journey toward realising the full potential of precision medicine continues, one question frequently arises among pharma executives and biotech founders: why is progress so slow? A significant part of the answer may lie in the limitations of current technology in handling complex, multi-modal data – data that is crucial for advancing drug discovery and identifying new targets.
In many cases, life sciences organisations are using technologies that were not specifically designed for scientific data, leading to inefficiencies in both research and operations. Below are three key challenges that need to be addressed to overcome this technological bottleneck.
Challenge 1: The diversity of life sciences data
The diversity of life sciences data is vast, encompassing everything from traditional formats like text files, tables and PDFs to more complex ‘frontier’ data, such as population genomics, single-cell data, bioimaging and various forms of multi-omics data.
The data is further categorised into ‘structured’ data, which typically includes tabular data and ‘unstructured’ data. This includes everything from text files and images to multimodal, multi-omics data, as well as ‘real-world’ data, such as electronic health records and disease registries.
According to estimates, up to 80 percent1 of data in the life sciences industry is unstructured and comes from diverse sources, making it difficult to analyse and use. Despite its vast potential, only 12 percent of this unstructured data is currently analysed, leaving vast amounts of data untapped.2
In its entirety, life sciences data is an asset that fosters innovation and often provides organisations with a significant competitive edge
In its entirety, life sciences data is an asset that fosters innovation and often provides organisations with a significant competitive edge. However, current systems are inefficient in managing it, especially when it comes to frontier data. As a result, organisations are left to adopt bespoke solutions for each data type, ultimately resulting in convoluted, hard-to-manage data infrastructures and out-of-control licensing and operational costs. Specifically, to address these challenges, organisations often adopt technologies such as databases, file managers, data catalogs and specialised scientific platforms. But standalone, such technologies cannot fulfil the requirements of organisations focused on discovery.
For instance:
- Database technology has the power to model and analyse data securely and efficiently. However, the vast majority of databases can handle only structured, mainly tabular data. Despite its importance, tabular data accounts for only a small fraction of the scientific data within most organisations. Force-fitting non-tabular data into a tabular database most often results in poor performance.
- File managers can store any type of data in binary files. However, they do not provide any context, semantics or specialised metadata about the underlying data modalities, which makes it very difficult to search and locate data relevant to a specific scientific workflow.
- Data catalogs add more meaningful information about the data they are cataloguing, exposing important relationships across the different data types and making searches far more effective. However, data catalogs do not have the computational power and scalability of databases.
- Scientific platforms are specialised for the scientific domain, offering deep understanding and functionality around the data modalities used in the life sciences industry (which may include features such as database, file management and data catalog functionality). However, these solutions are not designed to function as powerful database systems, which becomes evident when dealing with complex and demanding data modalities (such as genomics and single-cell data).
The extreme fragmentation across various solution types makes it almost impossible to effectively catalogue and search through the diverse data generated by different teams and roles. Additionally, there is a wide range of technical expertise across scientific disciplines; thus, while a bench scientist might have some familiarity with coding in R or Python, it is unlikely that a geneticist or cell biologist would want to focus their time on coding tasks rather than their core scientific work.
Organisations desperately require a way to catalogue centrally – storing and managing all their data and code types, including tables, text files, multi-omics, metadata, machine-learning models, Jupyter notebooks, user-defined functions, and more. When companies make it easier to search across all these different data formats, their teams can become far more productive in their everyday work.
Challenge 2: Life sciences data is often privileged or proprietary
Data privacy and security are paramount when dealing with sensitive data, such as patient records governed by HIPAA or other regulatory frameworks. Collaborating on this data, whether internally or across organisations, requires secure systems that facilitate safe data sharing without costly or time-consuming data transfers.
But, why is this so important? Having multiple data silos for various data types necessitates the work of a large team to harmonise the use of all these systems, ensuring secure and compliant collaboration. This, combined with skyrocketing licensing costs due to the use of duplicate systems offering the same functionality, can quickly lead to major cost overruns and reduced efficiency.
A new, single, centralised source of truth must be created, with security, governance and compliance as top priorities, serving as a trusted research environment. Ideally, this platform should be SOC 2 Type 2 and HIPAA-compliant and undergo constant penetration tests by third-party auditors. In addition to access control, the platform should include detailed logging features and record all activities on all assets by all users, as well as provide robust auditing capabilities essential for security. Finally, organisations should have the ability to self-host the platform in their own private environments, which helps enforce yet another security layer to protect sensitive research and data.
Challenge 3: Frontier data is large and computationally demanding
Consider single-cell data: As the number of datasets continues to grow, mapping new data to curated reference atlases holds enormous promise for advancing precision medicine. However, single cells make big data, and these large, unstructured datasets often end up in complex file formats that impede performance and slow down the discovery process.
Simply cataloguing complex data without the ability to analyse it quickly creates a significant architectural gap that hinders discovery
Simply cataloguing complex data without the ability to analyse it quickly creates a significant architectural gap that hinders discovery. It is possible to structure complex data modalities to improve performance, even on a single machine. This can be done by adopting shape-shifting multi-dimensional arrays, which adapt to any data type and transform unstructured data into structured data. The benefits multiply further when hard computations are scaled from just a few CPUs to hundreds or even thousands of machines. A new approach offers scalable computing, eliminating the need to manually configure and launch large compute clusters, which can slow down time-sensitive computations and discoveries if not managed correctly. Additionally, improperly utilising large compute clusters can lead to significant increases in overall costs.
In summary, a new approach is needed to manage life sciences data; one that offers numerous benefits to organisations. These include:
- Faster time to insights – making complex data accessible to scientists and enabling collaboration accelerates breakthroughs
- Simpler infrastructure – a unified, scalable and collaborative platform for all data types eliminates the need for multiple software tools and complex infrastructures
- Less engineering – a single platform that handles data modelling, ingestion, fast analysis and governance, significantly reducing the engineering effort
- Unprecedented speed – built on cutting-edge database technology offering unmatched speed and scalability
- Greater economies of scale – significant cost savings through improved performance, omni modality support and reduced engineering effort.
Life science leaders in pharma and biotech face significant challenges with scientific data, chiefly its diversity, complexity, governance needs, and the heavy demands for computational analysis. This raises an important question: does life sciences require a new system of record? The future of precision medicine is counting on it.
About the author
Stavros Papadopoulos, Founder and CEO of TileDB, Inc.
Before founding TileDB, Inc. in February 2017, Dr Stavros Papadopoulos was a senior research scientist at the Intel Parallel Computing Lab, and a member of the Intel Science and Technology Center for Big Data at MIT CSAIL for three years. He also spent about two years as a visiting assistant professor at the Department of Computer Science and Engineering of the Hong Kong University of Science and Technology (HKUST). Stavros received his PhD degree in Computer Science at HKUST under the supervision of Prof Dimitris Papadias, and held a postdoc fellow position at the Chinese University of Hong Kong with Prof. Yufei Tao.
References
- Pharmalive. Decoding Health: Transforming 80 Percent of Unstructured Data into Insights with NLP [Internet]. Available from: https://www.pharmalive.com/decoding-health-transforming-80-percent….)
- Data Dynamics Inc. The Untold Story of Big Pharma’s Unstructured Data Crisis and How to Solve It [Internet]. Available from: https://www.datadynamicsinc.com/blog-the-untold-story-of-big….)
Related topics
Drug Development, Drug Discovery, Genomics, Machine learning, Personalised Medicine, Proteomics
Related organisations
TileDB
Related people
Stavros Papadopoulos