AI-powered imaging for faster lung disease treatment
Posted: 22 April 2025 | Muhunthan Thillai (CEO - Qureight) | No comments yet
Deep learning is transforming lung disease treatment by enabling earlier diagnosis, precise monitoring and more efficient clinical trials. This article explores how AI-driven imaging is accelerating drug development and improving patient outcomes.
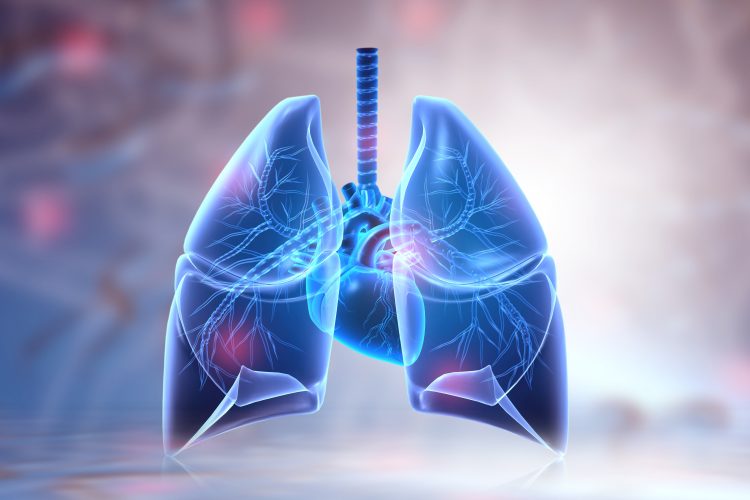
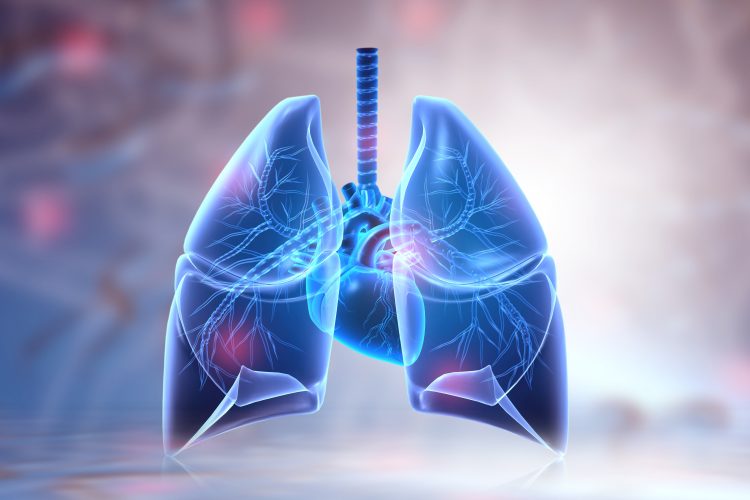
Artificial intelligence (AI) is transforming the landscape of modern medicine, with deep learning at the forefront of this revolution. Deep learning (DL) rapidly analyses complex medical data, identifying patterns in diseases with subtle, progressive symptoms such as respiratory conditions where early detection is crucial.
Among these conditions is idiopathic pulmonary fibrosis (IPF), which poses a significant health challenge due to its unpredictable course and high mortality rate.
IPF is a devastating lung condition that significantly impacts patients’ quality of life and carries a poor prognosis if left untreated. While there are treatment options available that can slow progression of the disease, these are not suitable for all patients and often have many negative side effects. There is therefore a need to develop new treatment options with improved tolerability.
The application of deep learning AI technology is emerging as a potential game-changer in IPF clinical trials, offering new hope for patients and researchers alike. This article explores the complexities of IPF, examines how deep learning imaging is enhancing research in this area, and discusses the potential for accelerated drug development and improved patient outcomes.
Lung disease is a complex medical problem
IPF is characterised by the gradual scarring of lung tissue (fibrosis), which leads to a decline in lung function and the onset of severe respiratory symptoms. As the disease progresses, patients typically face increasing difficulties with breathing, which severely impacts their quality of life.
The unpredictable nature of IPF progression adds another layer of complexity to its disease management.
Current diagnosis relies heavily on visually assessing fibrotic features in high-resolution computed tomography (HRCT) lung scans.1 The unpredictable nature of IPF progression adds another layer of complexity to its disease management. While some individuals may experience a swift decline, others might see a more gradual deterioration over an extended period, making prognosis and treatment planning particularly challenging.
Deep learning turns complex data into simple classifications
When applied to CT imaging for IPF, deep learning can analyse patterns and accurately map them to clinical outcomes. This approach is transformative for IPF, enabling detection of subtle image changes that humans might miss or take much longer to analyse. So, how does deep learning contribute to developing treatments for lung disease?
To judge how a treatment is working in a patient during a clinical trial, clinicians need to understand the progression of the disease and how a treatment is altering disease progression. Since IPF progression is measured through a combination of changes in fibrosis, lung volume, lobar flow and blood biomarkers,2 AI technology can effectively pick up these data points and feed the information back to clinicians.
To judge how a treatment is working in a patient during a clinical trial, clinicians need to understand the progression of the disease and how a treatment is altering disease progression.
Since deep learning detects lung image changes more precisely than humans, it enables more sensitive measurement of fibrosis progression and quantifies changes across multiple lung compartments in clinical trials.
Additionally, deep learning can effectively evaluate treatment response by linking multiple datasets together, an incredibly useful feature of AI that is particularly useful for complex medical problems. Curating data in this way gives a thorough assessment of treatment efficacy, leading to better-informed decisions about drug or treatment development within a clinical trial.
These aspects combined lead to hugely improved efficiency in clinical trial workflows, significantly reducing the time required for image interpretation and potentially accelerating the patient enrolment, clinical trial and data analysis timelines. Since the heavy lifting is being done by AI, there is increased reliability and consistency of image analysis – ie, AI gives scientists a deeper, more nuanced view of the disease as a whole, which enhances the reliability and reproducibility of clinical trial data for future research.
A more connected view of disease will lead to more effective medical treatments
Deep learning imaging holds significant promise for advancing our understanding and treatment of progressive pulmonary fibrosis (PPF), as demonstrated by recent studies showcasing its prognostic value in fibrotic lung disease.3-5 Its potential also extends to drug development, where it provides powerful tools to streamline and accelerate the traditionally long and expensive process of bringing new therapies to market.
By providing robust clinical trial outcome measures and enabling early detection of treatment efficacy, the integration of deep learning techniques has the potential to significantly improve drug development.
This will be driven by:
- Faster, data-driven drug development – AI enables researchers to quickly assess treatment effectiveness, accelerating decision-making in clinical trials
- More efficient clinical trials – AI-driven data analysis of disease progression and treatment response can potentially shorten trial duration and reduce participant numbers, leading to faster drug approvals
- Improved patient stratification – AI helps identify the right patients for trials, improving success rates and expediting patient recruitment
- Stronger safety and efficacy data – AI provides large-scale, accurate assessments of overall drug efficacy and safety, supporting continuous research and development on a larger scale.
Implementing DL in clinical trials presents challenges, including the need for large CT datasets for algorithm training, maintaining ethical standards and patient confidentiality, and conducting clinical utility studies to validate real-world benefits. While challenges remain, the impact on drug development is clear, especially in lung disease. DL is already being deployed in major IPF6 and PFF clinical trials (eg, MIST7 and ASPIRE)8 with increasing collaborations between deep learning labs, universities and hospitals worldwide.
Overcoming the barriers: integrating new tech into drug development
With growing attention on AI in various industries, now is a critical time to harness its potential in medicine. Despite the huge challenges ahead, it is clear that we have the technology and the expertise to change the status quo in clinical trials and drug development. This not only improves patient outcomes but also strengthens the UK’s position as a leader in science and technology.
As we continue to explore and refine deep learning for lung disease and other medical applications, we must balance AI safeguarding with innovation, especially in clinical trials where sensitive medical data and patient health are at stake. Effective collaboration between healthcare divisions, the NHS, hospitals, universities and tech companies will be essential. Additionally, fostering realistic expectations about the capabilities of DL technology and building trust in new technology will be key to its successful integration.
Meet the author
Muhunthan Thillai – CEO, Qureight
Muhunthan is the CEO at Qureight. A board-certified pulmonologist, he has over 20 years of experience in thoracic medicine . He qualified as a doctor from Imperial College London and undertook specialist medical training in Oxford, where he was appointed as a member of the Royal College of Physicians. He was awarded a PhD in molecular immunology from Imperial College London before being appointed as a consultant physician. He was previously Director of Interstitial Lung Diseases at Royal Papworth and Addenbrooke’s Hospitals Cambridge, UK and is currently an Honorary Associate Professor at the University of East Anglia.
References
[1] Sul B, Flors L, Cassani J, et al. Volumetric characteristics of idiopathic pulmonary fibrosis lungs: computational analyses of high-resolution computed tomography images of lung lobes. Respiratory Research. 2019 Oct 11;20(1).
[2] Maher TM. Biomarkers for Interstitial Lung Abnormalities: A Stepping-stone Toward Idiopathic Pulmonary Fibrosis Prevention? American Journal of Respiratory and Critical Care Medicine [Internet]. 2022 Aug 1 [cited 2025 Mar 25];206(3):244–6. Available from: https://pmc.ncbi.nlm.nih.gov/articles/PMC9890254/
[3] Walsh SLF, Humphries SM, Wells AU, Brown KK. Imaging research in fibrotic lung disease; applying deep learning to unsolved problems. The Lancet Respiratory Medicine [Internet]. 2020 Nov 1 [cited 2022 Mar 8];8(11):1144–53. Available from: https://www.sciencedirect.com/science/article/abs/pii/S2213260020300035
[4] Walsh SLF, Mackintosh JA, Calandriello L, Silva M, et al. Deep Learning–based Outcome Prediction in Progressive Fibrotic Lung Disease Using High-Resolution Computed Tomography. American Journal of Respiratory and Critical Care Medicine. 2022 Oct 1;206(7):883–91.
[5] Sean, Felder FN, Walsh SLF. From pixels to prognosis: unlocking the potential of deep learning in fibrotic lung disease imaging analysis. British Journal of Radiology. 2024 May 23;97(1161):1517–25.
[6] Pinching J. Qureight and Avalyn launch strategic partnership in pulmonary fibrosis – PharmaTimes [Internet]. PharmaTimes. 2025 [cited 2025 Mar 25]. Available from: https://pharmatimes.com/news/qureight-and-avalyn-launch-strategic-partnership-in-pulmonary-fibrosis/
[7] Home – Mist – A PPF Clinical Study [Internet]. Mist – A PPF Clinical Study. 2024 [cited 2025 Mar 25]. Available from: https://mistppfstudy.com/
[8] ASPIRE IPF Ph2b trial – Vicore Pharma [Internet]. Vicore Pharma. 2024 [cited 2025 Mar 25]. Available from: https://vicorepharma.com/our-programs/pipeline/aspire-ph2b-trial/
Related topics
Artificial Intelligence, Biomarkers, Clinical Trials, Drug Discovery, Drug Discovery Processes, Imaging
Related conditions
Idiopathic pulmonary fibrosis (IPF)
Related organisations
Qureight
Related people
Muhunthan Thillai (CEO - Qureight)