Machine-learning algorithm developed to aid in epigenetic drug discovery
Posted: 23 October 2019 | Rachael Harper (Drug Target Review) | No comments yet
A machine-learning algorithm has been created that automates high-throughput screens of epigenetic medicines.
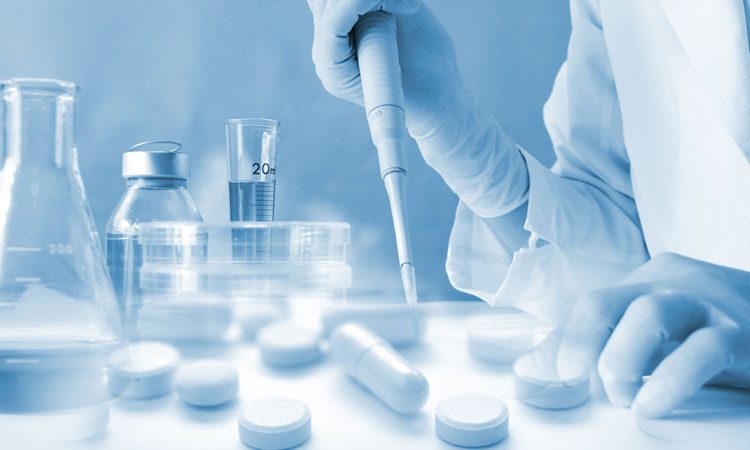
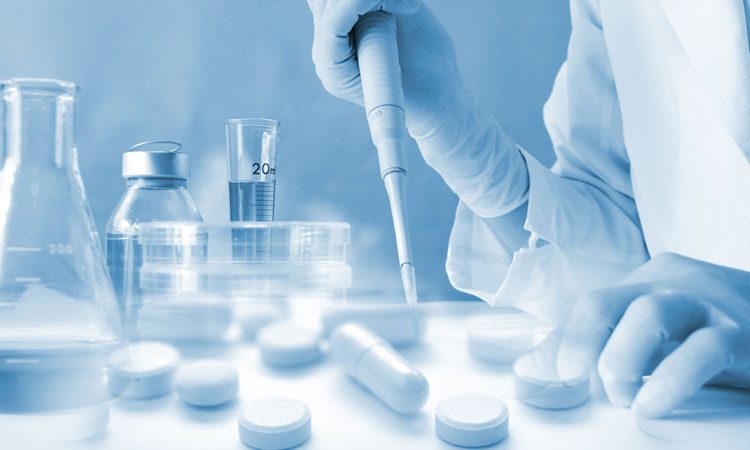
A machine-learning algorithm has been developed by scientists at Sanford Burnham Prebys Medical Discovery Institute, US that gleans information from microscope images. This allows for high-throughput epigenetic drug screens that could unlock new treatments for cancer, heart disease, mental illness and more.
“In order to identify the rare few drug candidates that induce desired epigenetic effects, scientists need methods to screen hundreds of thousands of potential compounds,” says Alexey Terskikh, PhD, associate professor in Sanford Burnham Prebys Medical Discovery Institute and senior author of the study. “Our study describes a powerful image-based approach that enables high-throughput epigenetic drug discovery.”
The scientists used the approach to identify epigenetic compounds that may be able to help treat glioblastoma”
Epigenetics refers to chemical tags on DNA that allow cellular machinery greater or less access to genes – thus altering gene expression. Several medicines that target epigenetic alterations are approved by the US Food and Drug Administration (FDA) for the treatment of cancer and researchers are working to find additional epigenetic-based therapies.
In the study, the scientists trained a machine-learning algorithm using a set of more than 220 drugs known to work epigenetically. The resulting method, called Microscopic Imaging of Epigenetic Landscapes (MIEL), was able to detect active drugs, classify the compounds by their molecular function, spot epigenetic changes across multiple cell lines and drug concentrations and help identify how unknown compounds work.
The scientists used the approach to identify epigenetic compounds that may be able to help treat glioblastoma, a deadly brain cancer.
Terskikh and his team are already using the algorithm to study epigenetic changes in ageing cells, with the aim of developing compounds that promote healthy ageing – the greatest risk factor for disease. Terskikh is also eager to broaden the technology from two dimensional (2D) images to three dimensional (3D) videos, which will expand the power of the approach.
The study was published in eLife.
Related topics
Drug Discovery, Drug Discovery Processes, Epigenetics, High-Throughput Screening (HTS), Imaging, Informatics
Related conditions
Cancer, Glioblastoma
Related organisations
Sanford Burnham Prebys Medical Discovery Institute
Related people
Alexey Terskikh PhD