Machine learning method to design better antibody drugs developed
Posted: 16 April 2021 | Victoria Rees (Drug Target Review) | No comments yet
Researchers have developed an artificial intelligence to identify the best potential antibody drug from groups of up to a few thousand.
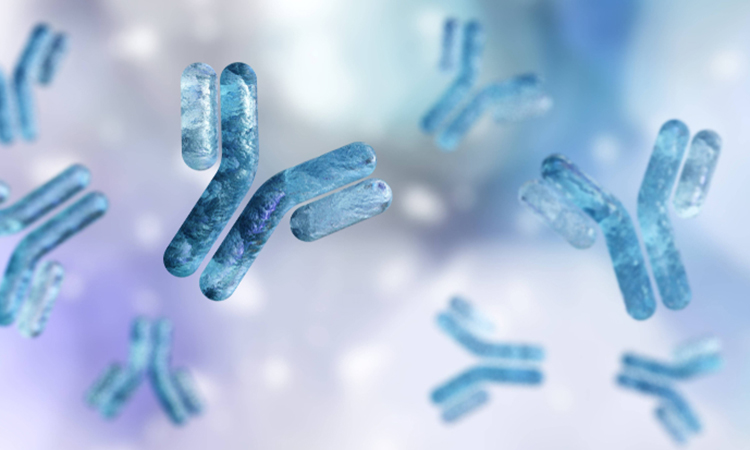
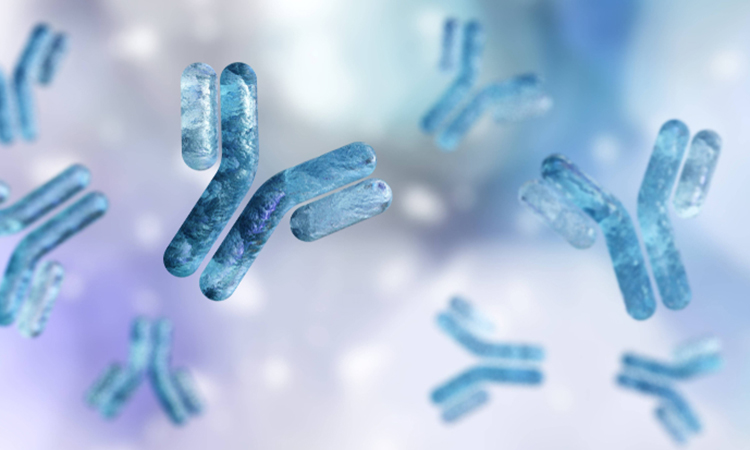
Scientists at ETH Zurich, Switzerland, have created a machine learning method that supports the optimisation phase of antibody drugs, potentially helping to develop more effective therapeutics.
According to the team, when researchers optimise an entire antibody molecule in its therapeutic form (ie, not just a fragment of an antibody), it used to start with an antibody lead candidate that binds reasonably well to the desired target structure. Then researchers randomly mutate the gene that carries the blueprint for the antibody in order to produce a few thousand related antibody candidates in the lab. The next step is to search among them to find the ones that bind best to the target structure.
“With automated processes, you can test a few thousand therapeutic candidates in a lab. But it is not really feasible to screen any more than that,” said lead researcher Professor Sai Reddy. Typically, the best dozen antibodies from this screening move on to the next step and are tested for how well they meet additional criteria. “Ultimately, this approach lets you identify the best antibody from a group of a few thousand.”
The researchers are now using machine learning to increase the initial set of antibodies to be tested to several million. They provided the proof-of-concept for their new method using Roche’s antibody cancer drug Herceptin, which has been on the market for 20 years.
“We were not looking to make suggestions for how to improve it – you cannot just retroactively change an approved drug,” Reddy explained. “Our reason for choosing this antibody is because it is well known in the scientific community and because its structure is published in open-access databases.”
Starting out from the DNA sequence of the Herceptin antibody, the researchers created about 40,000 related antibodies using a CRISPR mutation method they developed a few years ago. Experiments showed that 10,000 of them bound well to the target protein in question, a specific cell surface protein. The scientists used the DNA sequences of these 40,000 antibodies to train a machine learning algorithm.
They then applied the trained algorithm to search a database of 70 million potential antibody DNA sequences. For these 70 million candidates, the algorithm predicted how well the corresponding antibodies would bind to the target protein, resulting in a list of millions of sequences expected to bind.
Using further computer models, the scientists predicted how well these millions of sequences would meet the additional criteria for drug development (tolerance, production, physical properties). This reduced the number of candidate sequences to 8,000.
From the list of optimised candidate sequences on their computer, the scientists selected 55 sequences from which to produce antibodies in the lab and characterise their properties. Subsequent experiments showed that several of them bound even better to the target protein than Herceptin itself, as well as being easier to produce and more stable than Herceptin.
“One new variant may even be better tolerated in the body than Herceptin,” said Reddy. “It is known that Herceptin triggers a weak immune response, but this is typically not a problem in this case.”
The scientists are now applying their artificial intelligence method to optimise antibody drugs that are in clinical development. To this end, they recently founded deepCDR Biologics, which partners with both early stage and established biotech and pharmaceutical companies for antibody drug development.
The study was published in Nature Biomedical Engineering.
Related topics
Antibodies, Artificial Intelligence, Biopharmaceuticals, Drug Discovery, Informatics, Proteomics
Related organisations
ETH Zurich, Roche
Related people
Professor Sai Reddy