Artificial intelligence-aided screening could boost speed of new drug discovery
Posted: 15 December 2022 | Aida Tayebi (University of Central Florida), Dr Ozlem Ozmen Garibay (University of Central Florida) | No comments yet
Using a natural language-inspired technique, researchers at the University of Central Florida, US, developed an interpretable and generalisable drug target interaction model that achieves 97 percent accuracy in identifying drug candidates for a broad variety of target proteins. Here, Dr Ozlem Ozmen Garibay and Aida Tayebi, who worked on the study, outline their work and how their findings could shape drug discovery.
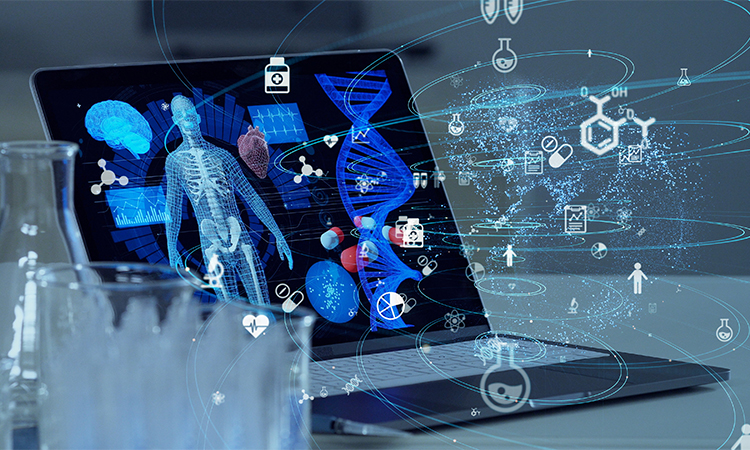
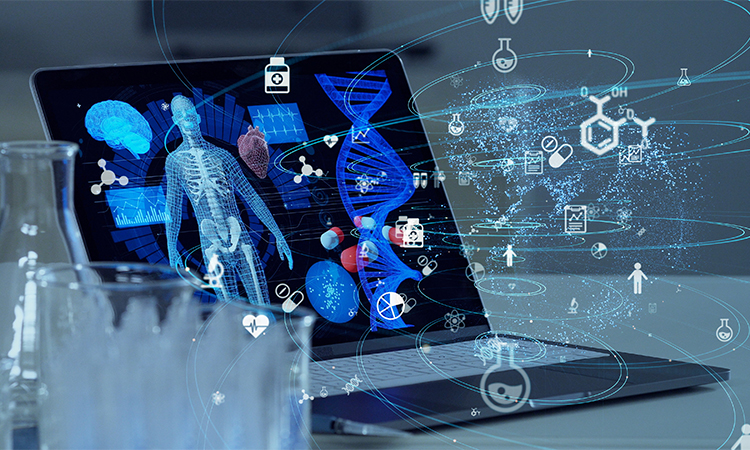
Drug target interaction (DTI) prediction tasks performed in vitro can be expensive and time consuming. In silico approaches have been used to reduce both cost and time to discover drugs virtually by screening previously known drugs for new treatments and new purposes. This is also known as drug repurposing. Virtual screening reduces the vast molecular interaction landscape to focus the further discovery on potentially promising candidate drugs. Additionally, it can also accelerate the drug discovery process for a new target and disease by repurposing previously known drugs that have already passed clinical trial studies for their effectiveness, safety and side effects and are therefore approved by the US Food and Drug Administration (FDA). Computational screening narrows the list of candidate drugs for further in vitro and in-lab experiments.
A new artificial intelligence (AI)-based DTI model developed by researchers at the University of Central Florida, has sped up the drug screening process against the COVID-19 virus. This research, published in Briefing in Bioinformatics,1 was conducted through an interdisciplinary collaboration between computer scientists and material scientists. This model, known as AttentionSiteDTI, is inspired by models developed for sentence classification in the field of natural language processing (NLP). It is also the first model that uses the pair of drug and target as a biochemical sentence, with relational meaning between protein pockets and drug molecules which is the key to capture the most valuable contextual semantic or relational information of the sentence. Furthermore, the AttentionSiteDTI model enables an end-to-end graph convolutional neural network model that learns embeddings from the graphs of small molecules and proteins which are not fixed and are sensitive to context similar in NLP.
The researchers outperformed other state‑of‑the‑art studies in predicting the interaction between drug and target and have identified candidates by using deep learning with a self‑attention mechanism to extract the features that rule the most in the complex interaction. They have proved high interpretability through the self-attention mechanism by focusing on the most important parts of the protein interacting with the drug compounds (binding sites); for example, those that contribute the most towards the interaction and high generalisability through the protein input representation that uses protein pockets in the form of graphs.
This is a critical step in the design and development of new drugs to know which biological properties of the compound governs the interaction. According to the study, a benefit of utilising graph convolutional networks is their robustness to different orientations of the three‑dimensional (3D) structures of proteins, however a drawback to this is to find high-quality 3D protein structure.
In this study, the 3D protein structures were extracted from the protein data bank (PDB) which provides all the experimental methods such as nuclear magnetic resonance (NMR), X-ray diffraction and cryogenic-electron microscopy (cryoEM). The binding sites were extracted through a docking-based model which was previously studied. This method provides bounding box co‑ordination for each binding site of a protein. Next, they are used to convert the protein structure to a set of peptide fragments. Then the graph of protein is constructed by each atom acting as a node and the connections between atoms acting as edges. The feature vector of each atom, one‑hot encoding of atom type, atom degree, total number of hydrogen atoms and implicit valence of the atom are also reported in the form of a vector. The Simplified Molecular-Input Line-Entry system (SMILE) of the drug compounds were also represented in the form of graphs in a way that each atom in the small molecule is represented as a node of the graph and the connections between them are represented as edges. In addition, the graph’s atom features using one-hot encoding of atom type, atom degree, formal charge of the atom, number of radical electrons of the atom, the atom’s hybridisation, atom’s aromaticity and number of total hydrogens of the atom are also reported in the form of a vector.
One-dimensional representation is insufficient for complex interactions, particularly for proteins, which are much larger and more complex molecules than drugs. The improved performance of this model is due to the use of graph representations, which are an advanced feature representation and can significantly affect the model’s performance in capturing the structural information of molecules. According to this study, traditional machine learning and deep learning methods that use string representations cannot learn complex non‑linear relationships in drug target interaction. The self‑attention mechanism aids the AttentionSiteDTI model to extract the features automatically and to learn higher order non-linear relationships. The team used three benchmark datasets, DUD-E, Human and BindingDB, to compare the new model with state‑of-the-art graph-based models. AttentionSiteDTI performs comparably well against the state-of-the-art DTI prediction models when using a target protein that the prediction models are trained on. However, when the target protein is changed to another that the models have not been trained on, the performance of AttentionSiteDTI remains robust while the performance of the other models decreases significantly, which indicates a greater degree of generalisability achieved by the new model. This is important because it highlights the AttentionSiteDTI model can be used for a broad variety of protein targets with high performance.
This study is significant since it will assist other researchers to accelerate the drug design by identifying the binding sites’ functional properties. Drug designers can use AI and quickly act in response to new diseases and pandemics such as COVID-19, focusing on the most important binding sites of the virus’s protein. They are able to screen many variations of the protein and small molecules using AI to get accurate predictions of the binding before doing any laboratory experiments.
Furthermore, the team evaluated the binding between spike protein (along with ACE2 protein) of the SARS-CoV-2 virus and the seven candidate compounds (N-acetyl-neuraminic acid, 3α,6α‑Mannopentaose, N-glycolylneuraminic acid, 2-Keto3-deoxyoctonate, N-acetyllactosamine, cytidine5- monophospho-N-acetylneuraminic acid sodium salt and Darunavir) using a binding inhibition assay kit. The strength of the interaction was measured through laboratory experiments in the form of IC50 (half maximal inhibitory concentration) between the pair of drug and target. In this study, candidate molecules were used as inhibitors of the spike protein-ACE2 complex formation. The activity threshold was set at 15nM to identify the best compounds. This evaluation and comparison proved high agreement between computational prediction and experiment results. This shows the potential of the AttentionSiteDTI model in providing the drug designers with an effective tool to pre-screen small molecules in drug repurposing applications for the current pandemic, as drugs to treat COVID are still of interest and to be prepared for future possible pandemics.
About the authors
Reference
- Yazdani-Jahromi M, Yousefi N, Tayebi A, et al. AttentionSiteDTI: An interpretable graph-based model for drug-target interaction prediction using NLP sentence-level relation classification. Briefings in Bioinformatics. 2022;23(4).
Related topics
Artificial Intelligence, Drug Discovery, Drug Discovery Processes, Drug Targets, Informatics, Lab Automation, Technology
Related conditions
Covid-19
Related organisations
US Food and Drug Administration (FDA)