Latest in lab automation
Posted: 9 February 2023 | Izzy Wood (Drug Target Review) | No comments yet
In this article Drug Target Review’s Izzy Wood highlights three of the latest findings using lab automation techniques and technologies that aid scientists.
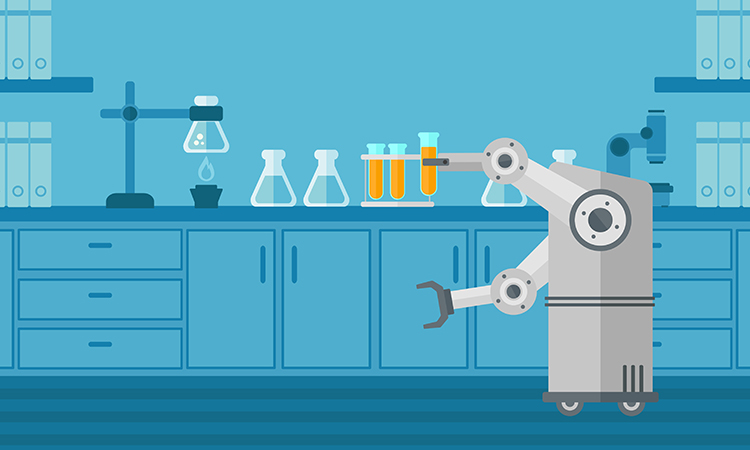
Lab automation is the integration of automated technologies into the laboratory to enable new and improved processes1.
Typically, lab automation offers solutions that eliminate repetitive tasks in a lab, such as liquid handling, that have usually been performed by scientists1.
As the technology advances, more complex processes, even whole workflows, can now be automated. Increasingly, automated technology is a requirement in today’s laboratories to remain competitive and meet demand.
In this article, Drug Target Review’s Izzy Wood highlights the latest discoveries using lab automation.
Database could predict cancer
Cancer prediction medicine gained a boost with the recent unveiling of a new cancer protein profile database compiled from artificial intelligence (AI) and machine learning. The new open-access Disease Blood Atlas provides a first-ever map of the proteome signature in blood from cancer patients.
The new database, produced by the Human Protein Atlas consortium,2 based within SciLifeLab, a joint research centre that includes KTH Royal Institute of Technology, Uppsala University, Karolinska Institutet and Stockholm University, all Sweden, highlights 1,463 proteins associated with 12 different types of cancer, and presents proteins that can be used to identify individual cancer types based on a drop of blood.
The Disease Blood Atlas was compiled from measurements of minute amounts of blood plasma collected from 1,400 cancer patients, at the time of diagnosis and before treatment. The blood samples underwent a combination of statistical analysis of gene expression and machine-learning-based disease prediction.
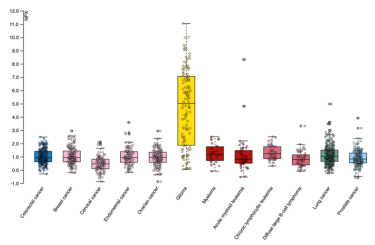
The yellow bar in this readout of protein GFAP indicates elevated expression in the blood of patients with brain tumours [Credit: Human Disease Blood Atlas]
The section called “Protein 3-D Structure” shows the three-dimensional structures for all human proteins using an AI-based prediction model (AlfaFold). In addition, a major update of the Tissue Atlas section provides detailed multiplex spatial profiling of proteins specific for human testis and kidney. More data is also provided on single cell analysis of tissues and organs, as well as data from an extensive catalogue of human cell lines.
”We believe that the new sections of the open access Human Protein Atlas with large amounts of novel data covering all human proteins provides new dimensions of valuable information for researchers interested in human biology and disease,” Uhlén concluded .
You can read the full piece here.
Mathematical model predicts long-term effectiveness of COVID-19 vaccine
Researchers from Massachusetts General Hospital (MGH), US, recently designed a mathematical model that can predict the course of vaccine-induced immunity against COVID-19 in different patient populations, including otherwise healthy individuals and those who have cancer or suppressed immune responses.
The model incorporates different variants of SARS-CoV-2.
The model also makes predictions under potential future scenarios (such as the emergence of SARS-CoV-2 variants with greater immune evasion) and reveals the benefits of the new bivalent vaccines.
The model, published in PNAS, builds on the investigators’ previously developed mathematical framework that they used to understand why treatment responses vary widely among people with COVID-19 and to identify biological markers related to these different responses.
In this latest work, also published in PNAS,2 the scientists addressed the need for predictions of vaccine effectiveness over time.
“We used this model to simulate how differences in viral, patient, and vaccine characteristics may affect COVID-19 outcomes,” said senior author Dr Rakesh K. Jain, director of the E.L. Steele Laboratories for Tumour Biology at MGH.
The model incorporates different variants of SARS-CoV-2 (including hypothetical ones), original and bivalent forms of the vaccine, and different considerations for certain patients, such as interactions between the virus, immune cells, and tumour cells in individuals with cancer.
The model predicted that a booster dose of either Pfizer-BioNTech or Moderna mRNA vaccines can induce robustly enhanced antibody and immune cell–based responses against SARS-CoV-2, to provide sufficient protection for more than one year in healthy individuals.
“Our results could help inform the timing of booster vaccinations in individuals with different characteristics and comorbidities, as well as for novel viral variants,” added Jain.
Read more about the study here.
Computer model determines drug candidate’s ability to bind to proteins
Combing computational physics with experimental data, researchers from the University of Arkansas, US, have developed computer models for determining a drug candidate’s ability to target and bind to proteins within cells. The work was recently published in Nature Computational Science.3
If accurate, such an estimator could computationally demonstrate binding affinity and thus prevent experimental researchers from needing to investigate millions of chemical compounds. The work could substantially reduce the cost and time associated with developing new drugs.
“We developed a theoretical framework for estimating ligand-protein binding,” said Associate Professor Mahmoud Moradi. “The proposed method assigns an effective energy to the ligand at every grid point in a coordinate system, which has its origin at the most likely location of the ligand when it is in its bound state.”
Moradi and Thallapuranam used biased simulations — as well as non-parametric re-weighting techniques to account for the bias — to create a binding estimator that was computationally efficient and accurate. They then used a mathematically robust technique called orientation quaternion formalism to further describe the ligand’s conformational changes as it bound to targeted proteins.
The researchers tested this approach by estimating the binding affinity between human fibroblast growth factor 1 — a specific signalling protein — and heparin hexasaccharide 5, a popular medication.
The project was conceived because Moradi and Thallapuranam were studying human fibroblast growth factor 1 protein and its mutants in the absence and presence of heparin. They found strong qualitative agreement between simulations and experimental results.
“When it came to binding affinity, we knew that the typical methods we had at our disposal would not work for such a difficult problem,” Moradi said. “This is why we decided to develop a new method. We had a joyous moment when the experimental and computational data were compared with each other, and the two numbers matched almost perfectly.”
Find out more here.
References:
- What is lab automation? [Internet]. Automata. 2023 [cited 2023Feb9]. Available from: https://automata.tech/what-is-lab-automation/
- The human protein atlas [Internet]. The Human Protein Atlas. [cited 2023Feb9]. Available from: https://www.proteinatlas.org/
- Voutouri C, Hardin CC, Naranbhai V, Nikmaneshi MR, Khandekar MJ, Gainor JF, et al. Mechanistic model for booster doses effectiveness in healthy, cancer, and immunosuppressed patients infected with SARS-COV-2. Proceedings of the National Academy of Sciences. 2023;120(3).
- Govind Kumar V, Polasa A, Agrawal S, Kumar TK, Moradi M. Binding affinity estimation from restrained umbrella sampling simulations. Nature Computational Science. 2022;3(1):59–70.
Related topics
Analysis, Analytical Techniques, Artificial Intelligence, Big Data, Bioinformatics, Covid-19, Drug Development, Drug Discovery Processes, Informatics, Lab Automation, Protein, Protein Expression, Targets
Related conditions
Cancer, Covid-19, SARS-CoV-2