Biochemical assays and deep cyclic inhibition in cancer treatment
Posted: 12 July 2023 | Taylor Mixides (Drug Target Review) | No comments yet
Drug Target Review’s Taylor Mixides exclusively interviews Brett Hall, Chief Scientific Officer at Immuneering, about his company’s aim to develop a universal-RAS therapy.
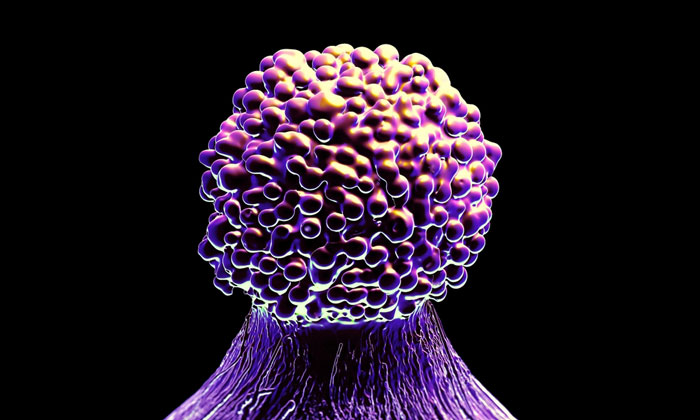
The clinical-stage oncology company develops medicines for broad populations of cancer patients and aims to achieve universal-RAS activity through deep cyclic inhibition of the mitogen-activated protein kinase (MAPK) pathway, selectively impacting cancer cells while sparing healthy cells.
In the drug discovery process, Immuneering uses patient-aligned 3D tumour growth assays (3D-TGAs), pharmacogenomics and bioinformatics data, deep learning AI technology, and available clinical data to identify potential tumour pathway addiction profiles and determine which types of RAS-mutant cancers may be well suited to the deep cyclic inhibition mechanism. Molecular-level biochemical assays like transcriptomics, genomics and proteomics have emerged as valuable tools for identifying potential targets in cancer treatment through deep cyclic inhibition (DCI). These assays provide insights into the molecular mechanisms of disease biology and drug response, enabling the characterisation of gene expression profiles and deviations in diseased cells. By quantifying changes in gene expression following exposure to diverse compounds, DCI establishes a blueprint for optimal engagement of specific disease states.
This systematic approach addresses challenges associated with traditional drug discovery methods and offers the potential to identify promising drug candidates
How do molecular-level biochemical assays like transcriptomics, genomics and proteomics help in identifying potential targets for DCI in cancer treatment?
Omics data, including pharmacogenomics data from 3D-TGAs, provide us with unique information about the molecular mechanisms of disease biology and drug response. Importantly, transcriptomics information shows that certain aspects of a disease change the gene expression profile. For example, tumour gene expression profiles can be interrogated for deviations from normal healthy cells. Our disease cancelling technology (DCT) platform evaluates reversal of tumour-specific gene expression profiles following exposure to certain targeted agents. This platform can help identify and optimise pharmacologic properties of new drugs.
We also leverage existing clinical gene expression data from different subsets of molecularly profiled cancer patients, who differ in a certain aspect of disease or response to a particular therapy. We associate transcriptomic profiles with each subset, which can be directly evaluated across several proprietary translational platforms to identify patient subsets most likely to benefit from a given therapy.
Like the mechanism of noise cancelling headphones but at the molecular level of a cell, DCT examines a particular aspect of disease, then characterises it at the gene expression level. Changes in gene expression profiles are quantified following exposure to thousands of different compounds, and the extent and timing to which disease signals are reversed can establish a blueprint for how to optimally engage specific disease states. This approach enables identification of potential drug candidates consistently and systematically. It also addresses challenges seen with traditional chemical or biological approaches in drug discovery, which often try to identify targets in cell lines that do not reliably exemplify a disease in relevant patient populations.
Many cell lines used in pancreatic cancer research, for example, are generally not genetically aligned with most patients in the clinic.
If there is robust gene expression data for the aspect of a disease that we are aiming to reduce or eliminate, the DCT mechanism will find results that can lead us to promising new drug candidates.
Once a new drug target is identified, a proprietary deep learning artificial intelligence (AI) platform we call Fluency can rapidly identify small molecules that selectively bind to a target of interest. This AI technology identifies attractive drug candidates within any virtual or real chemical libraries by making ranked predictions of binding affinity for all compounds within any library containing millions of compounds. Fluency is especially useful to help target new proteins or known proteins in new ways.
How does the DCI mechanism compare to the design of other drugs for cancer treatment?
Deep cyclic inhibition (DCI) is designed to administer medication at least once a day with the goal of disrupting key target pathways. Hitting tumours deeply with high peak drug exposures helps to break addiction to the underlying oncogenic pathways that drive many types of cancer. When endowed with a short drug half-life, high peak-drug exposures swiftly cycle to near-zero drug troughs in a cycle that is repeated at least once daily. As a result, in the DCI approach, patients experience a daily ‘drug holiday’. This is a counterintuitive approach compared to traditional treatments that are designed to administer drug that sustains target engagement 24/7 to shut down the MAPK pathway. DCI is designed to maximally impact tumour cells while sparing healthy cells, which has been a primary challenge with many first-generation cancer treatments.
The goal of DCI in preclinical and clinical assessments is to achieve a manyfold higher drug free-fraction pharmacokinetic (PK) Cmax level (the plasma concentration of therapy in a specific area of the body) to break tumour addiction to the MAPK signalling pathway followed by a rapid drop off of drug levels enabled by a short plasma half-life. With this drop off, the goal is to reach a near-zero drug trough before the 24-hour mark. This gives healthy cells an opportunity to restore homeostatic MAPK pathway signalling.
With this approach our hope is to treat cancer effectively while limiting drug resistance and improving drug tolerability. We are pleased that to date our lead product candidate has aligned DCI profiles as expected. We have so far observed extraordinary PK Cmax levels and a mean half-life of approximately two hours in our Phase I trial to date. In April 2023, we presented preliminary safety data at the AACR Annual Meeting where our lead product candidate was observed to be well tolerated with no dose limiting toxicities observed in cancer patients through the first four dose levels.
DCI is also designed to broadly target mutations in RAS genes (which are part of the MAPK pathway) that are known to drive many intractable solid tumours including pancreatic, melanoma, lung and colorectal, including KRAS, HRAS and NRAS. Currently, RAS-selective inhibitors focus on one specific RAS mutation, whereas the DCI approach targets MEK (a key protein kinase in the MAPK signalling pathway) downstream of RAS and thus could have broad potential applications in some of the most challenging tumour types.
How does DCI specifically target cancer cells while minimising damage to healthy cells?
Widely used cancer drugs are administered with the goal of 24/7 disruption of the MAPK pathway. This approach has thus far been restricted to RAF mutant tumours, most often in combination with RAF-specific inhibitors, and these drugs are associated with clinically limiting toxicities given the treatment also affects healthy cells that rely on sporadic MAPK pathway signalling.
Based on data analyses using our Disease Cancelling Technology, we found that both tumour cells and healthy cells use the MAPK pathway but in different ways. Tumour cells need continuous MAPK pathway signalling to grow and divide, whereas healthy cells can tolerate more moderate or sporadic levels of MAPK signalling. In other words, tumour cells need the MAPK pathway like we need air (ie, a constant supply) and healthy cells need the pathway in the same way we need water (ie, on a more intermittent basis). Thus, healthy cells can likely go several hours without ‘water’, or MAPK signalling. By deeply cycling the MAPK pathway on and off instead of chronic disruption, the goal of DCI is to focus therapeutic intervention more against tumour cells than healthy cells.
We have assessed the DCI approach extensively and seen dramatic tumour growth inhibition activity in animal models driven by several different types of RAS mutations, including but not limited to KRAS G12C mutations.
If researchers continue to use an intuitive approach of chronically suppressing the MAPK pathway, we will likely continue to see the same results in patients with little improvement. Our approach is to treat cancer in fundamentally new ways based on emergent data to optimise therapeutic outcomes. DCI is based on this key shift in mindset – we do not need to turn off the MAPK pathway 24/7, we only need to prevent it from being on 24/7.
What are some of the challenges associated with developing a DCI platform for cancer treatment using molecular-level biochemical assays?
We use humanised 3D tumour growth models or assays to predict drug response and give more weight to models that better align with the genetic signatures of patients likely to be encountered in the clinic. However, there are many different types of cancer and it can be difficult to determine the level of MAPK pathway addiction, which is ideal for response to monotherapy. We evaluated more than 100 different human growth assays across a dozen tumour types to determine sensitivity and resistance profiles to this therapeutic approach. Using our bioinformatics expertise, we then map those profiles onto a large database of patient data – the AACR Project GENIE database – to translationally predict which tumour types are likely to be most responsive to monotherapy or combination therapy to our drug candidate.
In just a few examples, following our analysis we found that in pancreatic cancer and melanoma most patients with RAS mutations display genomic profiles that may be sensitive to monotherapy. There are also opportunities with other RAS-mutant tumours including non-small cell lung cancer and colorectal cancer. These findings led us to start with four cancer types as our initial target indications. Based on our analysis, we also found that patients with prostate and breast cancer, or glioblastoma, would be in the resistant category, meaning very few patients with these cancers would likely benefit from MAPK signalling disruption. This is because these cancer types infrequently depend on the MAPK pathway, thus DCI of MEK would have limited effect. Our assays and bioinformatics modelling can help identify certain indications that are appropriate for DCI while ruling others out.
Can you describe how DCI is designed to address the issue of drug resistance in cancer treatment?
Given that traditional treatments have a long plasma half-life or have a moderately long half-life and are administered at a higher dose frequency, they are designed to chronically disrupt the MAPK pathway 24/7. This approach has historically shown issues with drug resistance and poor tolerability. DCI could help overcome these challenges. With DCI, drug is administered at least once per day and drives stop-and-start cycles in the target pathway. DCI is designed to enable patients to take daily ‘drug holidays’ given the number of drug half-lives between each dose. This new approach is designed to more effectively impact cancer cells by limiting adaptive resistance, a process where cells upregulate compensatory pathways in response to drug-related activities.
How do pre-clinical studies help to determine the safety and efficacy of DCI, and what types of pre-clinical research are typically conducted in this field?
Preclinical studies including assessments based on animal models, humanised 3D-TGAs, pharmacogenomics modelling, and in vitro and in vivo models all play important roles in determining the potential safety and efficacy of DCI and optimal targets for treating different cancers. In preclinical research, we leveraged more than 100 tumour models, including 75 different models displaying various RAS mutations. We assessed whether DCI was an optimal therapeutic approach for a range of cancers including pancreatic, lung, colorectal, melanoma, thyroid, sarcoma, breast, ovary, liver, neuroblastoma and others. These tumour models were essential to determine which types of cancer patients may be addicted to the MAPK pathway and responsive to the DCI mechanism of action.
Some of our animal models were also used to conduct direct comparisons with our lead product candidate based on DCI compared to standard of care therapies for several types of cancer. For example, we conducted a head-to-head comparison of our product candidate with and without sotorasib in a KRAS G12C pancreatic tumour mouse model, versus sotorasib and adagrasib in a KRAS G12V pancreatic tumour mouse model, versus binimetinib in a NRAS Q61R melanoma xenograft tumour mouse model, and versus selumetinib and binimetinib in a KRAS G12D colorectal cancer syngeneic tumour mouse model. Results from each of these head-to-head studies showed that our product candidate resulted in comparable to greater tumour growth inhibition and was well tolerated in each study.
In your opinion, what are the most promising applications for DCI beyond cancer treatment, and how might molecular-level biochemical assays help in these areas?
DCI is designed to broadly target mutations in RAS genes that are known to drive many intractable solid tumours including pancreatic, melanoma, lung and colorectal. DCI is not limited to cancer or MEK itself. The core thesis for DCI could, in theory, be applied to any target or pathway that is either inappropriately activated in the disease state, or where there is a ‘biologic addiction’ to sustained signalling, or the biology is critical within the DCI cycle time. We will continue to follow science and leverage our deep learning AI technology, biomolecular assays and expertise in bioinformatics to determine which diseases are potentially optimal targets for DCI.
Author Bio:
Brett Hall
Brett has been Chief Scientific Officer at Immuneering since November 2019. His extensive drug development and leadership experience have been instrumental to Immuneering’s success, including leading the research team in demonstrating for the first time the company’s novel deep cyclic inhibition mechanism for treatment of RAS-mutant solid tumours in humans in April 2023. Dr Hall is a former member of the US Air Force and a retired investment banker.
Related topics
Artificial Intelligence, Drug Development, Drug Discovery, Drug Discovery Processes, Drug Leads, Immuno-oncology, Immuno-oncology therapeutics, Oncology, Targets
Related conditions
Brain disease, Cancer, cancer treatment
Related organisations
immuneering
Related people
Brett Hall