AI in gene delivery vector discovery and design
Posted: 26 October 2023 | Dr Fernando Garces (BioMap) | No comments yet
Dr Fernando Garces, Director of Biotechnology and Innovation at BioMap, discusses the huge potential of AI to deliver successful cell and gene therapy products by optimised design of viral vectors.
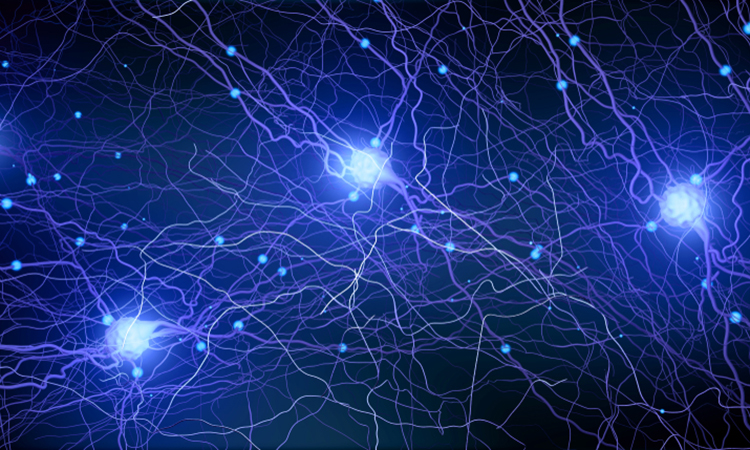
Genome engineering and gene therapies that manipulate DNA sequences in cells have driven a biotechnological revolution over the past decade.1 Adeno-associated virus (AAV) vectors are the leading platform for in vivo gene delivery for the treatment of various human diseases. Alipogene tiparvovec and voretigene neparvovec-rzyl, two recombinant AAV-based gene therapy products, were approved by the European Medicines Agency (EMA) to treat lipoprotein lipase deficiency in 20122 and by the US Food and Drug Administration (FDA) to treat inherited retinal dystrophy in 2017, respectively.3
Although AAV research has gained important achievements, from its genome configuration to virion assembly,4 the creation of cell-specific AAV capsids, a crucial step in realising their therapeutic potential, is still a longstanding challenge.5,6 Despite grafting receptor-targeting peptides to AAV capsids having shown huge potential for introducing the tropism,7,8 this may significantly change the original conformations of the peptides, due to their interactions with the capsids, and thus impact their binding affinities with target cells.9 In addition, host immune responses further add to the complexity of developing cell-specific AAV capsids for clinical applications.
It has been well established that exposure to wild-type AAV results in priming of the immune system against the virus. Ensuring diversity of newly designed AAVs is becoming essential to avoid the immune surveillance of patients due to previous AAV exposure. Although randomly shuffling the sequences between different AAV serotypes10 can diversify the variants of capsids, this has shown very low success in AAV capsid productions.
Artificial Intelligence- (AI-) driven AAV engineering
As biomedical research continues to advance, the discovery and design of gene delivery vectors has assumed critical importance, directly impacting progress in fields such as gene therapy and gene editing. The limitations of natural AAV serotypes in meeting all clinical needs has spurred growing interest in AI-driven AAV engineering approaches.
The limitations of natural AAV serotypes in meeting all clinical needs has spurred growing interest in AI-driven AAV engineering approaches.
Consequently, an increasing number of academic researchers and companies are entering this field. Many biotechnology start-ups, leveraging AI and high-throughput experiments, play a pivotal role in revolutionising gene therapy, while some traditional pharmaceutical giants have also displayed strong interest in this AI-driven AAV design approach. In this rapidly evolving field, numerous AI-designed AAV products are progressing towards the market.
Much like models such as ChatGPT that showcase AI’s vast capabilities in natural language processing and knowledge understanding, AI large language models (LLM) also hold immense potential in life sciences and pharmaceuticals. They can accelerate various aspects of drug research and development, providing novel opportunities for faster and more effective solutions to health challenges.
Much like models such as ChatGPT that showcase AI’s vast capabilities in natural language processing and knowledge understanding.
Large language AI models are now enabling the generation of functional proteins, where the technology can accurately model large numbers of protein designs, predict their characteristics and fitness for the designated task, and even predict their downstream effect within the cells and systems of the body. We are now seeing new methods using AI models to drive AAV discovery rapidly emerging.
The power of large foundation models
Using a foundation model approach does not require a ‘warmup’ library experiment, which typically supplies data for initial AI model training. For example, life science AI foundation model xTrimo, which stands for cross-model transformer representation of interactome and multi-omics, is a large language model that integrates billions of multi-dimensional data from the amino acid level to the bio system level to understand and predict the behaviour of life at multiple scales of complexity.
xTrimo maximises the capabilities of particularly large pre-trained foundation models and advanced AI infrastructure. These models have demonstrated high-performance design capabilities, enabling the emergence of highly effective designs. This approach has revealed several promising hit molecules, even in the absence of prior library data, providing an indication that this AI-driven approach could address numerous conventional hurdles impeding advancements in the delivery of gene therapies and gene editing.
AI-driven approach could address numerous conventional hurdles…
As data within the gene delivery vector domain continues to grow and with the emergence of large protein language models, it is anticipated that AI-driven discovery and design of delivery vectors will soon prove significant. Ensuring the ethical and safe application of these technologies is of paramount importance. Nonetheless, it is apparent that AI will play a pivotal role in gene delivery vector research, advancing a new era of innovation and significant breakthroughs for human health in the foreseeable future.
About the author
Dr Fernando Garces
Director of Biotechnology and Innovation at BioMap
Dr Garces boasts a distinguished career. Holding a bachelor’s degree in microbiology from the Catholic University in Porto, Portugal, he pursued international recognition during his PhD fellowship at the University of Barcelona, specialising in structural biology and biochemistry. Subsequent roles at the Institute of Cancer Research and The Scripps Research Institute solidified his expertise in protein design and vaccine development, leading to the 2015 Scripps CHAVI-ID Young Investigator Award.
Joining Amgen in 2016, Fernando pioneered a protein engineering team and contributed to industry-leading workflows for multi-specific antibodies. His mentorship produced numerous publications. In 2021, he assumed the role of Director in Protein Therapeutics at Gilead, driving biologics to prominence. With approximately 35 peer-reviewed publications and over 20 patents, Fernando’s contributions to biotechnology and innovation are profound.
References
- Doudna JA. The promise and challenge of therapeutic genome editing. Nature 578, 229-236 (2020).
- Ylä-Herttuala S. Endgame: glybera finally recommended for approval as the first gene therapy drug in the European union. Molecular Therapy 20, 1831-1832 (2012).
- Russell S, et al. Efficacy and safety of voretigene neparvovec (AAV2-hRPE65v2) in patients with RPE65-mediated inherited retinal dystrophy: a randomised, controlled, open-label, phase 3 trial. The Lancet 390, 849-860 (2017).
- Wang D, Tai PW, Gao G. Adeno-associated virus vector as a platform for gene therapy delivery. Nature reviews Drug discovery 18, 358-378 (2019).
- Nyberg WA, et al. An evolved AAV variant enables efficient genetic engineering of murine T cells. Cell 186, 446-460. e419 (2023).
- Deverman BE, et al. Cre-dependent selection yields AAV variants for widespread gene transfer to the adult brain. Nature Biotechnology 34, 204-209 (2016).
- Münch RC, et al. Off-target-free gene delivery by affinity-purified receptor-targeted viral vectors. Nature Communications 6, 6246 (2015).
- Münch RC, et al. Displaying high-affinity ligands on adeno-associated viral vectors enables tumor cell-specific and safe gene transfer. Molecular therapy 21, 109-118 (2013).
- Li C, Samulski RJ. Engineering adeno-associated virus vectors for gene therapy. Nature Reviews Genetics 21, 255-272 (2020).
- Grimm D, et al. In vitro and in vivo gene therapy vector evolution via multispecies interbreeding and retargeting of adeno-associated viruses. Journal of virology 82, 5887-5911 (2008).
Related topics
Artificial Intelligence
Related organisations
BioMap
Related people
Dr Fernando Garces (BioMap)