AI system develops features of complex brains
Posted: 15 December 2023 | Ellen Capon (Drug Target Review) | No comments yet
An AI system could be used to observe how physical constraints shape brains and impact people with cognitive difficulties.
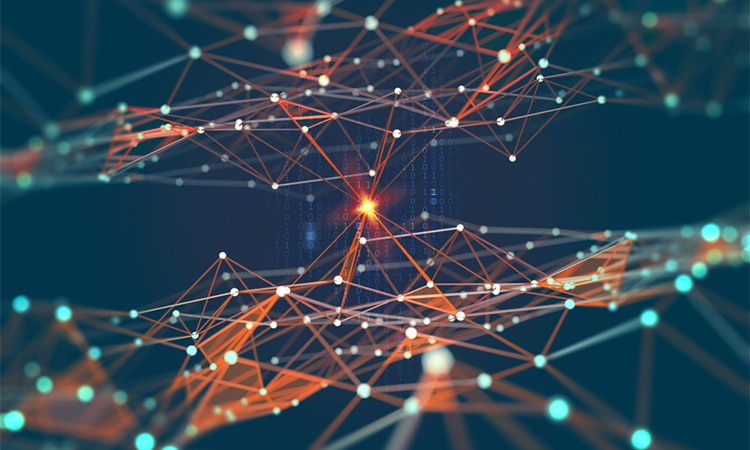
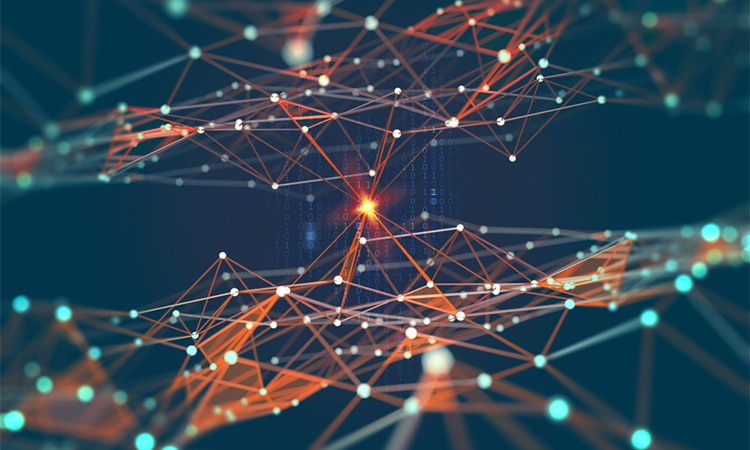
University of Cambridge scientists have demonstrated that putting physical constrains on an artificially intelligent system, similarly to how the human brain develops and functions within physical and biological constraints, enables it to develop features of complex organisms’ brains to solve problems.
Neural systems like brains must balance competing demands when they organise themselves and form connections, such as when resources are needed to grow and sustain the network in physical space whilst optimising the network for information processing. Within and across species, this competition happens which could explain why many brains converge on comparable organisational solutions.
A Gates Scholar from the Medical Research Council Cognition and Brain Sciences Unit (MRC CBSU) at University of Cambridge Dr Jascha Achterberg said: “Not only is the brain great at solving complex problems, but it does also so while using very little energy. In our new work we show that considering the brain’s problem-solving abilities alongside its goal of spending as few resources as possible can help us understand why brains look like they do.”
Dr Danyal Akarca, co-lead author also from MRC CBSU, noted: “This stems from a broad principle, which is that biological systems commonly evolve to make the most of what energetic resources they have available to them. The solutions they come to are often very elegant and reflect the trade-offs between various forces imposed on them.”
Applying physical constraints
Dr Achterberg, Dr Akarca and their colleagues created an artificial system aimed at modelling a simplified version of the brain and applied physical constraints. They discovered that their system developed some key characteristics like those found in human brains.
The system used computational nodes instead of real neurons. Nodes and neurons function similarly, as they both have an input, transform it, and produce an output. A single node or neuron could connect to many others which all input information to be computed.
To apply a physical constraint, each node was given an exact location in a virtual space. The further away two nodes were, the harder it was for them to communicate, which is like how human brains’ neurons are organised.
The task that the scientists gave the system was a simplified version of maze navigation, often given to rats and macaques when studying the brain. For example, a study by researchers at Porto Medical School addressed the issue of whether or not cytotoxic damage to the retrosplenial cortex (RC) impairs place navigation of Wistar rats in the Morris water maze and, if so, whether this is merely attributable to spatial learning deficits or to impaired learning of general (nonspatial) behavioural strategies required to correctly perform this task or both.1
For maze navigation, multiple pieces of information need to be combined to decide on the shortest route to the end point. The AI system is required to maintain multiple elements, such as start location, end location and intermediate steps. Once it has learnt to do the task reliably, the researchers could observe which nodes are used at different moments in the trial, as one cluster of nodes may encode available routes.
Real human brains and the system use the same tricks when required to perform the task. The system began to make hubs, highly connected nodes that act like conduits for passing information across the network. More surprisingly, the response profiles of individual nodes changed to have a flexible coding scheme, meaning at different moments, nodes could be firing for a mix of properties in the maze. For example, the same node could encode multiple locations of a maze, instead of needing specialised nodes for encoding specific locations, which is another feature seen in the brains of complex organisms.
Understanding cognitive difficulties
This AI system may start to uncover how these constraints shape differences between people’s brains and impact the differences observed in people experiencing cognitive or mental health difficulties.
“We can train the system to perform tasks and then play around experimentally with the constraints we impose, to see if it begins to look more like the brains of particular individuals.”
Dr John Duncan, co-author from the MRC CBSU, explained: “These artificial brains give us a way to understand the rich and bewildering data we see when the activity of real neurons is recorded in real brains.”
Dr Achterberg noted: “Artificial ‘brains’ allow us to ask questions that it would be impossible to look at in an actual biologic system. We can train the system to perform tasks and then play around experimentally with the constraints we impose, to see if it begins to look more like the brains of particular individuals.”
Future AI systems
This study will be interesting for the AI community, as it could influence the development of more efficient systems, especially in situations where there is a likelihood of physical constraints. Dr Akarca explained: “AI researchers are constantly trying to work out how to make complex, neural systems that can encode and perform in a flexible way that is efficient. To achieve this, we think that neurobiology will give us a lot of inspiration. For example, the overall wiring cost of the system we’ve created is much lower than you would find in a typical AI system.”
There are many AI solutions that currently use architectures that only superficially resemble a brain. Dr Achterberg commented: “If you want to build an artificially intelligent system that solves similar problems to humans, then ultimately the system will end up looking much closer to an actual brain than systems running on large compute cluster that specialise in very different tasks to those carried out by humans.”
“They need to constantly process new information coming in through their sensors while controlling their bodies to move through space towards a goal.”
Robots would benefit from having brain structures like humans, if they must process a large amount of constantly changing information with finite energetic resources. Dr Achterberg explained: “Brains of robots that are deployed in the real physical world are probably going to look more like our brains because they might face the same challenges as us. They need to constantly process new information coming in through their sensors while controlling their bodies to move through space towards a goal.”
He continued: “Many systems will need to run all their computations with a limited supply of electric energy and so, to balance these energetic constraints with the amount of information it needs to process, it will probably need a brain structure similar to ours.”
The study was published in Nature Machine Intelligence.
Reference
1 Andrade JP, Lukoyanov NV, Lukoyanova EA, Paula-Barbosa MM. Impaired water maze navigation of Wistar rats with retrosplenial cortex lesions: effect of nonspatial pretraining. Behavioural Brain Research. 2005 March 7 [2023 November 22]; 158(1):175-82. Available from: https://www.sciencedirect.com/science/article/abs/pii/S0166432804003602
Related topics
Artificial Intelligence, Neurons, Neurosciences
Related conditions
cognitive disorders, Mental Health
Related organisations
University of Cambridge