Unlocking the potential of natural products in drug discovery
Posted: 28 March 2024 | August Allen (Enveda Biosciences), Erica Forsberg (Bruker Life Sciences), Pelle Simpson (Enveda Biosciences) | No comments yet
Natural products play an underappreciated role in drug discovery. Tandem mass spectrometry (MS/MS)-based metabolomics coupled with machine learning is allowing new, highly diverse molecules from natural products to be identified, revealing bioactive compounds and pinpointing promising drug targets. The additional dimension provided by trapped ion mobility (TIMS) enables researchers to increase the speed, sensitivity and specificity of metabolite measurement.
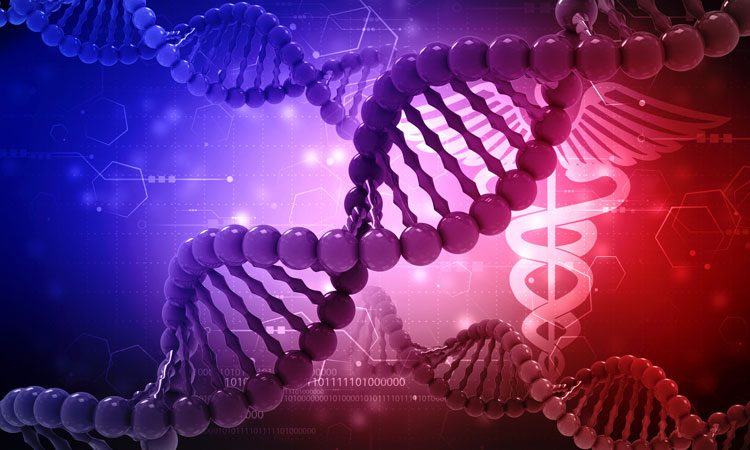
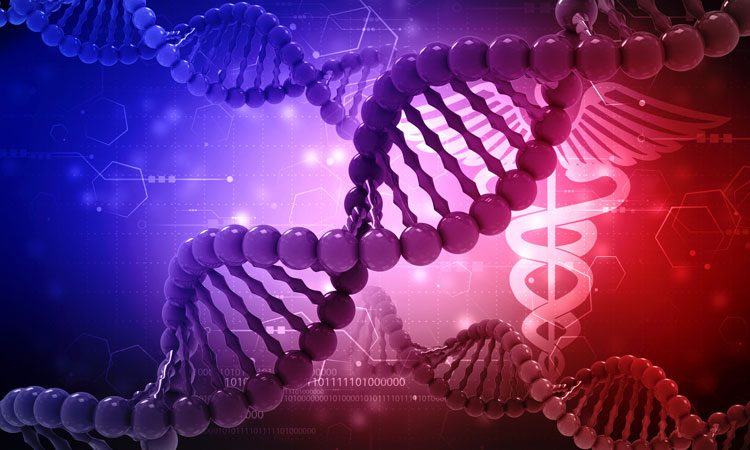
Between 2000 and 2020, approximately 30 percent of the newly introduced small molecule drugs were derived from natural products.1 Surprisingly, however, less than one percent of the natural world’s chemical composition has been identified or studied by scientists. While plant-derived molecules (phytochemicals) have long played an essential role in drug discovery due to their diverse biological activities and chemical properties, the reliance on time-consuming and expensive screening techniques has historically hindered progress. In recent decades there has been a decline in interest in natural products for drug discovery, with the industry gravitating towards screening libraries of synthetic molecules with predefined chemistries. A new breed of biotechs like Enveda Biosciences are re-energising the field of natural product drug discovery by integrating TIMS-MS/MS-based metabolomics and machine learning to speed up drug discovery.
Why focus on natural products and phytochemicals?
Some of the most widely prescribed and powerful medicines on the planet are natural products, including aspirin, Taxol, metformin, and artemisinin. These examples highlight the broad utility of natural products, given their use in treating inflammation, cancer, diabetes, and malaria, respectively. Bioactive phytochemicals exhibit a wide range of effects on the human body and metabolism, and this diversity in function is encoded in their diverse chemistry. In a 2022 review, the authors posited that natural product drugs, or those closely mimicking natural products, may possess conserved recognition elements and associated properties, functioning in evolutionarily optimized ways. In short, this hypothesis underscores the potential benefits of aligning pharmaceutical development more closely with nature.1 Researchers have also observed a correlation between greater translatability of natural products and increased progression through clinical trials. Notably, when complex chemical samples derived from natural sources are subjected to advanced analytical technologies for recall of known structures, fewer than 10 percent return a match.3 This indicates there is a large pool of undiscovered natural products with therapeutic promise waiting to be mined.
Accelerating characterisation
While there is substantial evidence supporting natural products as a high-quality source of potential drug leads, the process of discovering such molecules presents various challenges, including the scope of unknown chemistry and biological function.
While established techniques require isolating and studying natural products one at a time, Enveda has developed modern MS/MS-based approaches that can enable high-throughput assessment of biological activity, chemical structure and drug-like properties directly from mixtures, speeding up the characterisation step and advancing the most promising molecules for further study. This can allow researchers to routinely uncover about 10,000 distinct molecules per plant sample, each of which has the potential to interact with human biology and inspire new medicines.
In its new workflow, liquid chromatography (LC) is used to separate complex mixtures into fractions. A portion of each fraction is analysed using trapped ion mobility tandem mass spectrometry (TIMS-MS/MS) to identify chemical structure, while the other portion is tested in different biological assays to determine bioactivity. Molecules that are found to have the right combination of beneficial activity, low toxicity, and amenable structures are then prioritised for isolation and further characterisation.
TIMS and MS/MS: a powerful combination
The isolation of individual compounds from complex mixtures can make the drug discovery process slow, expensive and prone to failure. The key to identifying natural product structures is the combination of clean MS/MS fragmentation patterns and trapped ion mobility data.
TIMS-MS/MS-based metabolomics can be used to separate a mixture of thousands of compounds extracted from a natural source. Since the vast majority of these compounds do not have known structures, it is imperative to acquire as much high-quality data as possible to help identify them. Understanding the structure of a candidate molecule is essential for determining whether it has the required properties of a drug. Typical MS/MS experiments provide data on accurate mass, isotope patterns, and fragmentation patterns to identify unknowns. However, many natural product compounds have very similar (isobars) or identical (isomers) accurate masses. These can be challenging to separate with LC and often co-elute. When isobars and isomers co-elute, the MS/MS data generated are a mixture of multiple compounds that become extremely difficult to parse. This is where TIMS technology comes into play.
TIMS technology separates molecules based on their size and shape, which provides an additional dimension of separation for isobars and isomers. The result is cleaner MS/MS data that can be associated with the molecular structure of a compound. Additionally, TIMS measures collisional cross section (CCS) values, a physical property of an ion that does not change based on the ionization source, so every measurement is a unique characteristic of that molecule.
TIMS is coupled with MS/MS fragmentation in a mode called parallel accumulation serial fragmentation (PASEF). As mobility separated ions elute from the TIMS cell, they are sent to the time of flight (TOF) mass analyser, either directly to collect accurate mass data for the precursor (MS1) or fragmented to collect clean mobility-separated MS/MS data (MS2). Fragmentation patterns are acquired rapidly in data dependent acquisition (DDA) PASEF to give high MS/MS coverage across more molecules. The greater the MS/MS coverage, the more structural information is acquired. When this data is analysed by machine learning models, such as the de novo structure prediction model designed and built by Enveda, MS2Mol,4 fragmentation patterns can be used to predict structure for a given molecule. Structural information is then combined with biological assays to identify the compounds that have the most potential for therapeutic development.
The future of drug discovery
New ways of analysing the data produced by high resolution mass spectrometry using machine learning hold the key to identifying natural product structures. This powerful combination of technologies is enabling a paradigm shift in natural product discovery, from one molecule at a time, to 10,000 molecules at a time. This speed and depth of analysis overcomes the core challenges of working with natural products, allowing researchers to capitalise on their substantial benefits.
About the authors
August has a decade of experience building high throughput automation, scaling teams, and solving problems at the intersection of technology and life sciences. He was an early employee of Recursion Pharmaceuticals, where he scaled their high-content imaging platform by multiple orders of magnitude before joining Enveda in 2021. At Enveda, he is responsible for overseeing the company’s platform to annotate the structure and function of the natural world to discover new medicines.
Pelle has a background in chromatography and mass spectrometry, initially focusing on natural products drug discovery and cell signaling proteomics at the University of Amsterdam. He expanded his research in proteomics at the University of Colorado, Boulder, where he also developed interests in 3D printing, machining, and programming for lab automation. Since joining Enveda Biosciences in 2021, he has leveraged these skills to develop custom hardware and software to enable the company’s mass spectrometry and high throughput screening efforts.
Dr Forsberg manages the metabolomics business for Bruker Life Sciences Mass Spectrometry. She came to Bruker in 2021 from a faculty position in the Department of Chemistry at San Diego State University following a post-doctoral fellowship at The Scripps Research Institute in Gary Siuzdak’s group. Her major focus has been untargeted metabolomics method development and bioinformatics solutions for structure elucidation. Her current role involves managing the development and implementation of Bruker’s future metabolomics and lipidomics solutions.
References
- Newman DJ, Cragg GM. Natural products as sources of new drugs over the nearly four decades from 01/1981 to 09/2019. Journal of Natural Products. 2020;83(3):770–803. doi:10.1021/acs.jnatprod.9b01285
- Young, R.J., Flitsch, S.L., Grigalunas, M., Leeson, P.D., Quinn, R.J., Turner, N.J. and Waldmann, H. (2022). The Time and Place for Nature in Drug Discovery. JACS Au, 2(11), pp.2400–2416. doi:https://doi.org/10.1021/jacsau.2c00415.
- Butler T, Frandsen A, Lightheart R, Bargh B, Kerby T, West K, et al. MS2Mol: A transformer model for illuminating dark chemical space from mass spectra. ChemRxiv. 2023; doi:10.26434/chemrxiv-2023-vsmpx-v4 (preprint)
Related topics
Artificial Intelligence, Machine learning