Kickstarting the use of AI for biotechs: part three
Posted: 20 June 2024 | Dr Raminderpal Singh (Hitchhikers AI and 20/15 Visioneers) | No comments yet
Traditional wet lab scientists working on target discovery, drug identification and drug optimisation have an opportunity to catch up with their AI-enabled peers – but why should they, and how? In this article – the third of a three-part series – Dr Raminderpal Singh touches on the decisions that need to be made and their potential risks. The key is to start with a data strategy.
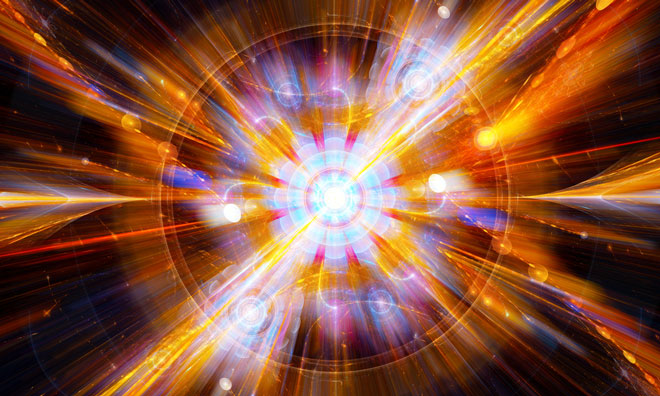
Early-stage biotechs are focused on survival until wet lab and/or animal data is generated that excites investors. Many biotech CEOs and CSOs are asking, often sceptically, whether computational methods (dry lab) can help them gain the required data faster and more cheaply. Algorithms could substitute for parts of the wet lab workflows, helping to reduce the number of experiments conducted by contract research organisations (CROs), by qualifying and prioritising them.
Often, but not always, there is a bioinformatician in the biotech team, who has experience in crunching data. While this experience is valuable, giving them a sense of the work required and the nature of the data, it can also be dangerous because their background with AI techniques and the availability of vendor tools is often limited. Furthermore, given that biotechs have relatively little funding available for computational tools, investments are often aimed at short-term wins; for example, Python scripts that take in study datasets and output interesting correlations – some nice graph plots are often helpful. This highlights that a more broad-based approach is needed.
However, whichever approach you take, you may spend a lot of time reaching the conclusion that your data is not good enough for even simple analyses. Your first investment must be in your data strategy – understanding and developing the quality and utility of your wet lab (and/or animal) data.
Please see below for the initial recommended steps:
- Assess your data quality and utility and develop a data build vs buy strategy. Some limited knowledge of AI/statistical techniques will be needed for this, as it affects the utility calculation; this knowledge can be gained through experienced advisors.
- Build a six to 12 months computational strategy that leverages current data and planned new data. Be open to paying for datasets and tools, and even look at open-source software if you have the in-house software skills. Each phase of the strategy should be targeted at helping answer key scientific questions that your investors are expecting you to answer.
Importantly, take some risk in going after these steps; you are already taking risk in your wet lab analyses! Use this opportunity to develop relationships with data partners and tool vendors, as well as the life-sciences data science community.
In addition to the above considerations, there is much that can be done quickly with large language models (LLMs), although the use of GenAI is nuanced and the value unpredictable. Also, LLM providers use your search terms to train their systems and ultimately share the search terms with other users. The pragmatic ways to avoid these constraints and create actionable value with LLMs will be explored in the next article in this series, going live Monday 24 June.
As a final point, it is important to remember with all the AI and data available, that the scientist must stay at the centre of the universe – see the figure below. Tools and data are only as good as the insights they generate; the creativity and invention will come from the scientists.
About the author
Dr Raminderpal Singh
Dr Raminderpal Singh is a recognised key opinion leader in the techbio industry. He has over 30 years of global experience leading and advising teams on building computational modelling systems that are both cost efficient and have significant IP value. His passion is to help early to mid-stage life sciences companies achieve novel biological breakthroughs through the effective use of computational modelling.
Raminderpal is currently leading the HitchhikersAI.org open-source community, accelerating the adoption of AI technologies in early drug discovery. He is also CEO and co-Founder of Incubate Bio – a techbio providing a service to life sciences companies who are looking to accelerate their research and lower their wet lab costs through in silico modelling.
Raminderpal has extensive experience building businesses in both Europe and the US. As a business executive at IBM Research in New York, Dr Singh led the go-to-market for IBM Watson Genomics Analytics. He was also Vice President and Head of the Microbiome Division at Eagle Genomics Ltd, in Cambridge. Raminderpal earned his PhD in semiconductor modelling in 1997. He has published several papers and two books and has twelve issued patents. In 2003, he was selected by EE Times as one of the top 13 most influential people in the semiconductor industry.
For more: http://raminderpalsingh.com ; http://hitchhikersAI.org ; http://incubate.bio
Related topics
Artificial Intelligence
Related organisations
HitchhikersAI