AI paves the way for new immunotherapy targets
Posted: 11 December 2024 | Michelle Teng - CEO of Etcembly | No comments yet
To fully unlock the potential of immunotherapy for cancer patients, we need better targets, not just better treatments. Hear from Michelle Teng, CEO of Etcembly, on how AI is decoding the immune repertoire and designing new therapies.
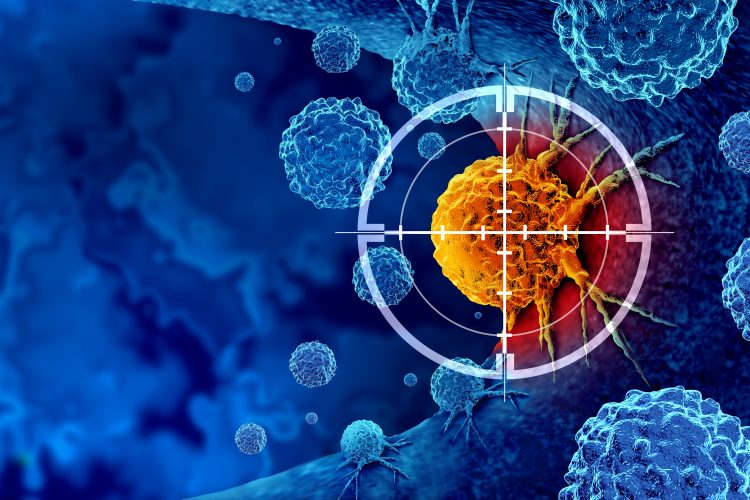
The impact of immunotherapy in oncology over recent years is undeniable. Novel therapeutic approaches have led to durable responses in some patients with metastatic disease, with some even experiencing results similar to a cure. Yet they do not work for everyone, and we still have a long way to go to fully harness the immune system’s ability to fight cancer.
Unlocking this potential requires a dual approach:
- Identifying suitable targets within tumours and developing effective biomarkers for patient stratification.
- Discovering and optimising potent therapeutics that direct the immune response against these targets.
This requires a deep understanding of how the key components of the immune system – antibodies and TCRs – recognise and interact with their targets on cancer cells. Advances in computing and single-cell sequencing now make it possible to explore this interface in real-time, accelerating the next wave of immunotherapy innovation.
From checkpoint inhibitors to TCR-based targeting
The first wave of immunotherapy introduced checkpoint inhibitors like PD1/PD-L1 and CTLA4, allowing T cells to recognise and destroy tumours. While transformative for some, these therapies have shown limited efficacy across the wider patient population and can cause side effects.1,2 Similarly, CAR T cell therapies have shown success in treating some blood cancers, but the complexity, cost, and delivery of cell therapies make them inaccessible for many patients.3
In response, there is growing interest in soluble bispecific T cell engagers – drugs designed to bind a cancer target with one ‘arm’ and recruit a T cell with the other.4 However, bispecific immune engagers made from antibodies can only recognise targets on the cell surface, limiting their effectiveness.
An emerging alternative is using T cell receptors (TCRs) for tumour targeting. TCRs recognise intracellular antigens displayed on the cell surface via the HLA complex, significantly expanding the range of potential targets.5 In contrast to antibodies, discovering and optimising TCRs to ensure they are both safe and effective for therapeutic use remains a significant challenge.6
The development of these therapeutics depends on identifying suitable targets that are highly expressed in tumours but absent in healthy tissue. Reliance on a narrow set of targets (eg PRAME and MAGEA4) has slowed progress, highlighting the urgent need for new targets to drive the development of a new wave of immunotherapies.
Decoding the immune repertoire to reveal novel immunotherapy targets
Conventional discovery approaches usually use multi-omic methods to analyse the molecular makeup of tumours. However, there is no guarantee that a target found in this way will be recognised by the immune system and trigger an effective response.
An alternative and potentially more effective strategy is to start from a position of success, by studying the immune systems of cancer survivors and reverse-engineer the immune responses that led to remission—a method known as de-orphanization.7
Recent technological advances now make it possible to generate the vast training datasets cost-effectively required for this task. Microfluidic single-cell sequencing platforms can capture millions of antibody and paired TCR sequences from blood and tumour samples of cancer survivors. This data provides a unique foundation for building models that map the immune-cancer interface in unprecedented detail, identifying not only novel targets, but also therapeutic strategies for eliminating them.
Sequence is not enough: Structure is key
Sequence data alone isn’t enough to identify potential targets and therapeutics. The interface between an antibody or TCR and its target is a complex multi-partite three-dimensional structure. Accurately mapping this interface is essential for understanding immune recognition and response in disease, as well as for developing more effective treatments.
Much attention has been given to the Nobel prize-winning advancements in predicting protein structures from amino acid sequences, particularly AlphaFold3.8 While AlphaFold is useful for predicting some TCR domains, there is currently no accessible version that can accurately model the complete TCR-antigen-HLA complex for TCRs that are not in the public domain.
Generative AI algorithms, known as large language models (LLMs), are useful for predicting and manipulating interactions between antibodies, TCRs and their targets. While LLMs are most commonly associated with consumer tools like ChatGPT, they can be trained on any text-based dataset, including DNA and protein sequences. In this context, they can identify patterns and correlations in immune responses to pinpoint potential targets.
Going a step further, LLMs can also predict and iterate upon this information, rapidly generating plausible TCRs or antibodies with specificity and affinity characteristics suitable for therapeutic use. These can then be built and tested in the lab, with the resulting data fed back into the model for further improvement and optimisation.
AI-first approach to immunotherapy
Platforms that combine large-scale immune repertoire data with advanced LLMs and structural modelling capabilities are set to transform our understanding of the immune system and its response to cancer. These platforms could also be applied to other diseases with a strong immunological component, such as autoimmune conditions.
In the future, we expect to see such platforms deliver true in silico de-orphanization for TCRs and antibodies by predicting the identity and structure of a target antigen based solely on their sequence. This approach has the potential to identify new targets more accurately than conventional identification methods, as well as spotting common themes across different cancers.
For example, our own LLM-based generative AI engine, EMLyTM already outperforms AlphaFold3 in predicting the structure of the TCR: HLA interface from the sequence, and is constantly improving as we feed in more data from our Long Term Survivor Study (as shown in Figure 1).10
Figure 1: An image showing the close agreement between the TCR-antigen-HLA complex crystal structure and the model predicted by Etcembly’s EMLy platform. A) Structure alignment. The modelled complex (magenta) is aligned on the crystal structure alpha-carbon of the HLA (cyan). B) Top view showing detail of the CDRs, comparing the crystal structure (top) and the model (bottom).
We are now working to open these tools and capabilities to the research community, harnessing intuitive co-pilot technology to enable real-time interrogation and manipulation of antibodies and TCRs. Just as AI is transforming fields like image analysis, multi-omics, and small molecule drug design, today’s technological advances are set to revolutionise immunology, bringing us closer to unlocking the full potential of the immune system in the fight against cancer and other diseases.
References:
- Fitzsimmons TS, Singh N, Walker TDJ, Newton C, Evans DGR, Crosbie EJ, et al. Immune checkpoint inhibitors efficacy across solid cancers and the utility of PD-L1 as a biomarker of response: a systematic review and meta-analysis. Frontiers in Medicine 2023 May 12;10.
- Johnson DB, Nebhan CA, Moslehi JJ, Balko JM. Immune-checkpoint inhibitors: long-term implications of toxicity. Nature Reviews Clinical Oncology. 2022 Jan 26;19(4):254–67.
- Cappell KM, Kochenderfer JN. Long-term outcomes following CAR T cell therapy: what we know so far. Nature Reviews Clinical Oncology. 2023 Apr 13;20(6):359–71.
- Rolin C, Zimmer J, Seguin-Devaux C. Bridging the gap with multispecific immune cell engagers in cancer and infectious diseases. Cellular and Molecular Immunology. 2024 May 24;21(7):643–61.
- Klebanoff CA, Chandran SS, Baker BM, Quezada SA, Ribas A. T cell receptor therapeutics: immunological targeting of the intracellular cancer proteome. Nature Reviews Drug Discovery. 2023 Oct 27;22(12):996–1017.
- Baulu E, Gardet C, Chuvin N, Depil S. TCR-engineered T cell therapy in solid tumors: State of the art and perspectives. Science Advances. 2023 Feb 15;9(7).
- Dolton G, Rius C, Wall A, Szomolay B, Bianchi V, Galloway SAE, et al. Targeting of multiple tumor-associated antigens by individual T cell receptors during successful cancer immunotherapy. Cell. 2023 Jul 24;186(16):3333-3349.e27.
- Abramson J, Adler J, Dunger J, Evans R, Green T, Pritzel A, et al. Accurate structure prediction of biomolecular interactions with AlphaFold 3. Nature. 2024 May 8;630(8016):493–500.
- Madani, A., Krause, B., Greene, E.R. et al. Large language models generate functional protein sequences across diverse families. Nature Biotechnology. 2023 41:1099–1106
- Etcembly Long Term Survivor study. Etcembly. Accessed 2024 Oct 28, https://www.etcembly.com/long-term-survivor-study
About the author
Dr Michelle Teng, CEO of Etcembly
Michelle Teng is the co-founder and CEO of Etcembly, a techbio company based in Oxford, UK, that is revolutionizing immunotherapy drug development with speed and precision. Etcembly recently launched the world’s first TCR designed using Generative AI, setting a new standard in targeted therapeutics. The company is also leading the Long Term Cancer Survivor initiative, a global project to sequence immune repertoires of cancer survivors, aiming to develop next-generation cancer therapies.
Related topics
Artificial Intelligence, Drug Discovery Processes, Drug Targets, Immuno-oncology, Immunotherapy, Sequencing
Related conditions
Cancer
Related organisations
Etcembly
Related people
Michelle Teng