How AI will reshape pharma in 2025
Posted: 20 December 2024 | Carrie Haslam | No comments yet
AI is set to transform drug development in 2025, streamlining processes and opening new possibilities. Learn how this technology is transforming clinical trials and reshaping the pharmaceutical industry.
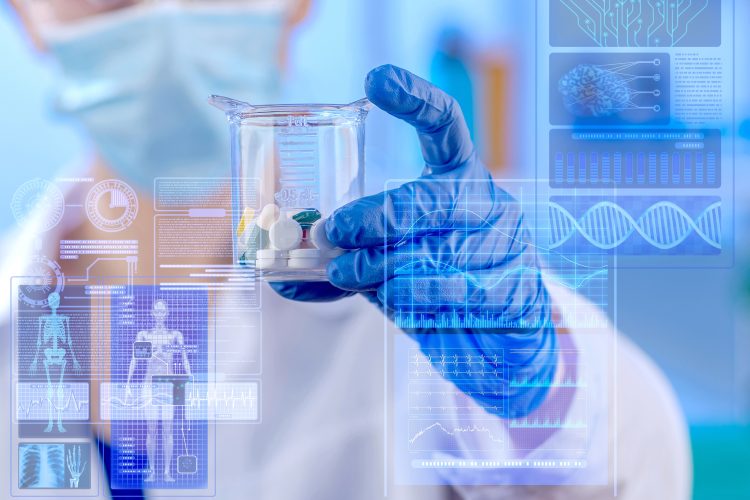
Artificial intelligence (AI) has revolutionised many industries, yet its adoption in pharmaceutical drug development has been notably slower. Concerns surrounding data security, the complexity of AI systems, and the rigorous regulatory frameworks in this field have caused the pharmaceutical industry to approach these technologies with caution. For years, AI and machine learning (ML) were often dismissed as little more than advanced statistics with little practical value in drug development.
However, recent breakthroughs in AI, such as predictive modelling, clinical trial optimisation, and personalised medicine, have demonstrated its potential. These innovations have started to shift industry perceptions, positioning AI as a transformative tool that could alter how drugs are developed, tested, and brought to market.
Looking ahead, 2025 could represent a major turning point for the pharmaceutical sector. We spoke with Aaron Smith, a mathematician-turned-machine learning scientist and the founder of Unlearn, a company leading the charge in applying AI to optimise clinical trial efficiency. At the heart of Unlearn’s approach is the use of AI to improve clinical trials, especially in phases two and three. Their work focuses on creating ‘digital twin generators’ – AI-driven models that predict how a patient’s disease may progress over time. These digital twins allow pharmaceutical companies to design clinical trials with fewer participants, while still providing reliable evidence to assess a drug’s effectiveness. This innovation could greatly reduce both the cost and duration of clinical trials, addressing two major challenges in drug development.
From distrust to collaboration
Despite the growing recognition of AI’s value, its adoption within the pharmaceutical industry faces several challenges. Smith identifies three key obstacles preventing broader acceptance of AI:
- Communication gaps: Pharmaceutical and computational science communities often struggle to understand each other’s technical languages, making collaboration difficult.
- Trust issues: Concerns about data security, algorithmic bias, and the reproducibility of AI’s predictions contribute to hesitation among stakeholders.
- Knowledge gaps: Several people in the pharmaceutical sector still have a limited understanding of AI’s capabilities and limitations, as well as the specific risks and benefits associated with its various applications.
While AI has great potential, the pharmaceutical industry has been slower to embrace it due to cultural and institutional challenges rather than scientific ones. Issues such as a lack of trust, limited understanding, and poor communication between AI companies and the pharmaceutical sector have often hindered progress. However, as the capabilities of AI tools such as large language models (LLMs) become apparent, attitudes are beginning to change. As Smith puts it, “now the viewpoint is that computational science is very powerful, and these AI/ML methods are going to be transformative in a lot of ways.”
We’ve definitely come across people in the pharma industry who worry that AI means companies might steal our data or use it in harmful ways.
A key challenge is the pharmaceutical industry’s reluctance to trust AI, mainly because of concerns surrounding data misuse. Many worry that AI companies could misuse sensitive patient data for purposes other than intended. Smith states, “we’ve definitely come across people in the pharma industry who worry that AI means companies might steal our data or use it in harmful ways.” However, he believes that these fears will dissipate as pharma companies come to realise that AI firms like Unlearn adhere to stringent data control measures, ensuring the ethical use of patient information. As the industry gains more exposure to AI’s real-world applications and the rigorous standards AI companies adhere to, trust will continue to grow.
AI-powered digital twins
A key component of Unlearn’s work is its focus on digital twin technology, which uses AI to create personalised models of disease progression for individual patients. These ‘twins’ simulate how a patient’s condition might evolve without treatment, enabling researchers to compare the real-world effects of an experimental therapy against predicted outcomes. By proving that their digital twin models can significantly reduce the number of subjects needed in clinical trials – without compromising trial integrity – Unlearn is making a compelling case for the value of AI.
Smith explained that the use of AI in clinical trials does not introduce additional risks, particularly regarding the approval of ineffective drugs. “Regulators care a lot about controlling the Type 1 error rate of the clinical trial,” Smith notes. “Even if the model we used has some error, it could not increase the Type 1 error rate of the trial. So, it’s about putting guardrails around any potential risks.”
AI to cut costs and speed recruitment in trials
The practical benefits of AI in clinical trials are already becoming clear. Unlearn’s technology has the potential to significantly reduce the size of control arms in phase three trials. This reduction not only saves money, particularly in costly therapeutic areas like Alzheimer’s, where trial costs can exceed £300,000 per subject but also speeds up patient recruitment. By increasing the chances that more participants will receive the treatment being tested, recruitment becomes more efficient, speeding up the overall trial process.
In 2025, I think we’ll see breakthroughs that allow us to make significant advances in rare diseases, where the data is small.
As AI adoption increases, clinical trials, traditionally the most resource-intensive and time-consuming phase of drug development, are set to benefit the most. AI tools are positioned to address long-standing inefficiencies in clinical trials, with significant advancements expected by next year. While current breakthroughs are impressive, Smith anticipates even greater advancements by 2025. He believes that improving data efficiency by training powerful AI models with less data will drive the next wave of innovation in clinical trials. This is particularly important in areas such as rare diseases or niche cancer subtypes, where data is scarce. By enabling AI to apply the same insights from large datasets to smaller, specialised datasets, we could see a revolution in drug development in these fields.
“Approaches that improve data efficiency will drive this space,” Smith explains. “In 2025, I think we’ll see breakthroughs that allow us to make significant advances in rare diseases, where the data is small.”
The future of pharma
By 2025, Smith believes the pharmaceutical industry will have fully embraced AI – not just as a theoretical concept, but as a valuable tool that dramatically improves the efficiency of drug development. The coming years could witness a cultural and institutional revolution as the industry becomes more comfortable with the risks and rewards of AI. With companies like Unlearn leading the way, AI’s role in drug development will continue to evolve, offering opportunities to improve patient outcomes and accelerate the discovery of new treatments. As Smith concludes, “it’s not going to be a scientific revolution, it’s going to be an institutional industry revolution.” As a result, 2025 could be the year AI truly transforms the pharmaceutical sector.
Aaron Smith, Founder & Machine Learning Scientist at Unlearn
Aaron Smith is a mathematician with research interests at the interface of computer vision, machine learning, physics, and geometry. Previously, Aaron was an algorithm engineer and manager of the hand tracking team at Leap Motion. He was a postdoctoral researcher in mathematics at the University of Waterloo. Aaron received his Ph.D. in mathematics from The University of Pennsylvania for work on aspects of differential topology and geometry inspired by theoretical physics.
Related topics
Artificial Intelligence, Big Data, Biopharmaceuticals, Clinical Trials, Computational techniques, Drug Development, Drug Discovery, Machine learning, Personalised Medicine, Translational Science
Related conditions
Alzheimer's disease (AD), Cancer
Related organisations
Unlearn
Related people
Aaron Smith (Founder of Unlearn)