Scientific workflow for hypothesis testing in drug discovery: Part 2 of 3
Posted: 14 January 2025 | Dr Raminderal Singh (Hitchhikers AI and 20/15 Visioneers, Nina Truter | No comments yet
In part two of the step-by-step scientific workflow for drug discovery series, Dr Raminderpal Singh and Nina Truter describe the functions of the workflow previously outlined and include key considerations.
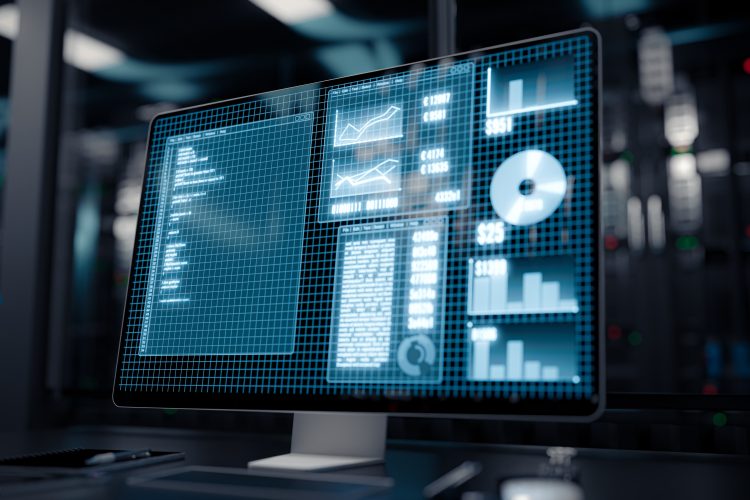
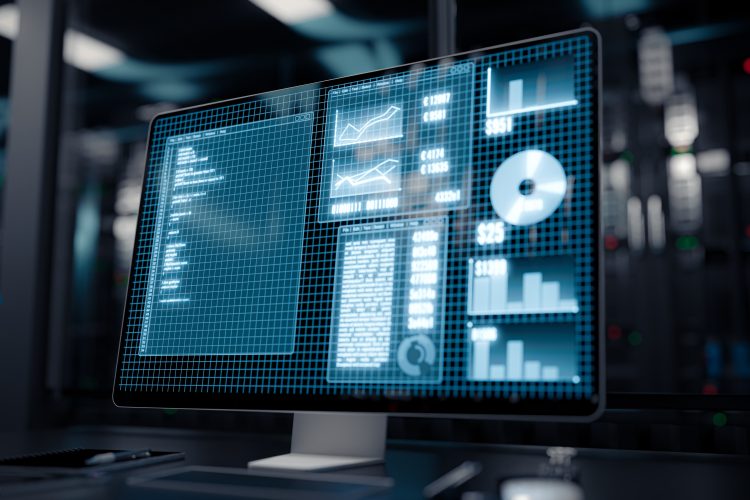
Drug discovery scientists spend their days developing and testing complex hypotheses, leveraging data and expertise through workflows that utilise available tools. Operating a workflow, such as the one described in Figure 1, involves several key considerations that affect both the accuracy of results and the efficiency of the research process.
Defining the research question
A well-defined research question is the cornerstone of an effective scientific workflow in drug discovery. The more specific your question, the easier it becomes to identify relevant data and design subsequent steps in your workflow. This initial phase often involves an iterative process: refining your question, conducting a literature review, and assessing available data to ensure the right level of specificity and relevance of your research question. AI tools like ChatGPT can help refine your question and provide an overview of the research landscape before commencing a full literature review.
Hypothesis generation
The hypothesis generation process is equally important. Before undertaking data analysis, a hypothesis must be developed based on literature reviews and public datasets. While the scientific question guides the entire investigation, without a clear hypothesis, the research could become unfocused and exploratory. Having a well-defined hypothesis allows researchers to assess datasets critically and ensures that their analysis remains grounded in the biological context. Creating a rough map containing the relevant variables that influence the outcome of the scientific question based on literature review and logic can help structure the hypothesis. This map can be used as a ‘checklist’ when assessing whether a dataset contains the necessary variables to answer the research question.
Data identification
When searching for public data, tools like Perplexity.ai can help identify relevant databases. By posing questions such as, ‘Which database should I use to search for data on the effects of longevity drugs in rodents?’ you can obtain more accurate, fact-based answers, whereas ChatGPT and Claude.ai provide more general information. Google Dataset Search and PubMed’s ‘Associated Data’ feature can identify datasets linked to publications. After discerning a potentially useful dataset, Claude.ai can summarise experimental methods to determine if the dataset is the right fit for your research question. Creating a descriptive spreadsheet to catalogue potential datasets, along with a broad description of their contents, helps streamline the selection process. In some cases, combining multiple datasets may be necessary to comprehensively address your research question.
Understanding data
Before initiating analysis, ample time should be spent reviewing the raw data. Browsing through datasets, often in Excel format, can clarify how the data were generated, helping you choose appropriate analysis methods and the requisite sanity checks. For data types that are less familiar, ChatGPT can be helpful in explaining the experimental method and for establishing potential validation steps. Alternatively, search for review papers or papers using a similar method and understand how it was applied in that context.
Visualisation is another powerful tool for understanding data and experimenting with different methods can provide varied perspectives. ChatGPT can also aid in deciding which visualisation options are available and what information each will provide, based on the data and your research question. Additionally, running analyses on both the raw/‘uncleaned’ and ‘cleaned’ versions of the dataset helps assess the impact of outliers and can guide decisions on whether to include or exclude them.
Analysing and interpreting results
When it comes to data analysis, Claude.ai has tools that offer specific methods to improve the data analysis process. Although ChatGPT is helpful as an initial step to understanding results, it should be used as a tool for creating literature review ideas and hypothesis generation, not as a fact-based system. The scientific question should remain the anchor of the interpretive process, alongside your understanding of the raw data and output from analytics. Here, it is helpful to toggle between two mindsets: one of a creative scientist, useful for creating avenues of exploration, and one of a critic when assessing the merit of these avenues. In the next article, we will discuss the tools scientists can use to execute the workflow described in Figure 1.
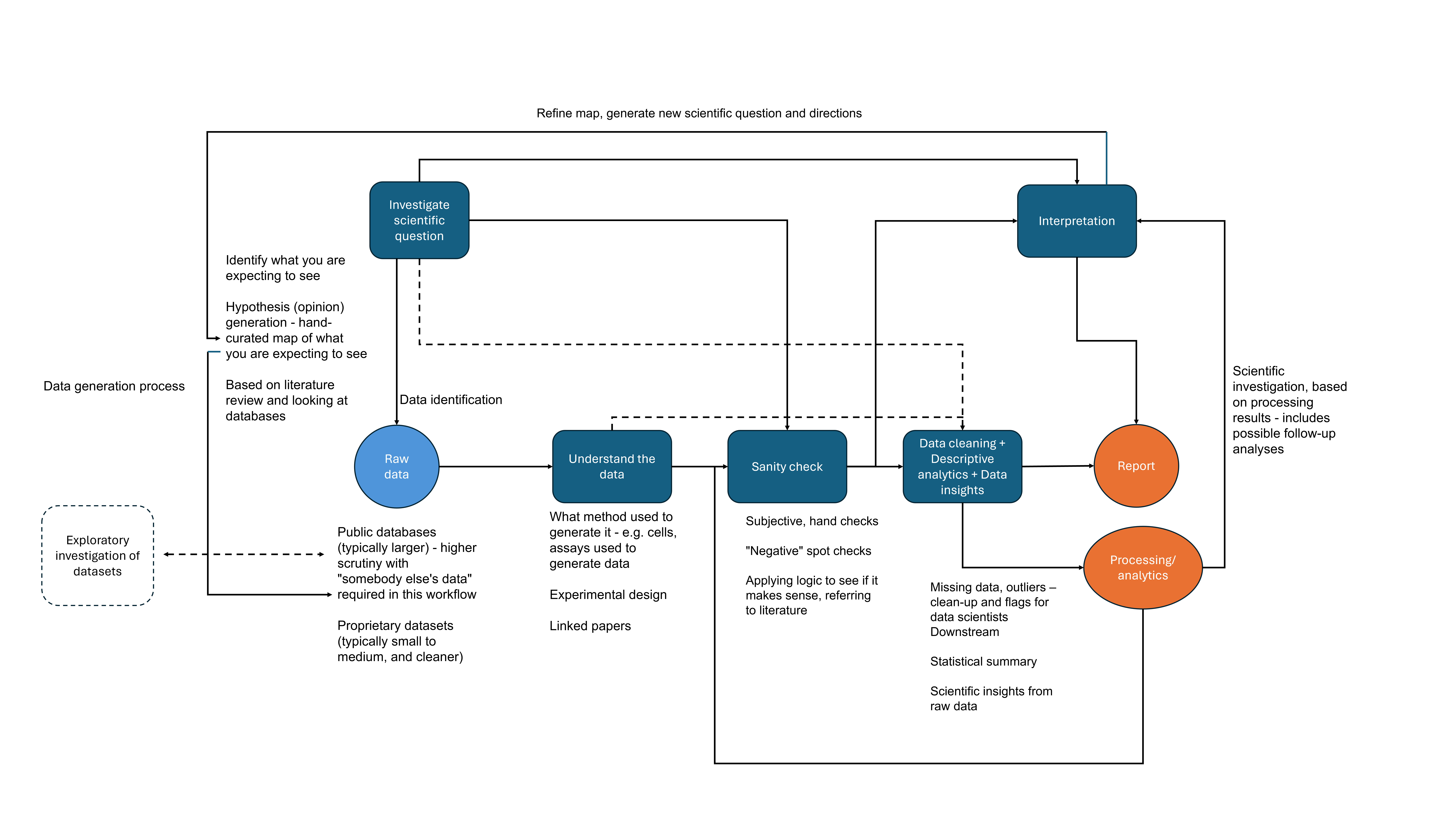
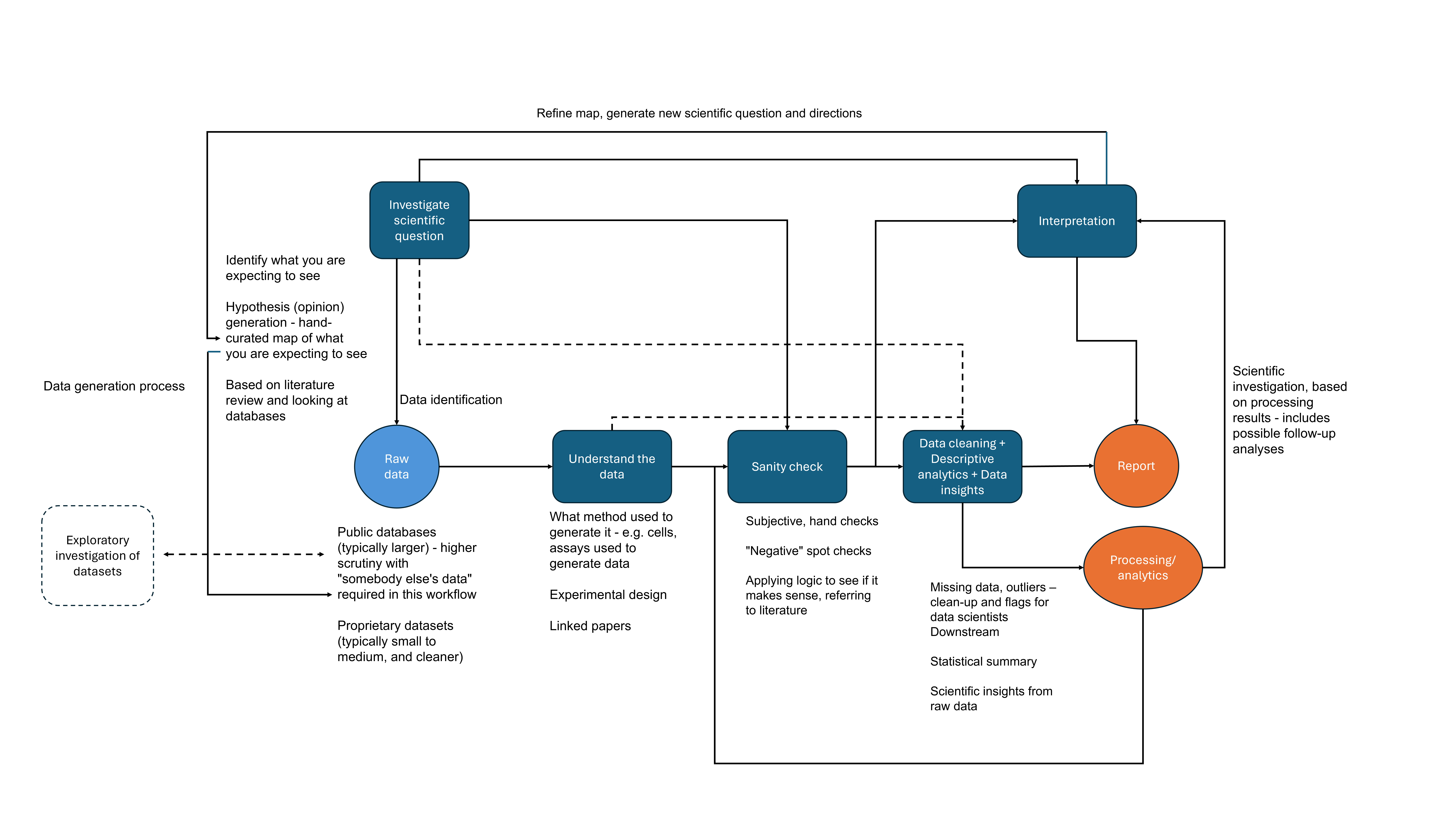
Figure 1: High-level workflow for early drug discovery.
Exploratory investigations and missed opportunities
Often, datasets are generated for a specific research question, but they may contain additional information that could be useful for answering new or unrelated questions. This is particularly true for large public datasets, where the breadth of data available can sometimes be overwhelming. Researchers may miss opportunities to generate new insights simply because they are focused on their initial question and do not have the resources to explore other possibilities.
Additionally, exploratory analyses can be valuable for identifying new biological markers or hypotheses. For instance, a dataset generated to study protein expression in one context might also reveal valuable information about other biological pathways or processes. However, exploratory investigations can be resource-intensive, both in terms of time and computational power. Researchers should therefore balance their focused analysis with the potential for broader discoveries.
For more insights, refer to Part 1 of this series, where we explore foundational concepts for early drug discovery workflows.
Dr Raminderpal Singh
Dr Raminderpal Singh is a recognised visionary in the implementation of AI across technology and science-focused industries. He has over 30 years of global experience leading and advising teams, helping early- to mid-stage companies achieve breakthroughs through the effective use of computational modelling. Raminderpal is currently the Global Head of AI and GenAI Practice at 20/15 Visioneers. He also founded and leads the HitchhikersAI.org open-source community and is Co-founder of the techbio, Incubate Bio.
Raminderpal has extensive experience building businesses in both Europe and the US. As a business executive at IBM Research in New York, Dr Singh led the go-to-market for IBM Watson Genomics Analytics. He was also Vice President and Head of the Microbiome Division at Eagle Genomics Ltd, in Cambridge. Raminderpal earned his PhD in semiconductor modelling in 1997 and has published several papers and two books and has twelve issued patents. In 2003, he was selected by EE Times as one of the top 13 most influential people in the semiconductor industry.
Nina Truter
Nina Truter is a translational scientist with a deep focus on understanding mechanisms of action in drug development and leveraging disparate datasets in biotech. Based in South Africa, she has worked extensively with international biotech companies, specialising in therapeutic development for ageing-related diseases and complex conditions such as glioblastoma and autosomal dominant polycystic kidney disease (ADPKD).
Her recent work includes consulting for UK-based biotech firms and leading initiatives in HitchhikersAI.org to advance the translation of AI and data science into practical biotech solutions such as identifying combination therapy opportunities and enhancing patient selection. In her work, she uses a systems approach to integrate insights from diverse datasets across in vitro, in vivo and human models—to answer critical scientific questions, and translates biological mechanisms into models that are used by advanced analytical methods such as Pearlian causal inference.
Related topics
Analysis, Artificial Intelligence, Drug Discovery, Drug Discovery Processes, Translational Science
Related organisations
20/15 Visioneers, HitchhikersAI