Large language models: now more affordable and reliable than ever
Posted: 24 February 2025 | Dr Raminderal Singh (Hitchhikers AI and 20/15 Visioneers | No comments yet
In this article, Dr Raminderpal Singh explores the transformative impact of the Deepseek R1 open-source large language model on drug discovery. Its potential offers exciting opportunities for both scientists and software developers, marking a significant advancement for the life sciences community.
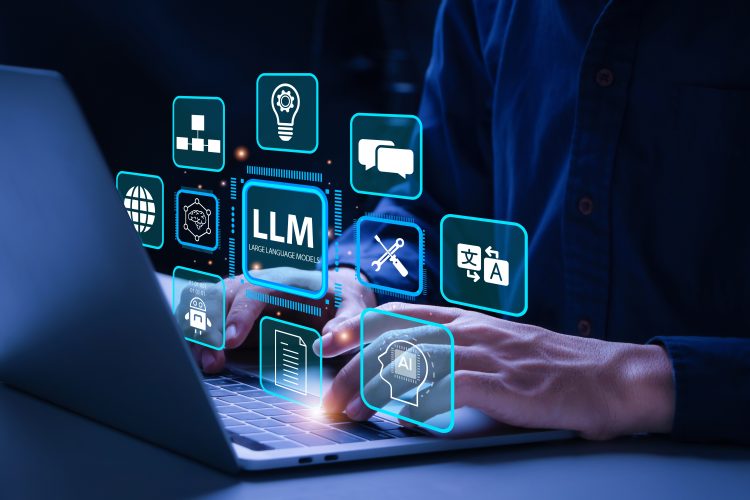
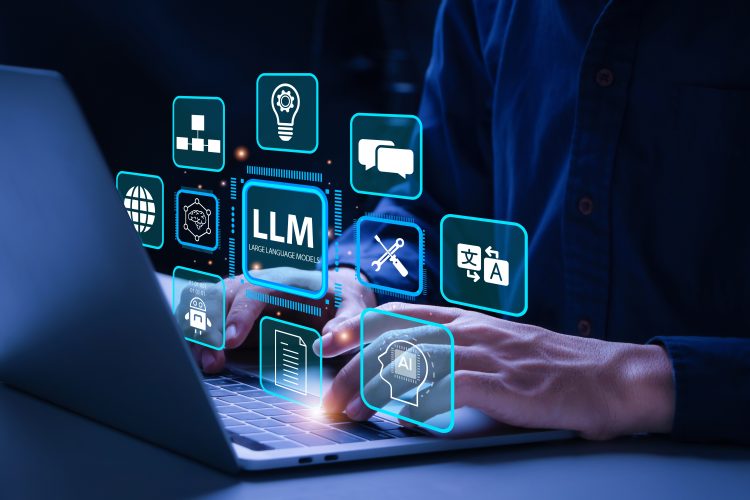
While presenting at the recent SLAS conference in San Diego,1 I found myself at the centre of numerous discussions about the seismic shifts occurring in the AI landscape – ie, Deepseek R12,3 had just gone viral. As someone deeply involved in explaining large language models to life scientists – both in wet labs and drug discovery – I’ve developed a unique perspective on how recent developments are reshaping our industry.
In life sciences, we are largely consumers of AI technologies, rather than developers. We rarely build or fine-tune our own large language models. Two critical factors are important to us: cost and reasoning capabilities – both of which have been significantly transformed by recent developments.
The cost landscape has experienced a remarkable shift. Previously, working with leading AI providers like OpenAI and Anthropic came with significant financial barriers, with monthly costs ranging from $1,000 to $10,000, depending on usage. However, with the recent release of open-source models like DeepSeek R1, we are witnessing the emergence of a true commodity market. Even when accessing these models through cloud services, costs have dropped by an order of magnitude.
To put this in perspective, imagine if someone suddenly introduced a high-quality electric car priced at $10,000 in a market where competitors charge three to four times more: that’s essentially what is happening in the AI space. This cost reduction isn’t just about savings – it’s about democratising access to powerful AI tools across our industry.
The reasoning capabilities of these models are equally impressive. Larger models, with 70 billion parameters, can handle more complex contexts and demonstrate sophisticated reasoning compared to their smaller counterparts of 1-2 billion parameters. This is crucial in life sciences, where we deal with intricate knowledge networks and require a nuanced understanding of complex biological systems.
Large language models will profoundly change life sciences.
What excites me most is how these developments enable us to integrate AI into our workflows more comprehensively. Whether analysing research papers, interpreting experimental results, or automating laboratory processes, we now have access to powerful AI tools to quickly facilitate these tasks at a fraction of the previous cost. This combination of improved reasoning capabilities and affordability opens new possibilities for innovation across the entire life sciences value chain.
The implications are profound for our industry, which fundamentally operates as a knowledge economy. This is not a matter of incremental improvements – this is a paradigm shift that enables us to harness AI’s reasoning skills in ways that were previously cost-prohibitive. As we move forward, I believe we should be bold in exploring how to integrate these capabilities into our offerings and workflows, regardless of where we sit in the supply chain.
For the life sciences industry, this democratisation of AI technology is not just an opportunity, but a call to action. The tools are now accessible; it’s up to us to leverage them effectively.
About the author
Raminderpal has extensive experience building businesses in both Europe and the US. As a business executive at IBM Research in New York, Dr Singh led the go-to-market for IBM Watson Genomics Analytics. He was also Vice President and Head of the Microbiome Division at Eagle Genomics Ltd in Cambridge. Raminderpal earned his PhD in semiconductor modelling in 1997. He has published several papers and two books and has twelve issued patents. In 2003, he was selected by EE Times as one of the top 13 most influential people in the semiconductor industry.
References
SLAS2025 International Conference & Exhibition [Internet]. Society for Laboratory Automation and Screening. 2025 [cited 2025 Feb 5]. Available from: https://www.slas.org/events-calendar/slas2025-international-conference-exhibition/
2. DeepSeek-AI, Guo D, Yang D, Zhang H, et al. DeepSeek-R1: Incentivizing Reasoning Capability in LLMs via Reinforcement Learning [Internet]. arXiv.org. 2025. Available from: https://arxiv.org/abs/2501.12948
3. CNBC Television. China’s DeepSeek triggers global tech sell-off [Internet]. YouTube. 2025 [cited 2025 Feb 5]. Available from: https://www.youtube.com/watch?v=V-Fla5hxMRg
Related topics
Artificial Intelligence, Computational techniques, Lab Automation, Machine learning
Related organisations
Anthropic, Hitchhikers AI and 20/15 Visioneers, OpenAI, SLAS (Society for Laboratory Automation and Screening)