Using knowledge graphs in drug discovery (Part 1): how they link to large language models
Posted: 21 April 2025 | Andreas Kollegger (Head of GenAI innovation at Neo4), Dr Raminderpal Singh (Hitchhikers AI and 20/15 Visioneers) | No comments yet
In this first interview of a two-part series, Andreas Kolleger explores the convergence of knowledge graphs and large language models. As the head of GenAI innovation at Neo4j, Andreas brings a unique cross-industry perspective on how these technologies can enhance life sciences workflows.
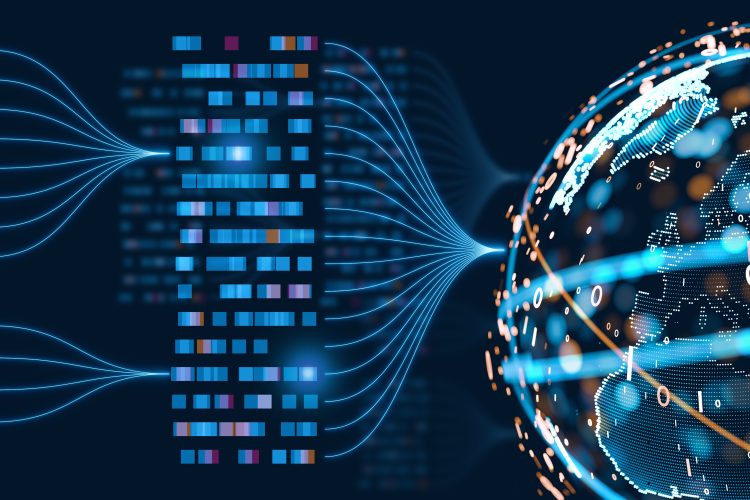
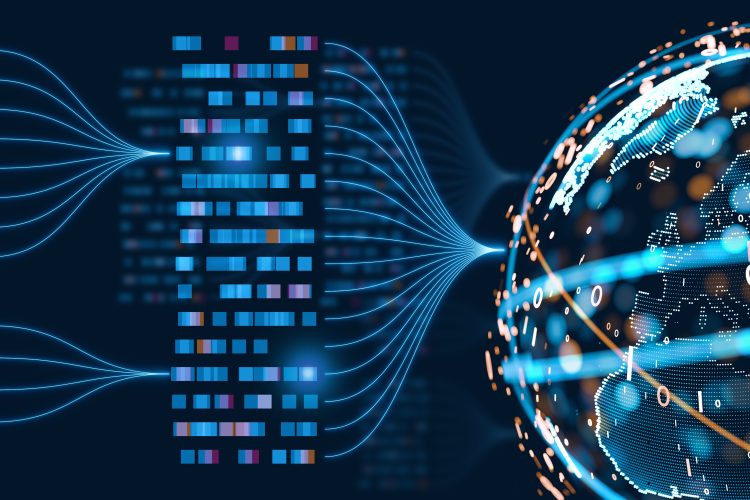
Knowledge graphs, long established in data management and symbolic reasoning, are experiencing a renaissance in the era of generative AI, particularly in drug discovery. These structured data representations, which predate the current AI boom, have evolved from their origins as tools for data interchange and symbolic reasoning into sophisticated platforms for knowledge management and discovery.
What are knowledge graphs?
At their core, knowledge graphs serve as interconnected networks of information where relationships between entities are explicitly defined and meaningful. In drug discovery, these entities might include compounds, proteins, genes, diseases and clinical outcomes – all connected through verified scientific relationships. This structured approach to data organisation offers two key advantages:
- Systematic reasoning – enables understanding of complex relationships between data points
- Standardised framework – allows seamless data interchange across different systems and organisations.
The fundamental strength of knowledge graphs lies in their ability to represent relationships between data points in a way that mirrors natural language structure. As Andreas Kollegger explains, “You can read the graph… almost read a sentence along it.” This natural alignment between graph structures and language has become particularly valuable with the rise of large language models (LLMs), creating what Kollegger describes as “a very nice property that maps nicely to natural language.”
Moving beyond traditional NLP
The integration of knowledge graphs with large language models represents a significant evolution from their traditional use with natural language processing (NLP). In the earlier NLP era, knowledge graphs primarily served as structured repositories that NLP tools could query and update. The current integration with LLMs is fundamentally different and more powerful. While NLP tools previously handled the basic processing of text and relationships, now LLMs serve as sophisticated processors with enhanced reasoning capabilities, able to:
- understand context
- identify connections between complex data points
- generate insights in ways that resemble human cognitive processes.
This evolution has enabled a more nuanced approach to handling unstructured data – from research papers and documents to images and other scientific content. When working with unstructured data, LLMs can now perform what Kollegger terms “distilling the essential facts.” For example, when analysing scientific literature, the system can identify key entities and their relationships, effectively creating a structured knowledge representation from unstructured text.
When presented with a question, an LLM can break down complex queries into component parts, identifying what information it needs to discover.
One of the key innovations in this space is the way LLMs interact with knowledge graphs. When presented with a question, an LLM can break down complex queries into component parts, identifying what information it needs to discover. The system then uses the knowledge graph to find relevant data points and, crucially, the relationships between them. This process creates what Kollegger describes as a path between points of interest, essentially building a specialised mini knowledge graph specific to the query at hand. This capability is particularly powerful because it allows the system to answer questions that might require information from multiple sources or complex chains of reasoning.
Why it matters for drug discovery
The combination of knowledge graphs and LLMs has proven particularly valuable in drug discovery, where relationships between compounds, proteins, pathways and clinical outcomes are complex and multifaceted. The knowledge graph provides a curated, validated foundation of scientific relationships, while the LLM offers the flexibility to explore and reason about these relationships in novel ways. This hybrid approach addresses one of the fundamental challenges in drug discovery: the need to both maintain scientific rigor and enable creative exploration of new possibilities.
Furthermore, this integration helps address a critical concern in scientific research – the verification and validation of results. While LLMs can generate insights rapidly, the knowledge graph serves as a source of validated, structured information that researchers can audit and verify. As Kollegger notes, this creates a form of “pre-computed” knowledge, where the hard work of validating scientific relationships has already been done through research and academic studies.
About the authors
Dr Raminderpal Singh is a recognised visionary in the implementation of AI across technology and science-focused industries. He has over 30 years of global experience leading and advising teams, helping early to mid-stage companies achieve breakthroughs through the effective use of computational modelling.
Raminderpal is currently Global Head of AI and GenAI Practice at 20/15 Visioneers and leads the HitchhikersAI.org open-source community. He is also a co-founder of Incubate Bio – a techbio providing a service to life sciences companies who are looking to accelerate their research and lower their wet lab costs through in silico modelling.
Raminderpal has extensive experience building businesses in both Europe and the US. As a business executive at IBM Research in New York, Dr Singh led the go-to-market for IBM Watson Genomics Analytics. He was also Vice President and Head of the Microbiome Division at Eagle Genomics Ltd, in Cambridge. Raminderpal earned his PhD in semiconductor modelling in 1997. He has published several papers and two books and has twelve issued patents. In 2003, he was selected by EE Times as one of the top 13 most influential people in the semiconductor industry.
For more: http://raminderpalsingh.com; http://20visioneers15.com; http://hitchhikersAI.org
Andreas is a technological humanist. He began at NASA, designing systems from scratch to support scientific missions. In Zambia, he developed medical informatics systems to apply technology for social good. Now at Neo4j, he’s focused on democratising graph databases to validate and extend our understanding of how the world works.
Related topics
Analysis, Artificial Intelligence, Big Data, Computational techniques, Drug Discovery, Drug Discovery Processes, High-Throughput Screening (HTS), Informatics, Machine learning, Screening, Translational Science
Related organisations
Hitchhikers AI and 20/15 Visioneers, Neo4j