Drug resistance mechanisms and target selection in disease treatment strategies
Posted: 10 May 2014 | Bhupinder Bhullar (Novartis Institute for Biomedical Research) | No comments yet
In November 2013, the US Food and Drug Administration (FDA) allowed for the first time the marketing of high-throughput DNA sequencing technology for diagnostic testing. It was a breakthrough year for Next Generation Sequencing (NGS)…
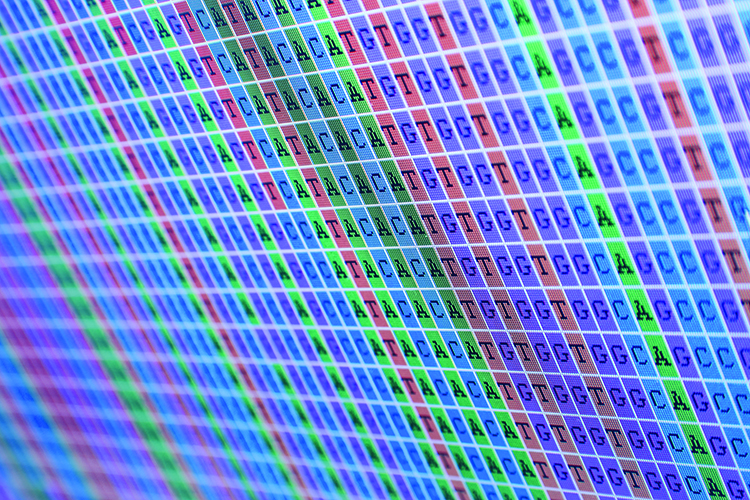
The approval by the regulatory authority will help to realise the promise of precision medicine – enabling the use of the patient’s genetic information to detect unique disease driving DNA mutations and determine (in real time) how the patient responds to treatment.
Unlike conventional DNA sequencing-based diagnostics, NGS will enable a faster and more sensitive detection of nucleotide sequences in the clinic, offering potential life-saving applications in cases where the identification of the protein target’s mutational status is critical to guide the treatment.
Selecting the best targets is a key challenge for drug discovery in general, and an exasperating impediment in therapeutic areas where drug resistance is a likely occurrence. Cancer cells and infectious pathogens (parasites, bacterial and viral pathogens) are notorious for acquiring resistance to therapeutic regimens.
We can illustrate this difficult problem using the example of Human Immunodeficiency Virus (HIV). Individuals infected with HIV can produce up to 10 billion viral particles daily. Because of the rapid turnover, it is estimated that approximately one nucleotide mutation is made per new HIV RNA1. The single base changes (or point mutations) can result in amino acid substitutions (called missense mutations) or protein truncations (non-sense mutations). Mutations can also lead to base insertions and deletions (indels) which effectively alter the reading frame and result in loss of function downstream of the indel.
Multiple studies have demonstrated minor nucleotide variants in HIV viral population are clinically relevant and can lead to therapy failure2. Thus, applying evolutionary principles with the appropriate selection pressure (i.e. drug treatment), infected patients can experience outgrowth of scarce virus particles that harbour nucleotide variation which confer growth advantage under the selection pressure.
In the clinical environment, HIV proliferation in response to antiretroviral drug therapy can be measured by quantitative PCR, an assay routinely used in hospital settings. When antiretroviral drugs are working optimally, viral replication is suppressed and viral load in the patient is below the assay’s detectable limits. In a fraction of the patients, however, the viral load is suppressed only for a period of time, before the virus levels rebound due to acquired resistance to the treatment. Two major questions are clinically relevant: 1) what medications is the patient taking that the rebound virus have become resistant to?; and 2) which drugs could still be effective and used as second line treatment? For HIV treatment, the answers to both of these questions hinge on understanding the molecular mechanism of action of the drugs and elucidating the variation that might have occurred in the target space.
It is important to understand the biological basis of drug resistance that results from acquired mutations. Generally, resistance mechanisms fall into two major categories – either the drug target is unchanged or the target has attained modifications. The first category includes alterations to cellular mechanisms that regulate drug import, export, or metabolism. For example, elevated drug export is the mechanism that is commonly referred to multi-drug resistance (MDR) and can arise from expression level changes of membrane solute pumps. The second category encompasses altering the kinetics of interactions between the drug and target, for example, through point mutations that alter the drug binding site or the level of protein expression that allow the enzyme activity to prevail over the pharmacokinetic properties of the compound. The acquired mutations in the target protein must allow it to continue to undertake its normal function, otherwise the pathogen’s and/or cell’s viability will be compromised and the mutation will not propagate or be detected in the patient. For example, there are 14 important amino acids in the HIV protease, which when mutated to a specific alternate amino acid, can confer resistance to eight of the clinically used protease inhibitors (see Figure 1). These mutations create structural variations that alter the binding of the drug to the protease, but do not impede its function which is critical to the viral replication cycle.
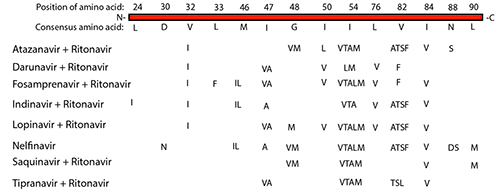
Figure 1: Amino acid mutation in HIV Protease which confer strong resistance to the major protease inhibitor drugs.
While many of the point mutations in the protease can confer resistance to multiple protease inhibitors, some mutations uniquely confer resistance to one protease inhibitor and not the other. For example, I50L variant confers robust resistance to Atazanavir, whereas I50V variant (same location but different amino acid) confers a robust reduced viral response to Darunavir, Forsamprenavir, and Lopinavir. Neither I50L nor I50V amino acid changes confer resistance to the protease inhibitors Indinavir, Nelfinavir, Saquinavir, or Tipranavir, which may be used as preferred treatments3. Thus, knowing the mutation space in the HIV protease gene, and in general the HIV genome, can help guide the best clinical treatment, especially when resistance arises.
HIV is the one of the fastest mutating genomes and develops a rapid resistance to all the available single therapies. The treatment that diminishes the viral load to below assay detection utilises a combination of five drugs that inhibit the: i) nucleoside reverse transcriptase; ii) non-nucleoside reverse transcription proteins; iii) a protease; iv) entry/fusion proteins; and v) integrase required for insertion of the viral DNA into the genome of the host CD4+ cell. This combination therapy leverages the improbability of multiple mutations events acquired in a single viral particle’s RNA genome that could overcome the inhibitory effects of five drugs targeting these required functions by the virus4. This cocktail is effective at halting the further progression of the viral particles, however treated HIV positive individuals still harbor active virus and can pass the virus to other individuals.
The nucleotide variations present in individuals infected with HIV have major implications on drug resistance, pathogenesis, and vaccine development; however, the diagnosis of the resistance ‘potential’ remains a challenge. NGS technology was generally thought to have high error rates with aberrant base calling which impeded the application of this technology in the clinic – where therapeutic decision making require very accurate diagnostic detection. In November 2013, the FDA approved the use of the Illumina MiSeq platform for developing clinical protocols for detection of nucleotide variation in clinical settings. The MiSeq platform is very suitable for focused DNA variation detection (e.g. survey a few human genes, sequence small genomes like HIV), and coupled with its 15 million sequence reads, DNA sequence variations can be ascertained with high quality and confidence for small sequencing projects (e.g. de novo sequencing of viral and bacterial genomes, targeted sequencing of gene sets). For example, among the approved protocols by the FDA for the MiSeq platform is the assessment of the mutation status of the CFTR receptor for Cystic Fibrosis detection, which can acquire one of 139 known disease causing mutations. A protocol for clinical HIV genotyping for drug resistance detection using NGS platforms remains to be approved for clinical applications5.
One clinical application where NGS will experience growth is detection of the mutation status of cancer driver genes in tumour biopsies – enabling an oncologist to use the results of the sequence scan to choose the drugs that are most likely to work. Over the last decade, large cancer sequencing projects, led by the International Cancer Genome Consortium (ICGC) and the Cancer Genome Atlas (TCGA), have resulted in a catalog of genes, which, when mutated, can promote tumourigenesis.
The current data release from ICGC (release #15, February 2014) comprises data from 10,067 cancer samples from 50 different tumour types. In a 2013 science journal article, Bert Vogelstein et al. summarised the results which were startling: approximately 140 mutated genes were identified as drivers (or promoters) of tumourigenesis6. These 140 genes fall into 12 signalling pathways. In any particular tumour type, only two to eight of these driver mutations were discovered6. This suggests that for a given patient biopsy, a survey of the sequence status of a handful of genes that are relevant drivers for that tumour type would be sufficient to determine the most effective therapeutic modality for that patient.
Among the tumour samples surveyed, the total number of detected SNP/indel variants that have a deleterious effect on the protein structure and function, range from approximately two to 200 per tumour – depending on the age of the patient and tissue of origin6. For instance, pediatric cancers have fewer acquired mutations compared to an adult tumour of the same tissue origin because of the fewer number of cellular divisions that have occurred. Lung cells exposed to DNA damaging agents like cigarette smoke have picked up more mutations, than a lung from a non-smoker. This difference in mutation numbers suggests that mutations do accumulate over time and with exposure to carcinogens. The majority of these mutations are ‘passenger’ mutations because they do not play a significant role in the growth and promotion of the emerging cell mass.
It is not clear what role a driver and/or passenger mutations may play in combination, but the model that cancer is simply an ‘evolving mutation machine’ is backed up by the wealth of sequencing data that we have amassed thus far7. In a survey of 30 cancer genome sequences cataloged by TCGA, researchers uncovered 21 mutation signatures (out of a possible 192 types of mutations) that were prevalent in cancer genomes – which can be attributed to specific DNA repair defects (e.g. microsatellite instability) that arise in cancer cells7. These 21 signatures give researchers an idea of which DNA repair mechanisms commonly fail to produce the total genomic heterogeneity in a tumour.
In the tumour mass lies enough genetic diversity that, at the molecular level, should be viewed as an amalgamation of different growths. Some variability might be at a low occurrence such that it might not be detected by the current methods of conducting biopsies and sequencing the isolated genomic material. Following a successful treatment regimen to reduce the initial tumour mass, rare and undetected variability can facilitate resistance to the drug therapy regimen resulting in the relapse outgrowth.
With this information at hand, and the catalogue of approved drug molecules that inhibit a selection of the activated targets and molecular signaling pathways, reducing cancer morbidity appears within grasp. After the initial tumour biopsies are sequenced and the mutations identified, the first drug regimen is selected to target the combination of driver mutations detected in the tumour’s mutagenic space. This will decrease the initial tumour burden. When the patient exhibits the first relapse, the tumour biopsy is again taken to determine the driver mutation(s). The second therapy regimen is changed to include inhibitors to the new target(s) or signalling pathway(s). Doggedly pursuing the tumour’s mutation space with detection and treatment cycles will bring the tumour to a possible dead-end – where it can no longer acquire new mutations that will give it a growth advantage over the applied drug therapy.
The challenges for implementing this model, though, are many. This model for precision medicine presumes that detection of the mutation signal is possible using comprehensive biopsy sampling and NGS platforms. There have been significant improvement in NGS technology and applications development, however, the technical challenges of sampling and sequencing remain. There is no biopsy method to dynamically sample a tumour to ensure that the presence of rare mutations can be evaluated with a high degree of confidence. In addition, our current sequencing and mutation calling algorithms cannot differentiate a very low frequency variant (i.e. one read in 50x coverage) from a sequencing error. For this reason, the current challenge for clinical centers is to keep all the raw data generated from sequencing a biopsy such that if future data sheds light on new mutations and mechanisms, it can be identified in the original biopsy, verified and acted upon.
Accurate cataloguing of the cancer driver mutations in tumour types still has a long way to go. A recent publication analysing 4,742 samples from 21 different cancers, detected many of the genes that studies mentioned above found. However, using an analysis method to look across different cancer types, an additional 33 new cancer driver genes were found8. The research team speculates that sequencing 100,000 tumour samples representing different subtypes would ensure a more thorough sampling of cancer driver mutations8.
The majority of cancer genome sequencing projects only assess the exome (the protein coding regions of approximately ~23,000 protein coding genes). Additional components that need to be analysed include epigenetic changes and the status of non-protein coding genes. Epigenetic regulation has a pivotal role in controlling proper expression levels of genes. Aberrant methylation, for example, can result in up-regulation of growth promoting proteins or down-regulation of tumour suppressor proteins. The annotation of the number of non-coding RNA (ncRNA) genes is growing and maybe estimated at more than 40,000 such genes in the human genome, some with a clear role in human disease9. For example, the micro-RNA miR21 has a role in cancer progression. The targets of miR-21 are primarily genes with tumour suppressor and DNA repair functions, and miR-21 upregulation is associated with many types of cancers which makes it an attractive target for therapeutic intervention10.
The net effect of these activities to annotate the mutational landscape of the cancer diseasome will identify many new target opportunities. The state of our drug resistance detection and disease management strategies will get better as the thoroughness and accuracy of these large databases increases. As databases continue to expand and integrate the molecular picture of the underlying disease biology, we will further illuminate the underlying factors driving many diseases, especially drug resistant cancers and infectious diseases, and usher in the next generation of revolutionary diagnostic and therapeutic technologies to enable target guided treatment modalities.
Biography
BHUPINDER BHULLAR is Laboratory Director at Novartis Institute for Biomedical Research.
References
- Hirsch MS, Conway B, et al., (1998) Antiretroviral drug resistance testing in adults with HIV infection: implications for clinical management, International AIDS Society–USA Panel. JAMA, 279 (24), 1984-91
- Johnson JA, Li JF, et al., (2008) Minority HIV-1 drug resistance mutations are present in antiretroviral treatment-naive populations and associate with reduced treatment efficacy, PLoS Med 5: e158. doi: 10.1371/journal.pmed.0050158
- Stanford University database of mutations in HIV (accessed January 2014) http://hivdb.stanford.edu/DR/PIResiNote.html
- Cane PA, (2009) New developments in HIV drug resistance, J Antimicrob Chemoth, 64 (Suppl.1): 37–40.
- Di Giallonardo F, Zagordi O, et al., (2013) Next-Generation Sequencing of HIV-1 RNA Genomes: Determination of Error Rates and Minimizing Artificial Recombination, PLoS One, DOI: 10.1371/journal.pone.0074249
- Vogelstein B, Papadopoulos N, (2103) Cancer Genome Landscapes, Science, 339, 1546-1558
- Alexandrov, L.B., Nik-Zainal, S., et al. (2013) Signatures of mutational processes in human cancer, Nature, 500, 415-421
- Lawrence MS, Stojanov P, et al., (2014) Discovery and saturation analysis of cancer genes across 21 tumour types, Nature, 505, 495-501
- Aceview database: integrative annotation of cDNA-supported genes in human (accessed January 2014) http://www.ncbi.nlm.nih.gov/IEB/Research/Acembly
- Sicard F, Gayral M, et al., (2013) Targeting miR-21 for the Therapy of Pancreatic Cancer, Nature, 21, 986-994.
Related topics
DNA, Genomics, Next-Generation Sequencing (NGS), Oncology, Screening, Therapeutics