PAINS management: an open source model to eliminate nuisance compounds
Posted: 4 December 2018 | Stephen J. Capuzzi and David C. Morris (Eshelman School of Pharmacy - University of North Carolina Chapel Hill) | No comments yet
High-throughput screening (HTS) technologies have enabled the routine testing of millions of compounds towards the identification of novel ‘hit’ molecules for therapeutic targets. Oftentimes in this drug discovery process, however, compounds that show promising activity in primary screens show no activity during subsequent hit qualification or progression efforts.
Alarmingly, these compounds frequently masquerade as false hits in multiple assays against multiple targets, constituting a major waste of resources during drug discovery campaigns.
The identification and elimination of these nuisance compounds – which, in recent years, have also been called PAINS, or Pan-Assay INterference compoundS – is thus of great interest and importance to the drug discovery community.
At the same time, open pre-competitive science has been gaining momentum in the drug discovery community. This new paradigm has resulted in the sharing of many useful tool compounds in and between pharmaceutical companies and academic institutions, in order to effectively explore disease biology.
In this review, we propose that a similar model should be adopted for the identification and segregation of nuisance compounds. We argue that, as with higher-quality compounds, knowledge of these lower-quality and non-progressed compounds should be openly shared. Public sharing of these data would focus efforts away from nuisance compounds and towards compounds that exhibit criteria of progressable molecules. We further propose that these nuisance compounds be shared through several mechanisms in both a fully-open and ‘semi’-open manner. We also illustrate how information about these nuisance compounds can be leveraged to assess the behaviour of new and untested compounds. We envision that this model will become the gold-standard for the identification and elimination of nuisance compounds.
Introduction
“It is a very sad thing that nowadays there is so little useless information” – Oscar Wilde
Open pre-competitive science has been gaining momentum as a productive method of research among drug discovery scientists.1–3 This new paradigm has resulted in the sharing of many useful chemical tools among and between pharmaceutical companies, as well as academic institutions, in order to foster innovation and progress these compounds in a pre-competitive manner.4 Examples of this open science model can be found in the Published Kinase Inhibitor Set (PKIS) constructed by a consortium of academic and industrial partners,5 and by Boehringer Ingelheim’s opnMe platform through which select tool compounds are openly and freely shared with the scientific community.
At the same time, there are far more low-quality compounds in high-throughput (HTS) collections that will likely never be progressed to clinical candidacy since many of them elicit their ‘activity’ through nonspecific effects, such as chemical reactivity, assay interference, and colloidal aggregation.6–8 Moreover, these compounds frequently masquerade as false hits in multiple assays against multiple targets – an observation that has given rise to the term PAINS, or Pan-Assay INterference compoundS. These nuisance compounds[1] constitute a major waste of resources during drug discovery campaigns.9 Unfortunately, the actual structures of these compounds are seldom reported in the public domain, constituting a wealth of untapped ‘useless’ information about non-progressed/non-progressable compounds. Instead, structural alerts/filters are used to identify potential nuisance compounds computationally, though it is well-known that these tools are limited in efficacy and utility.10,11
We propose that these low-quality, problematic, and unprogressable nuisance compounds should be openly shared to reduce waste and avoid repeat frustrations. We will demonstrate that these nuisance compounds can be shared through several mechanisms in both a fully-open and ‘semi’-open manner. We will also illustrate how these historical nuisance compounds can be leveraged to assess the nuisance behaviour of new and untested compounds. We envision that an open model will become the gold-standard for the identification and elimination of nuisance compounds. Through this model, information pertaining to less valuable molecules will be transformed into actionable knowledge.
How much of a nuisance are Nuisance Compounds?
“In God’s garden, even the weeds are beautiful. In my garden, I’ve only got weeds. I think they’re a nuisance.” – Anthony T. Hincks
Although we do not have an exact number of nuisance compounds in every HTS collection for every pharmaceutical company or academic institution, publicly available data on this issue from industry partners can provide insight into the scope of the problem. In 2018, Chakravorty et al published an analysis of nuisance compounds in the GlaxoSmithKline (GSK) high-throughput screening collection that were flagged by any one of the 410 structural alerts used in their hit triage.12 The authors computed an Inhibitory Frequency Index (IFI) for those compounds that had been tested in at least 50 HTS assays at 10μM. The IFI was defined as the proportion of non-kinase assays in which a compound shows inhibition ≥ 50 percent, calculated according to Equation 1:
According to parameters set by the authors, compounds with IFI > 2 were considered noisy. By their own definitions, there were 502,895 noisy compounds that represented potential nuisance compounds, or ~22 percent of all compounds in the analysed collection.12 The goal of this study was to analyse the efficacy of the 410 structural alerts used in hit triage to flag nuisance compounds. It should be noted, therefore, that the actual number of nuisance compound in the overall collection is likely to be higher considering the noisy compounds (IFI > 2) that were not flagged by these alerts.
It is clear that nuisance compounds pollute HTS collections. Indeed, if the same analysis as above is performed across several companies/institutions, the number is likely to exceed several million nuisance compounds. Presently, however, knowledge of these compounds and their identities are not openly shared; instead, a known bad actor from one collection will continue to masquerade in another, perpetrating this avoidable cycle of futility and frustration.9 Echoing the sentiment of Anthony T. Hincks, these nuisance compounds, which insidiously weave among better compounds like weeds, could be cultivated and used to deracinate new nuisance compounds before they sprout within the collection.
The rogues’ gallery of nuisance compounds – a database
In 1855, Allan Pinkerton, founder of the Pinkerton National Detective Agency, established the first rogues’ gallery – a collection of mug shots and names of criminals, replete with methods of operation, reported hideouts, and known associates.13 The purpose of this rogues’ gallery was to help law enforcement and witnesses alike identify and locate known criminals. Indeed, the FBI’s Ten Most Wanted List serves as a modern-day rogues’ gallery.
This paradigm could be adopted to rid screening collections of known nuisance compounds from various sources and help flag additional compounds for scrutiny. This paradigm has, in fact, been employed in the Aggregator Advisor tool developed by the Shoichet group at UCSF.14 This web-based application attempts to identify potential colloidal aggregators by comparing the structural similarity of queried compounds to ~12,500 known aggregators collected from 18 different screening campaigns.14 This same methodology is also implemented in the ZINC15 database.15
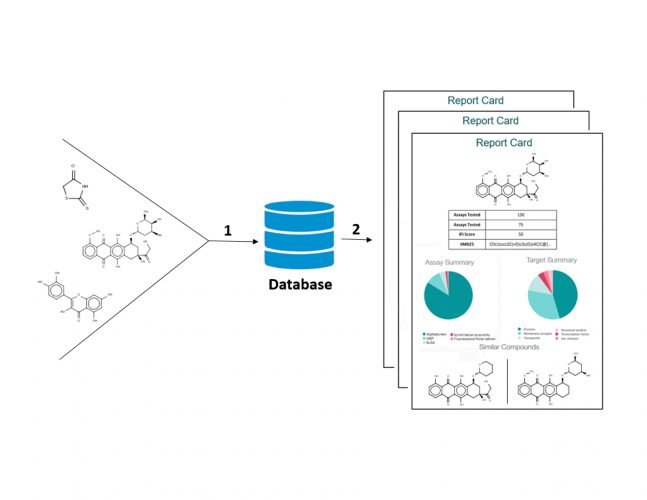
Figure 1. Database of Nuisance Compounds. (1) Nuisance compounds, with underlying chemogenomics data, are uploaded to the database. (2) The database can be accessed, and a compound report card can be obtained for all compounds. This compound report would contain total number of assays tested, the number of assays in which the compound is active, the IFI score and SMILES of the compound. Additionally, a summary of the assay types and target classes against which the compound was tested/active would be reported. Structurally similar compounds to the query compound would be linked.
We envision that a similar database and web application could be developed for all such nuisance compounds, regardless of etiology. Using a harmonised and systematic definition of nuisance, such as the one developed by Chakravorty et al,12 rogue compounds, as well as their purported nuisance mechanisms (methods of operation) and susceptible assay platforms and targets (reported hideouts), could be uploaded to the database, constituting a virtual rogues’ gallery. This database would, thus, contain a large collection of actual ‘identities’ of nuisance compounds, in terms of chemical structure, as well as provide the experimental data used to indict these compounds. A schema for this database can be found in Figure 1.
The database could then be combed through for known offenders, like mugshots in a real rogues’ gallery, and structural similarity searches could be performed to find additional ‘suspects’ (known associates) (Figure 2). We also imagine that specific chemotypes/scaffolds could be queried in order to return a profile of nuisance compounds that fit this description. Through this open-access database, researchers could triage and/or flag screening libraries using full chemical structures of known nuisance compounds, rather than simple substructural alerts, and avoid susceptible assay platforms and targets that may fall victim to these compounds.
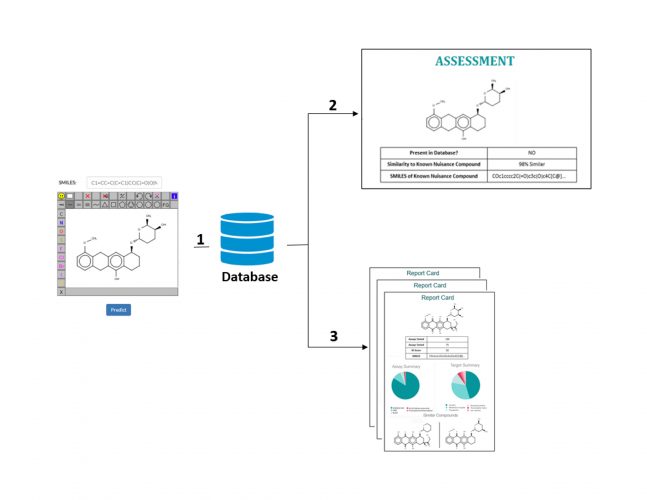
Figure 2. Assessment of Nuisance Potential. (1) A compound of interest could be sketched or its SMILES uploaded. This compound would be compared against all compounds present in the database. (2) An assessment of the compound would be returned, such as the presence/absence of this compound in database and its maximum structural similarity to a compound in the database. (3) All compounds in the database above a designated similarity threshold would be returned and the nuisance profile of these compounds could be assessed.
We also recognise that such a fully-open model may have several limitations and barriers to adoption. First, industry partners may be reticent to reveal chemistry and therapeutic targets of interest for fear of ceding competitive advantage. We recommend, therefore, that industry partners ‘opt-in’ and only reveal chemical structures and data for those compounds they deem of least value, so to speak. For instance, a form of this paradigm can be found in Boehringer Ingelheim’s opnMe platform, wherein the chemical structures, biological targets and activities of ~30 high-quality tool compounds (though not all such compounds) are made publicly accessible. We speculate that if these fears of divulgence can be allayed for useful compounds, a similar system may be adopted for useless compounds.
Second, we recognise the considerable effort required to implement, maintain, and manage this database. Moreover, we recognise that stewardship of the database – and, thus, the data that underlies it – may be a barrier to its construction. Again, however, examples of this paradigm functioning well within the public domain for useful tool compounds can be found in both the Published Kinase Inhibitor Set (PKIS)/Comprehensive Kinase Chemogenomic set (KCGS)4,5 and the Chemical Probes Portal – a database of chemical probes.16 Each of these open-access endeavours were achieved through close collaboration of a consortium of academic and industrial partners. We suspect that if a similar ethos is adopted for the elimination of nuisance compounds, the successful implementation of this database could be well within reach.
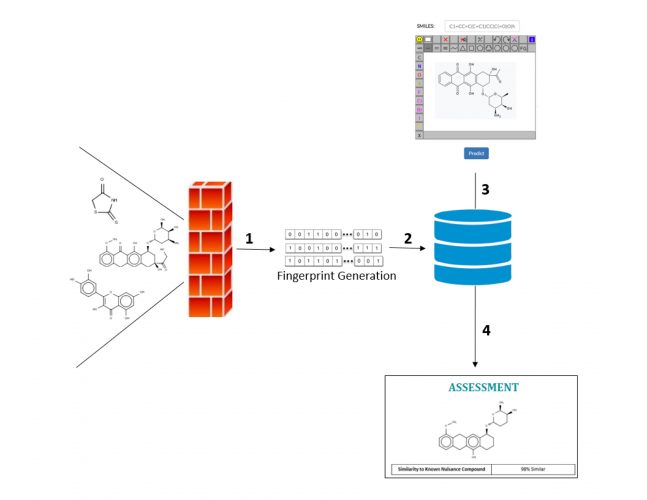
Figure 3. A Semi-Open Model of Nuisance Compounds. (1) Nuisance compounds are uploaded to the database, but are not publicly accessible. (2) Fingerprints are generated for the uploaded compounds and stored in a de-identified database. (3) The fingerprint of a compound of interest is generated and then queried against fingerprints present in the database. (4) An assessment of the compound would be returned based on its maximum structural similarity to a compound in the database.
If, however, this fully open-access database is not embraced, a ‘semi’-open model could be deployed to combat the challenge of problem compounds in screening collections. A simple solution would be for participants to merely annotate their compounds as “nuisance compounds” as per the previously described parameters, calculate chemical descriptors/fingerprints for these compounds, and then upload this information to the database. These reported nuisance compounds could thus be stored in the database in a pseudo-classified manner.17 A schema for this model can be found in Figure 3.
Final remarks
For any single HTS, teams typically derive a list of compounds that satisfy pre-determined criteria for progression to lead optimisation. Following multiple HTS campaigns, it is possible to assess the quality of the compound collection in terms of the hits identified, the molecules pursued and those that were not progressed for one reason or another. As chemists pursue and, in some instances, discard chemotypes that prove to be more problematic than others, the cumulative data summary of which chemistry did not work/was not progressed represents another repository of useful information. Extending this scenario across multiple companies/institutions effectively creates an industry-wide knowledgebase of compound fates. We propose that a mechanism to increase awareness of non-progressed compounds also represents valuable information for teams mulling decisions to progress – or not – specific compounds from HTS campaigns. The industry has a tremendous opportunity to save resources and costs by not pursuing molecules found to be wanting in terms of real target engagement. The open sharing of information about the ‘least wanted’ would be a significant step towards this goal.
Acknowledgements
The authors gratefully thank the financial support from NIH (grant 1R01GM114015) and UNC ROI (IPF ID 17-4673) and from the Eshelman Institute for Innovation. We also thank Dr Johnathan Baell for his valuable suggestions. SJC thanks MFM and JMC for institutional support.
Biographies
Dave Morris is a former VP of Biological Sciences in R&D at GSK where he led teams that built reagents and assays to enable hit discovery, lead optimisation, mechanism of action and product differentiation studies. Dave has maintained a long-standing interest in exploring technologies and scientific approaches that facilitate the progression of drug-like molecules. He is currently a Research Director in the UNC Catalyst for Rare Diseases at UNC, Chapel Hill, North Carolina.
Stephen J Capuzzi received his PhD in Chemical Biology and Medicinal Chemistry at the University of North Carolina – Chapel Hill, working under the direction of Professor Alexander Tropsha. He is currently a Post-Doctoral Fellow at the UNC Catalyst for Rare Diseases.
References
- Masum H, Rao A, Good BM, Todd MH, Edwards AM, Chan L, et al. Ten Simple Rules for Cultivating Open Science and Collaborative R&D. Lewitter F, editor. PLoS Comput Biol [Internet]. Public Library of Science; 2013 Sep 26 [cited 2018 Sep 25];9(9):e1003244. Available from: http://dx.plos.org/10.1371/journal.pcbi.1003244.
- Müller S, Ackloo S, Arrowsmith CH, Bauser M, Baryza JL, Blagg J, et al. Donated chemical probes for open science. Elife [Internet]. 2018 Apr 20 [cited 2018 Sep 25];7. Available from: https://elifesciences.org/articles/34311.
- Edwards A, Morgan M, Al Chawaf A, Andrusiak K, Charney R, Cynader Z, et al. A trust approach for sharing research reagents. Sci Transl Med [Internet]. American Association for the Advancement of Science; 2017 May 31 [cited 2018 Oct 3];9(392):eaai9055. Available from: http://www.ncbi.nlm.nih.gov/pubmed/28566431.
- Drewry DH, Wells CI, Andrews DM, Angell R, Al-Ali H, Axtman AD, et al. Progress Towards a Public Chemogenomic Set for Protein Kinases and a Call for Contributions. bioRxiv [Internet]. 2017 [cited 2017 Apr 17]; Available from: http://biorxiv.org/content/early/2017/01/31/104711.
- Elkins JM, Fedele V, Szklarz M, Abdul Azeez KR, Salah E, Mikolajczyk J, et al. Comprehensive characterization of the Published Kinase Inhibitor Set. Nat Biotechnol [Internet]. 2016 Jan 26 [cited 2017 Jun 5];34(1):95–103. Available from: http://www.nature.com/doifinder/10.1038/nbt.3374.
- Baell JB, Holloway GA. New Substructure Filters for Removal of Pan Assay Interference Compounds ( PAINS ) from Screening Libraries and for Their Exclusion in Bioassays. J Med Chem. 2010;53:2719–40.
- Baell JB. Screening-Based Translation of Public Research Encounters Painful Problems. ACS Med Chem Lett [Internet]. American Chemical Society; 2015 Mar 12 [cited 2016 Nov 23];6(3):229–34. Available from: http://pubs.acs.org/doi/abs/10.1021/acsmedchemlett.5b00032.
- Aldrich C, Bertozzi C, Georg GI, Kiessling L, Lindsley C, Liotta D, et al. The Ecstasy and Agony of Assay Interference Compounds. J Chem Inf Model. American Chemical Society; 2017 Mar;57(3):387–90.
- Baell J, Walters MA. Chemistry: Chemical con artists foil drug discovery. Nature [Internet]. 2014 Sep 24 [cited 2018 Sep 25];513(7519):481–3. Available from: http://www.nature.com/doifinder/10.1038/513481a.
- Alves VM, Muratov EN, Capuzzi SJ, Politi R, Low Y, Braga RC, et al. Alarms about structural alerts. Green Chem [Internet]. Royal Society of Chemistry; 2016 [cited 2016 Aug 9];18(16):4348–60. Available from: http://xlink.rsc.org/?DOI=C6GC01492E.
- Capuzzi SJ, Muratov EN, Tropsha A. Phantom PAINS: Problems with the Utility of Alerts for Pan-Assay INterference Compound. S. J Chem Inf Model [Internet]. American Chemical Society; 2017 Mar 27 [cited 2017 Apr 13];57(3):417–27. Available from: http://pubs.acs.org/doi/abs/10.1021/acs.jcim.6b00465.
- Chakravorty SJ, Chan J, Greenwood MN, Popa-Burke I, Remlinger KS, Pickett SD, et al. Nuisance Compounds, PAINS Filters, and Dark Chemical Matter in the GSK HTS Collection. SLAS Discov Adv Life Sci R&D [Internet]. SAGE PublicationsSage CA: Los Angeles, CA; 2018 Jul 26 [cited 2018 Sep 25];23(6):532–45. Available from: http://journals.sagepub.com/doi/10.1177/2472555218768497.
- Rogues’ Gallery [Internet]. Wikipedia. [cited 2018 Sep 25]. Available from: https://en.wikipedia.org/wiki/Rogues%27_gallery.
- Irwin JJ, Duan D, Torosyan H, Doak AK, Ziebart KT, Sterling T, et al. An Aggregation Advisor for Ligand Discovery. J Med Chem [Internet]. American Chemical Society; 2015 Sep 10 [cited 2018 Sep 25];58(17):7076–87. Available from: http://pubs.acs.org/doi/10.1021/acs.jmedchem.5b01105.
- Sterling T, Irwin JJ. ZINC 15–Ligand Discovery for Everyone. J Chem Inf Model [Internet]. American Chemical Society; 2015 Nov 23 [cited 2016 Apr 1];55(11):2324–37. Available from: http://dx.doi.org/10.1021/acs.jcim.5b00559.
- Scott AR. Chemical probes: A shared toolbox. Nature [Internet]. 2016 May 12 [cited 2018 Sep 25];533(7602):S60–1. Available from: http://www.nature.com/articles/533S60a.
- Matlock M, Swamidass SJ. Sharing Chemical Relationships Does Not Reveal Structures. J Chem Inf Model [Internet]. American Chemical Society; 2014 Jan 27 [cited 2018 Sep 25];54(1):37–48. Available from: http://pubs.acs.org/doi/10.1021/ci400399a.
[1] We have elected to use nuisance compounds in place of pan-assay interference compounds (PAINS) throughout this manuscript. We prefer to use “PAINS” as those compounds flagged by the substructural filters developed by Baell and Holloway.6
Related topics
Analysis, Analytical Techniques, Drug Discovery, Hit-to-Lead, Research & Development, Screening, Therapeutics
Related organisations
North Carolina University