Beyond ‘simple’ biology – turning organoids, spheroids, and 3D tissue models into physiologically relevant high-content assays for drug discovery
Posted: 11 December 2018 | Matthias Nees and Malin Åkerfelt (University of Turku - Finland) | No comments yet
Drug discovery is still hampered by the routine use of cell-based models that often fail to recapitulate the truly relevant biological complexity of the corresponding disease. Just moving from two- (2D) towards three-dimensional (3D) cell culture model systems like spheroids and organoids is not enough to generate truly representative and predictive assays for high-content screening (HCS) or personalised medicine.
The more physiological complexity one tries to capture, the more difficult the corresponding 3D assays become to analyse, miniaturise, validate, and automate. Any attempt to mimic the high biological complexity of tissues – and even a fraction of their heterogeneity – is still intricately linked to low experimental throughput, higher prize, difficulties with standardisation, and possibly poor reproducibility. Or so it was.
Multiple avenues can be taken to overcome these technological hurdles. There is much promise in the diverse and innovative fields of matrix biology and machine vision, 3D bioprinting, and advanced lab-on-a-chip combined with microfluidics technologies.1 Organoids and simple spheroid models are a widely established tool in personalised medicine, particularly in basic cancer research today, but more complex models are only reluctantly adapted in screening – and certainly not in high-throughput assays. To generate more predictive in vitro models for early-stage drug discovery, emerging 3D models still require meticulous characterisation and comparison with each other, mainly with the pathophysiology and tissue characteristics of the disease. To increase the predictive value of 3D assays and to reduce attrition in clinical trials, the role of the extracellular matrix (ECM), the microenvironment, tissue-specific differentiation and cell-cell interactions must be more seriously addressed. This is perhaps most important in the field of oncology. More attention must also be given to the heterogeneity of tissues; eg, the interaction of stromal and epithelial (cancer) cells in 3D co-cultures, or dynamic features such as cell motility and invasion. Traditional metabolic endpoint readouts should be replaced or, if possible, combined with automated image analyses to allow multivariate assays.
Goodbye flat high-content screening?
Over the last decade, three-dimensional cell and tissue cultures have become almost commonplace. It is widely accepted that organoids and spheroids are, in principle, superior to 2D cell cultures on plastic and recapitulate at least some features of the disease biology reasonably well.2 The recent success of organoid cultures – eg, of primary cancer cells (patient-derived organoids or PDO, living biobanks) – has started to replace panels of ‘classic’ cell lines for drug target validation, personalised medicine, animal models, and hopefully soon for drug discovery.3 However, the speed of analyses and ease of standardisation are still too often considered more critical than the biological relevance of the models. As a result, only very few examples have been demonstrated in which drug responses in vitro faithfully predict patient outcome4 – in personalised medicine, not yet in drug discovery. The exceeding time frame for generation of PDOs so far severely handicaps their translation into screening facilities.
There is also too little consensus regarding what might be truly critical parameters for generating the most informative and physiologically-relevant models. These requirements were shown to behave differently between disease models and cell/tissue types, as well as between PDOs from different cancer patients or different tumour subtypes.5 Such factors as cell culture conditions, growth factors, serum or serum-free media, the nature and density of the ECM and other add-ons cannot be easily transferred even between related models. They require meticulous and repeated optimisation, followed by characterisation of biomarker expression and evaluation of the most relevant pathway activities, which must be adapted as part of the screening readout.
Matrix-free biology – spontaneous formation of spheroids
The observation that many cell types spontaneously form spheroids by aggregation when deprived of adhesion to a solid plastic substrate is long held and dates back to the 1970s.6 Most spheroids essentially represent dense aggregates of cells struggling to hold on for dear life, in a hostile environment devoid of pro-survival signalling, but typically provided integrin and other adhesion receptors. It is not widely known that spheroid formation in ultra-low attachment plates or by the hanging drop method can cause massive changes in gene expression, differentiation status and cell-cell interactions.7-8 The procedure also appears to enhance trans-differentiation of tumour cells such as epithelial-to-mesenchymal-transition (EMT), and stress response pathways like NFkB.8 This is promoted by hypoxia observed within large spheroids > 300µM diameter. In some cases, gene expression signatures characteristic of stem cells were markedly increased in spheroids, indicating partial de-differentiation of epithelial, stromal or neuronal cells within spheroids.9
The exact opposite can also be true: spheroids formed by hepatocytes, even when mutation-bearing cancerous hepatoma cell lines such as HepG2 are used, consistently show increased expression of metabolic and synthetic enzymes such as critical P450 family.10 Even functional bile canaliculi are formed inside such spheroids.11,12 Thus, the biological relevance of spheroids for HCS purposes must be carefully validated on a case-by-case basis. On the positive side, increasing stem-cell characteristics within the depths of larger spheroids render these a valuable model for compound screening that specifically addresses stemness or the effects or hypoxia.
Biologically-relevant matrix promotes development of functional organoids
In contrast to spheroids, the formation of biologically-functional organoids and PDOs is either derived from induced pluripotent stem cells (iPS), or better from native stem cells that reside in the primary tissues.13 Organoids from cell lines also readily form when single tumour cells are embedded into supportive matrix, media and growth factors.14,15 Typically, the ECM in these models is Matrigel, which represents a solubilised basement membrane and contains large but variable amounts of laminin, fibronectin, collagens type I and IV, and thousands of other proteins including many growth factors. Matrigel often gets a ‘bad press’ when used for screening and standardisation purposes, due to batch-to-batch variations and its impossible-to-characterise complexity. However, the research community continues to use Matrigel and other laminin-rich matrices on a large scale, simply because – it works! Laminin and collagens are recognised by cell surface adhesion receptors, most importantly the integrins. Integrin signalling provides slow-growing organoids with the necessary pro-survival, anti-apoptotic stimuli and topical information for tissue-specific differentiation. Due to the strong environmental cues exerted by laminin, seemingly normal-like phenotypes can dominate over the transformed genotype of aggressive cancers in Matrigel.16 Such 3D models also recapitulate the intrinsic epithelial plasticity observed in many solid cancers (Figure 1).
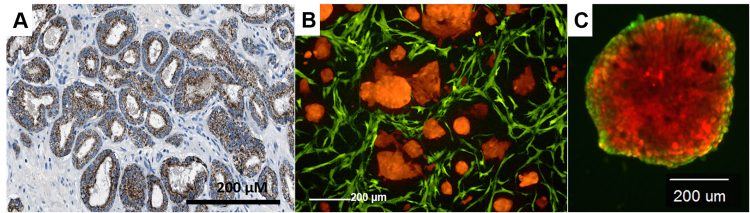
Figure 1: Recapitulation of prostate cancer tissues (histological image, left) by 3D co-culture of tumor and stromal cells (middle), as compared to a single organoid (right) derived from re-aggregation for only tumor cells.
The shape, size and differentiation of organoids demonstrate strong dynamic features, such as cell motility and invasion, that correlate with tumour aggressiveness.17 This is unfortunately not part of the focus of many screening campaigns – but would be very informative for fighting aggressive, metastatic cancers. Laminin-rich ECM also supports the spontaneous formation of complex tissue-like architectures in 3D co-culture models.18 So far, 3D co-culture systems have seen very little application in HCS: the research community already struggles with much simpler 3D monoculture models. Homogeneous assays (TGA, tumour growth assays) that also contain tumour and stromal cells have been used for over a decade in compound screening, although typically with simplified metabolic plate reader-friendly readouts.19 Only very few attempts have been made to quantitatively address the complex phenotypic information from 3D co-culture systems.20,21 The image analysis and machine vision tools to adopt such complex 3D models for high-content screening still require much work.
Alternatives to Matrigel abound; eg, in the form of synthetic hydrogels. The intention is clear: to replace an exceedingly complex ‘natural’ preparation with a chemically-defined product that still mimics the main functions of the ECM. Hydrogels based on PEG polymers (polyethylene glycol), alginate, or cellulose, can be functionalised by attaching peptides that serve as ligands for cellular receptors. This field has become highly diverse over the past few years; it appears that many cells accept artificial hydrogels rather well and often form fairly functional organoids.22 After embedding cells into chemically defined ECM, cells may also secrete critical ECM components themselves, satisfying their own needs for local adhesion/survival stimuli. The nature of this self-made ECM requires further investigation, but it’s difficult to explore using standard technologies.
One interesting alternative to ECM preparations is the re-population of genuine scaffolds generated by de-cellularised tissues with cells – although this may not result in miniaturised disease models for HCS any time soon. A clear advantage is the retention of the natural complexity of extracellular structures, including blood vessels and basement membranes. A similar approach has been successfully introduced by23 performing chemosensitivity tests on tumour explant cultures that retain much of the original tissue heterogeneity. This approach can be applied for personalised medicine, but also for (small-scale) compound screens.
Conclusions
The complexity of disease (and normal) tissues can be increasingly well recapitulated by combining several technologies that do not exclude, but instead strongly rely on, the presence of extracellular and microenvironmental scaffolds. The preferred readout for 3D assays should be considered as equally important as their design: endpoint analyses are fine in many cases, but capturing the heterogeneity and complexity provides so much more. However, this requires fast and detailed, automated image analysis, which is only just emerging.
Biography
Dr Matthias Nees received his PhD at the German Cancer Research Center in 1997. He explored the use of organotypic cultures and 3D models at positions at National Cancer Institute NCI, Heidelberg University Hospital, VTT Technical Research Centre of Finland, and currently coordinates the High Content Screening Laboratory (HCSLab, www.hcslab.fi) at University of Turku in Finland.
Malin Åkerfelt is a Senior Scientist at High-Content Screening Laboratory at Institute of Biomedicine, University of Turku, Finland. She defended her PhD in cell biology at Åbo Akademi University in 2009. Since then she has worked with development of organotypic 3D cell culture models, live-cell imaging and high-content screening.
References
- Rothbauer M, Zirath H, Ertl P. Recent advances in microfluidic technologies for cell-to-cell interaction studies. Lab on a Chip. 2018.
- Marina S, Bissell MJ. Organoids: a historical perspective of thinking in three dimensions. J Cell Biol. 2017.
- Drost J, Clevers H. Organoids in cancer research. Nature Reviews Cancer. 2018.
- Vlachogiannis G, Hedayat S, Vatsiou A, Jamin Y, Fernández-Mateos J, Khan K, et al. Patient-derived organoids model treatment response of metastatic gastrointestinal cancers. Science (80-). 2018.
- Van De Wetering M, Francies HE, Francis JM, Bounova G, Iorio F, Pronk A, et al. Prospective derivation of a living organoid biobank of colorectal cancer patients. Cell. 2015.
- Sutherland RM, McCredie JA, Inch WR. Growth of Multicell Spheroids in Tissue Culture as a Model of Nodular Carcinomas<xref ref-type=”fn” rid=”FN2″>2</xref>. JNCI J Natl Cancer Inst. 1971.
- Karlsson H, Fryknäs M, Larsson R, Nygren P. Loss of cancer drug activity in colon cancer HCT-116 cells during spheroid formation in a new 3-D spheroid cell culture system. Exp Cell Res. 2012.
- Kumar M, Allison DF, Baranova NN, Wamsley JJ, Katz AJ, Bekiranov S, et al. NF-κB Regulates Mesenchymal Transition for the Induction of Non-Small Cell Lung Cancer Initiating Cells. PLoS One. 2013.
- Pastrana E, Silva-Vargas V, Doetsch F. Eyes wide open: A critical review of sphere-formation as an assay for stem cells. Cell Stem Cell. 2011.
- Tostões RM, Leite SB, Serra M, Jensen J, Björquist P, Carrondo MJT, et al. Human liver cell spheroids in extended perfusion bioreactor culture for repeated-dose drug testing. Hepatology. 2012.
- Ramaiahgari SC, Den Braver MW, Herpers B, Terpstra V, Commandeur JNM, Van De Water B, et al. A 3D in vitro model of differentiated HepG2 cell spheroids with improved liver-like properties for repeated dose high-throughput toxicity studies. Arch Toxicol. 2014.
- Abu-Absi SF, Friend JR, Hansen LK, Hu WS. Structural polarity and functional bile canaliculi in rat hepatocyte spheroids. Exp Cell Res. 2002.
- Sato T, Vries RG, Snippert HJ, Van De Wetering M, Barker N, Stange DE, et al. Single Lgr5 stem cells build crypt-villus structures in vitro without a mesenchymal niche. Nature. 2009.
- Lee GY, Kenny PA, Lee EH, Bissell MJ. Three-dimensional culture models of normal and malignant breast epithelial cells. Nat Methods. 2007.
- Härmä V, Virtanen J, Mäkelä R, Happonen A, Mpindi JP, Knuuttila M, et al. A comprehensive panel of three-dimensional models for studies of prostate cancer growth, invasion and drug responses. PLoS One. 2010.
- Kenny PA, Bissell MJ. Tumor reversion: Correction of malignant behavior by microenvironmental cues. International Journal of Cancer. 2003.
- Han J, Chang H, Giricz O, Lee GY, Baehner FL, Gray JW, et al. Molecular predictors of 3D morphogenesis by breast cancer cell lines in 3D culture. PLoS Comput Biol. 2010.
- Åkerfelt M, Bayramoglu N, Robinson S, Toriseva M, Schukov H-P, Härmä V, et al. Automated tracking of tumor-stroma morphology in microtissues identifies functional targets within the tumor microenvironment for therapeutic intervention. Oncotarget. 2015.
- Krausz E, De Hoogt R, Gustin E, Cornelissen F, Grand-Perret T, Janssen L, et al. Translation of a tumor microenvironment mimicking 3D tumor growth co-culture assay platform to high-content screening. J Biomol Screen. 2013.
- Härmä V, Schukov HP, Happonen A, Ahonen I, Virtanen J, Siitari H, et al. Quantification of dynamic morphological drug responses in 3D organotypic cell cultures by automated image analysis. PLoS One. 2014.
- Ahonen I, Åkerfelt M, Toriseva M, Oswald E, Schüler J, Nees M. A high-content image analysis approach for quantitative measurements of chemosensitivity in patient-derived tumor microtissues. Sci Rep. 2017.
- Gjorevski N, Sachs N, Manfrin A, Giger S, Bragina ME, Ordóñez-Morán P, et al. Designer matrices for intestinal stem cell and organoid culture. Nature. 2016.
- Majumder B, Baraneedharan U, Thiyagarajan S, Radhakrishnan P, Narasimhan H, Dhandapani M, et al. Predicting clinical response to anticancer drugs using an ex vivo platform that captures tumour heterogeneity. Nat Commun. 2015.
Related topics
Disease Research, Drug Targets, Organoids, Research & Development, Therapeutics
Related organisations
University of Turku - Finland