Spotlighting data in upstream bioprocesses – a recipe for quick and successful cell lines
Posted: 17 September 2019 | Unjulie Bhanot (IDBS) | No comments yet
Upstream bioprocessing is the epicentre of biologics development, wherein scientists piece together a series of carefully chosen processes with contributing components and parameters to enable the production of highly effective biotherapeutics. Unjulie Bhanot explains why an effective data management system is vital in this quest for the next big therapeutic.
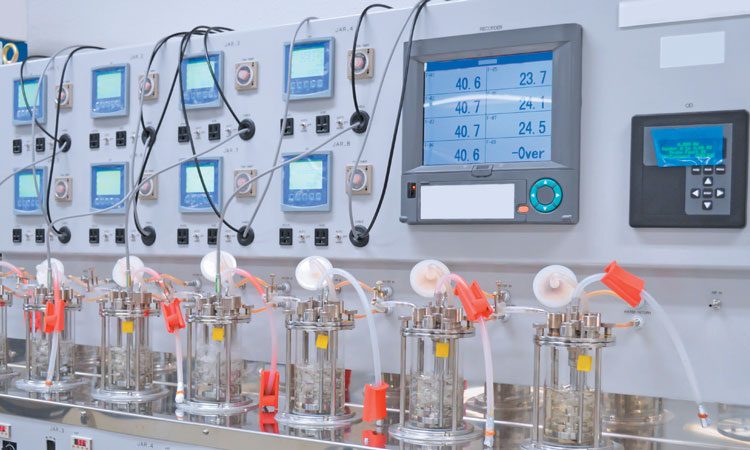
TO GENERATE pure populations and high yields, organisations invest huge amounts of time, money and resource into defining and refining the expression, culturing, fermentation and harvesting steps of development. In an industry that is anticipated to grow at a CAGR of almost 10 percent,1 the pressure is on to get high quality and effective biotherapeutics to market faster. However, with almost 800 molecules expected in the pipeline over the next 10 years,1 maximising stable drug production at a high concentration with the desired attributes and required quality may prove to be a challenge.
While the ability to use a cell’s inherent machinery as a vehicle for biologics development is beneficial, the cell is designed to produce more than just the product of the desired gene. Accounting for minimal undesirable post-translational protein modification and generation of excessive host cell proteins can mean scientists spend hours of their time qualifying and requalifying their methodologies.
The journey from cell to culture
As organisations begin their process development phase, an appropriate expression system must be determined to develop the product cell line. Considerations include:
- The number of amino acids in the gene sequence
- The expression vector’s chances of successful uptake of the gene and amount of protein required
- The expression vector’s own behaviour; eg, potential for post-translational modifications such as glycosylation or additions requiring cleaving.
At this stage, organisations are faced with questions around how similar this molecule is to one that has been produced before. Can the same expression system be used? Is there data to suggest the potential success or failure rate of a vector?
To tackle these questions, it is essential to have a co-ordinated data management system; one in which historical data can quickly be recalled to make sequencing comparisons, recall expression vector data associated with a similar version of the molecule at hand, identify the culturing requirements and potential process steps of the vector and the expected growth rate.
Data is often buried in the minds of scientists, paper notebooks and numerous Excel spreadsheets”
This data is often buried in the minds of scientists, paper notebooks and numerous Excel spreadsheets. Since these formats are difficult to combine and assimilate, additional effort is expended running a process with no assumed knowledge, when in fact, the information does exist.
Larger biologics development organisations may have better-documented platform procedures but suffer from data overload in a multiplicity of formats. Sifting through too much data that cannot be queried or filtered is equally tiresome and detracts scientists from being able to focus on the science.
A question of artificial intelligence
If data can be recorded, mapped and associated correctly in an electronic format, can organisations re-use existing data through learned prompting?
As transfected cells are taken forward for expression screening under parameters such as varied incubation conditions, vehicles, growth media, etc, the traceability of the cells, their containers, positions in plate wells and their corresponding metadata becomes critical to the success of the process.
Imagine keeping track of all these disparate details across multiple Excel spreadsheets or manually creating IDs for cell references on a plate or reconciling vial IDs to results from an analysis system. It starts to sound quite complicated. Knowing which cells and conditions are associated with a particular ‘location’ is imperative to discern whether the biologic generated is correct. Where several combinations are screened, data volumes can explode – for example, Molecular Devices’ ClonePix2 can screen up to 10,000 clones in three weeks,2 for which there is both image and numerical data.
Additional properties such as the cell density and cell count are important to measure, especially as culturing conditions are formalised – scientists must balance achieving high quantities of the product with the productivity of the process and the presence of impurities and dead cells.
These parameters are either measured using bespoke software or taken manually. They are critical for establishing the stability of a cell line. As the development of a cell line moves from 384-well down to six-well plates, to other containers such as cell culture flasks and shake flasks to generate the seed train, other significant factors to consider include the nature of cell growth (adherent or suspension), gas exchange, temperature maintenance, etc.
The growth phases of cells… and data
The process of cell culturing focuses on maintaining the stability of a cell line and establishing optimal growth conditions, which lays the foundations for producing the therapeutic on a larger scale. The passaging of cells aims to keep them in their exponential growth phase to maintain consistency in their genetic and phenotypic expression.3 Since culturing must be performed aseptically in a tissue culture hood, this step is often recorded manually, with individual annotations per flask noting the passage number, conditions, date of seed and volume and date of media change.
Accounting for minimal undesirable post-translational protein modification and generation of excessive host cell proteins can mean scientists spend hours of their time qualifying and requalifying their methodologies”
Vendors of cell culturing flasks are increasingly becoming aware of the data integrity challenges and scientific risks associated with this manual transcription and have consequently developed barcoded flasks;4 the data against which needs to be associated by a scientist in an inventory management system. The traceability and genealogical linking of these flasks when generating the seed train must not be overlooked; the accurate and immediate recording of data is imperative.
Using an integrated data management system for this removes the burden from the scientist to maintain this information; it allows the system to generate unique IDs, create linkages between entities and keep track of volumes and pooling. Ideally, scientists can then call upon that information in an experiment, which is where this proves most useful so that scientists need not handle or repeat data across different systems.
Once a sufficient density and volume of cells has been generated, scientists will select the size and type of bioreactor and the volume of inoculum to use; an activity that requires more data collation and mathematical planning than first meets the eye. For example, not only is there discussion around the use of single-use bioreactors versus stainless steel stirred tank bioreactors, but scientists must also plan for the scale of production expected, the duration in which cells will reach optimal production and conditions of the run such as oxygen supply levels, flow rates, shaker speeds and substrate concentrations.
During the run of a bioreactor, scientists will perform a series of different raw data analyses (in-line, at-line, off-line, etc), which can entail the automatic or manual creation of samples for in situ or external testing while also continuously monitoring input and output levels of key materials of the run using the associated control unit.
With the average mammalian bioreactor run spanning 10-14 days,5 this can generate huge volumes of data across different time points (varying from seconds to minutes to hours), manually keeping track of which is a herculean task. To complicate this further, multiple bioreactors with the same or different conditions can be run in parallel, leaving the scientist with reams of data points – but not always information that can be surfaced and used quickly.
Today, organisations rely on high-throughput instruments such as multi-parallel bioreactors6 that enable 12-48 mini bioreactors to run in parallel. These allow organisations to screen for the optimum combinations of media, feeds and operating conditions to take top performers through to bench scale and simulate manufacturing runs. This strategy is designed to effectively manage laboratory space, resource, media and consumables with some almost halving the time spent on process optimisation.7
Of course, with the ability to define and perform so many runs at one time, the volume of data multiplies accordingly – be this setpoint information, monitoring data or analysis results. Often this data is analysed and reviewed in either the host proprietary software or Excel spreadsheets. While taken individually these software allow users to manipulate and analyse data, their roles are significantly localised to the operation at hand. Finding and consolidating the data across multiple runs, parameters and unit operations is still carried out manually; an activity that can cost scientists up to five hours per week.8
The handover to downstream processing
When taking the scientific decision to harvest the biologic-producing cells from the bioreactor, scientists aim to do this at a point of high cell viability. Identifying this stage requires continuous monitoring of the bioreactor.
However, aside from the viability, downstream scientists must know key attributes pertaining to the material they receive: the expression system used, the impurities or host cell proteins they can expect to encounter, the desired or unwanted post-translational modifications they should account for when defining their processes, the yields and titres achieved from preceding steps, etc, in order to make decisions regarding the most suitable downstream methodology.
Given that relaying this information often involves the manual intervention of a scientist – either through email, a hand-written label or a face-to-face conversation – this presents the possibility that key information is missed or erroneously transcribed, which can have harmful consequences. For example, incorrect information about post-translational modifications can lead to miscalculations regarding the molecule’s stability, solubility and aggregation.5 Inaccurate information can cause repeated rework for the downstream team.
Additionally, for downstream scientists, knowing whether a similar protein and its (platform) development process exists is of immense value to the organisation. After all, the strategy is to streamline development to shorten the overall time to market.
From choosing the optimal cell line through to optimising the media and conditions and scaling up the production of a therapeutic-producing cell line, the amount of data recorded grows exponentially given the multitude of instrumentation and the iterative nature of development steps.
It is clear to see that while these steps themselves take time, there is inevitably another factor of time that must be considered; for the collation and presentation of the relevant data from which to make decisions about the product and the process.
Given the resource and material burden, it is therefore unsurprising that there has been a surge in automation instrumentation, custom-built software and thus data management tools within upstream development. With each bespoke system, vast amounts of high-value scientific and process data can end up stored in disparate locations and systems in unstructured and structured formats, and organisations can often lose sight of how the business will need to share and make use of this data overall. Consequently, they are pushed to urgently implement any viable data management strategy.
An effective data management strategy underpins the success of this domain. It will be centred around a platform that can connect process and product data – one that can streamline results data acquisition efficiently, while maintaining data integrity through direct integrations, establish relationships between experiment metadata and experimental outcomes and enforce linkages automatically between consumed and generated materials in experiments. Most importantly to the cell-line development and upstream teams, it must associate data to a relevant ontology such that data can be quickly and reliably resurfaced in order to make process and product decisions and share information. A digital platform that promotes re-use of high-value knowledge will empower biologics development organisations to realise the full benefits of their scientific investments and get their therapeutic to market faster.
About the author
Unjulie Bhanot is a UK-based Solutions Consultant at IDBS and has worked in the biologics R&D informatics space for over five years. Unjulie holds a BSc in Biochemistry and an MSc in Immunology, both from Imperial College London. Prior to joining IDBS, Unjulie worked as an R&D scientist at both Lonza Biologics and UCB, and later went on to manage the deployment of the IDBS E-WorkBook Platform within the analytical services department at Lonza Biologics in the UK.
References
- Global Biologics Market Size, Market Share, Application Analysis, Regional Outlook, Growth Trends, Key Players, Competitive Strategies and Forecasts, 2018 to 2026, Research and Markets, April 2018
- ClonePix 2 Mammalian Colony Picker Product Brochure https://www. moleculardevices.com/ sites/default/files/en/ assets/product-brochures/ biologics/clonepix2- system.pdf
- Masters JR, Stacey GN. Changing medium and passaging cell lines, Nature Publishing Group Protocol, Sept 2007
- Nunc TripleFlask Cell Culture Flasks, ThermoFisher Scientific https://www.thermofisher. com/uk/en/home/life- science/cell-culture/cell- culture-plastics/ cell-culture-flasks/t500- flasks.html
- Biopharmaceutical Processing: Development, Design, and Implementation of Manufacturing Processes, Edited by Günter Jagschies, Eva Lindskog, Karol Łącki, Parrish Galliher, Elsevier, 2018
- Mayer-Bartschmid A, Trautwein M, Mueller- Tiemann B. Getting Cell Line Development off the critical path in Biologics Drug Discovery, Biologics Congress, 2nd & 3rd Feb 2015
- Li J, Zoro B, Wang S, Weyand J. Case Study: Shortening Timelines for Upstream Bioprocessing of Protein-based Therapeutics, Sartorius, BiopharmAsia Nov-Dec 2015
- Making the Most of Drug Development Data – Pharmaceutical Manufacturing, 01 December 2005 https:// www.pharma manufacturing.com/ ticles/2005/399/?show=all
Related topics
Biotherapeutics, Cell Cultures, Cell Line Development, Informatics, Screening
Related organisations
NanoTemper