Using big data approaches to develop cell therapies
Posted: 4 June 2020 | Dr Owen Rackham (Duke-NUS Medical School) | No comments yet
An area where stem cell biology and medicine are combining effectively is the establishment of new cell therapies. However, current therapies are limited to a narrow set of cell types that can be isolated or created and expanded in vitro. Dr Owen Rackham discusses how utilising computational approaches will further enhance applications of stem-cell-derived therapies in the future.
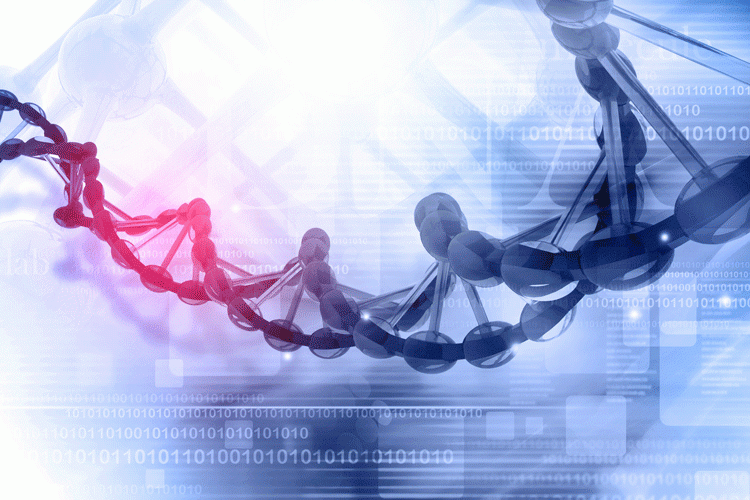
For decades (or perhaps centuries) the approach in cell biology has remained relatively unchanged. We isolate cells and with our confined knowledge of their endogenous conditions, begin to experiment until we can sustain them in vitro. Once established, we can conduct further investigation to assess a cell’s response to different conditions, changes over time or response to manipulation. This is especially true of stem cell biology, established from tireless efforts to incrementally improve culture conditions or differentiation protocols based on fragmented knowledge of developmental processes. Despite this, the promise of stem-cell therapies is already being realised in the clinic, but the breadth of cell types being used is still relatively narrow. Recent technological advances in the field have been focused on the safe and scalable manufacture of therapies. While these are revolutionary breakthroughs, the applications are largely limited to T cells, haematopoietic- and pluripotent-stem cells (HSCs and PSCs), a small fraction in the grand heterogeneity of cell types. Consequently, the lack of cell source diversity prevents cell therapy from fulfilling its clinical potential, pointing to the need for new means to isolate or generate source cells.
Stem-cell therapies: a historical perspective
Stem cells possess the ability (i) to self-renew and (ii) to differentiate into at least one other cell type and often various cell types. In theory, this potency gives PSCs, ie, embryonic and induced pluripotent stem cells (ESCs/iPSCs), the ability to transform into any somatic cell type. Conversely, adult stem cells, such as HSCs, are limited to the cell types within their lineage tree. The HSC differentiation ability and ease of isolation has enabled the development of the first generation of stem-cell therapies. These transplant-based therapies rely on the natural ability of the HSCs to confer some therapeutic benefit. They are widely used in bone marrow transplants to reconstitute the immune system of a patient undergoing chemotherapy for leukaemia, a procedure performed thousands of times annually across the globe1 and first performed over 60 years ago.2
The second generation of cell-based therapies emerged with PSC-derived cells for transplantation. Early applications were focused on retinal pigment epithelial cells (RPEs) (for macular degeneration),3 oligodendrocytes (for spinal injuries), pancreatic endoderm (for diabetes)4 and cardiomyocyte progenitors (for myocardial infarctions).5 Some of these products have proven to be effective, but have required improvements for safety and scalability. For instance, incomplete differentiation of PSCs presents a tumourigenic risk,6 a problem that has been overcome in existing therapies but remains a concern in future development. These challenges are increasingly addressed by technological advancements, reducing technical barriers and manufacturing difficulties, to make progress towards future therapies.
The lack of cell source diversity prevents cell therapy from fulfilling its clinical potential”
The third (or next) generation of cell-based therapies has advanced to endow upon new capabilities through cell engineering. Recently, the engineered expression of antigen-specific T-cell receptors (TCRs) or the chimeric antigen receptors (CAR) has enabled T cells to target specific proteins, such as those on the surface of tumour cells. Importantly, the approval of products such as KYMRIAH® and YESCARTA® has shown that manufacturing and regulatory infrastructures have now matured to support these products. Overall, these three generations of therapies have provided much optimism about the future of stem-cell-based therapies. However, despite incredible progress, one aspect of development appears to be falling behind – the breadth of applicable source cell types.
As outlined in Figure 1, current estimates are that there are over 450 human cell types.7 Of these, only 56 can be generated by differentiation8 and 41 by transdifferentiation.9 In either case, the quality of the generated cells is questionable, with many protocols generating immature cells or cells expressing partial phenotype. As a result, only 39 different cell types have been subjected to clinical trials and according to the UK Cell and Gene Therapy Catapult clinical trials database,10 only around 10 different cell types are currently being used in on-going trials. The majority of clinical studies involve T-cell or HSC-based therapies, owing to their historical prevalence and proven efficacy, but many other cell types have yet to be explored for therapeutic use. This represents an exciting opportunity for stem-cell-based therapies; building on lessons learnt from existing cell therapies and developing new data-driven tools will help to enable an expansion of available cell types. In turn, this could reveal new opportunities in research and treatment options.
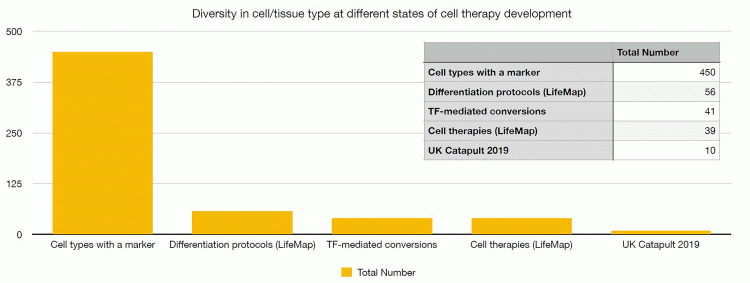
Figure 1: The breadth of cell/tissue types at various stages of cell therapy development. Only a small proportion of known cell types can be generated through differentiation or transdifferentiation and substantially less cell types are being used or developed as cell therapies. The data is taken from the LifeMap resource on differentiation protocols,18 a review of transcription factor (TF)-mediated conversion,19 the LifeMap resource on cell therapies18 and the UK Cell Therapy Catapult survey of clinical trials 2019.10
The data-driven era
Like many disciplines, “big data” is making an impact on stem cell biology. Firstly, single-cell next-generation sequencing data is uncovering several novel rare cell types, many of which are appealing for cell therapy. Secondly, data-driven tools can provide predictions of perturbations to direct cell fate systematically. For instance, it is now possible to use data-driven tools to predict which transcription factors are required to convert between any two human cell types. Combined, these will enable a new data-driven development pipeline for cell therapies, as outlined in Figure 2.
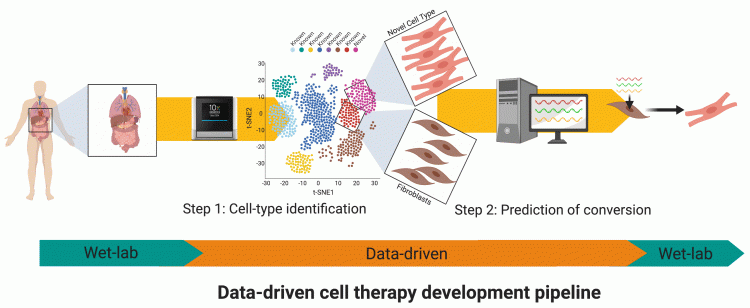
Figure 2: The data-driven pipeline aims to streamline the process of solving combinatorial problems in stem cell biology. This everincreasing pipeline can already find new rare cell types from single-cell data and then predict how to generate them by differentiation or transdifferentiation. Increasingly, these tools will also be able to address other important issues such as what conditions can be used to maintain cells in vitro and how drug combinations can be chosen to correct disease-driven gene expression changes.
Data-driven discovery of cell types
Recent advances in single-cell sequencing technology,11 consortia such as the Human Cell Atlas12 and the development of sophisticated analysis pipelines means that the number of cell types and clinically interesting phenotypes are likely to increase. With every discovery of a new cell type is the possibility of a novel stem-cell-derived therapy, as elucidated by the following examples:
- A novel cell type, called the pulmonary ionocyte, was identified from analysis of single-cell data from the airway epithelial. This cell type only represented around one percent of all cells detected but appears to be the primary source of cystic fibrosis transmembrane conductance regulator (CFTR) protein expression.13
- A study of the human gut at the single-cell level identified 10 cell types, one of which was previously unknown. This novel cell type was a pH-sensing absorptive colonocyte, a function that was not restricted to a single cell type previously and a cell type that appears to be lost in patients with inflammatory bowel disease.14
- A study of the human liver at the single-cell level found several new cell types, including one rare population of cells that could form organoids in vitro and was shown to be able to differentiate into both hepatocytes or bile duct cells.15
Each of these novel cell types has a specialised role within the body. In some cases, the loss of these cell types appears to be enough to trigger disease, whilst in other types is the ability to give rise to cells that are frequently lost or damaged, offering new ways to regenerate tissues. However, for these new cells to be developed as therapies, it is necessary to be able to generate them through differentiation or transdifferentiation, a challenge that data-driven tools are helping to enable.
Data-driven generation of cell types
As early as the 1980s, it was shown that reprogramming between cell types by transcription factor overexpression was possible.16,17 Following the discovery by Yamanaka, there was a renewed excitement that iPSCs could be created by over-expression of four transcription factors in fibroblasts18 and, in principle, derive any somatic cell type. This excitement was promoted by a number of subsequent experiments that described the conversion of fibroblasts to a number of other cell types, including neurons,19 cardiomyocytes20 and hepatocytes21 through different sets of transcription factors. There was hope for a new era of cell therapy where the underlying cell type could be any human cell type. However, to achieve this, the correct set of transcription factors to drive a cell conversion to any cell type is required. Despite this early promise, the field struggled to identify new sets of transcription factors and the number of cell types that could be generated remained relatively small. As there are at least 1,600 transcription factors,22 to identify the best combination of, for example, four, we would have to test 2×1011 sets. Consequently, we have relied on educated guesses and experimental trial-and-error to find combinations that work, but with little or no guarantee that we are headed towards the best solution.
Single-cell next-generation sequencing data is uncovering several novel rare cell types, many of which are appealing for cell therapy”
Recently, a solution has been found in the establishment of algorithms that predict the factors required for improving existing conversions or driving novel cell conversions.23,24 These algorithms integrate data from gene expression and gene regulatory networks to predict which transcription factors can generate the cell type of interest. These approaches have already been used to generate a number of cell types including vascular endothelial cells and keratinocytes,24 as well as improve the generation of hepatocytes and macrophages.25 In each case, the predictions were purely data-driven and needed little trial-and-error to optimise the combination of transcription factors. The only requirements are gene expression data, of the source and target cell types, combined with information on how genes are known to interact.
Taken together, this demonstrates why the future of stem cell biology will require the adoption of big data-driven methods. Throughout its 60-year history, stem-cell therapy has had a number of significant developments, but none has been able to facilitate the adoption of a broad set of cell types. Now with single-cell biology, this problem is set to become even more pronounced. New data‑driven tools are already revealing a large number of rare cell types, many of which possess great therapeutic potential. However, with our current approaches, it is unlikely we will be able to maintain them in vitro or create them by differentiation or transdifferentiation. Subsequently, we will need to turn to data-driven tools for a solution and, in doing so, the promise that we have seen in existing cell therapies will be felt in a much broader range of indications.
About the author
Dr Owen Rackham is an Assistant Professor at the Duke-NUS Medical School in Singapore. He is a world expert in the development of computational approaches for cell reprogramming and disease-gene association. His group works on ways to identify key regulators that can control cell fate in order to find novel routes for cell conversion, as well as identifying drug targets. Originally trained in computer science and machine learning, Owen now combines experimental and computational expertise with a goal of making biology a more data‑driven discipline.
References
1. WHO | Haematopoietic Stem Cell Transplantation HSCtx. 2013 Dec 11 [cited 2020 Apr 30]; Available from: https://www.who.int/transplantation/hsctx/en/
2. Thomas ED, Lochte HL Jr, Cannon JH, Sahler OD, Ferrebee JW. Supralethal whole body irradiation and isologous marrow transplantation in man. J Clin Invest. 1959 Oct;38:1709–16.
3. Lu B, Malcuit C, Wang S, Girman S, Francis P, Lemieux L, et al. Long-term safety and function of RPE from human embryonic stem cells in preclinical models of macular degeneration. Stem Cells. 2009 Sep;27(9):2126–35.
4. Kimbrel EA, Lanza R. Current status of pluripotent stem cells: moving the first therapies to the clinic. Nat Rev Drug Discov. 2015 Oct;14(10):681–92.
5. Garbern JC, Lee RT. Cardiac stem cell therapy and the promise of heart regeneration. Cell Stem Cell. 2013 Jun 6;12(6):689–98.
6. Sato Y, Bando H, Di Piazza M, Gowing G, Herberts C, Jackman S, et al. Tumorigenicity assessment of cell therapy products: The need for global consensus and points to consider. Cytotherapy. 2019 Nov;21(11):1095–111.
7. Zhang X, Lan Y, Xu J, Quan F, Zhao E, Deng C, et al. CellMarker: a manually curated resource of cell markers in human and mouse. Nucleic Acids Res. 2019 Jan 8;47(D1):D721–8.
8. Edgar R, Mazor Y, Rinon A, Blumenthal J, Golan Y, Buzhor E, et al. LifeMap DiscoveryTM: the embryonic development, stem cells, and regenerative medicine research portal. PLoS One. 2013 Jul 17;8(7):e66629.
9. Kamaraj US, Gough J, Polo JM, Petretto E, Rackham OJL. Computational methods for direct cell conversion. Cell Cycle. 2016;1–12.
10. Cell and gene therapy clinical trials [Internet]. [cited 2020 May 4]. Available from: https://ct.catapult.org.uk/clinical-trials-database
11. Stuart T, Satija R. Integrative single-cell analysis. Nat Rev Genet. 2019 May;20(5):257–72.
12. Regev A, Teichmann SA, Lander ES, Amit I, Benoist C, Birney E, et al. The Human Cell Atlas. Elife [Internet]. 2017 Dec 5;6. Available from: http://dx.doi. org/10.7554/eLife.27041
13. Montoro DT, Haber AL, Biton M, Vinarsky V, Lin B, Birket SE, et al. A revised airway epithelial hierarchy includes CFTR-expressing ionocytes. Nature. 2018 Aug;560(7718):319–24.
14. Parikh K, Antanaviciute A, Fawkner-Corbett D, Jagielowicz M, Aulicino A, Lagerholm C, et al. Colonic epithelial cell diversity in health and inflammatory bowel disease. Nature. 2019 Mar;567(7746):49–55.
15. Aizarani N, Saviano A, Sagar, Mailly L, Durand S, Herman JS, et al. A human liver cell atlas reveals heterogeneity and epithelial progenitors. Nature. 2019 Aug;572(7768):199–204.
16. Davis RL, Weintraub H, Lassar AB. Expression of a single transfected cDNA converts fibroblasts to myoblasts. Cell. 1987 Dec 24;51(6):987–1000.
17. Xie H, Ye M, Feng R, Graf T. Stepwise reprogramming of B cells into macrophages. Cell. 2004 May 28;117(5):663–76.
18. Takahashi K, Tanabe K, Ohnuki M, Narita M, Ichisaka T, Tomoda K, et al. Induction of pluripotent stem cells from adult human fibroblasts by defined factors. Cell. 2007 Nov 30;131(5):861–72.
19. Vierbuchen T, Ostermeier A, Pang ZP, Kokubu Y, Südhof TC, Wernig M. Direct conversion of fibroblasts to functional neurons by defined factors. Nature. 2010 Feb;463(7284):1035–41.
20. Ieda M, Fu J-D, Delgado-Olguin P, Vedantham V, Hayashi Y, Bruneau BG, et al. Direct Reprogramming of Fibroblasts into Functional Cardiomyocytes by Defined Factors. Cell. 2010 Aug;142(3):375–86.
21. Sekiya S, Suzuki A. Direct conversion of mouse fibroblasts to hepatocyte-like cells by defined factors. Nature. 2011 Jul 21;475(7356):390–3.
22. Lambert SA, Jolma A, Campitelli LF, Das PK, Yin Y, Albu M, et al. The Human Transcription Factors. Cell. 2018 Oct 4;175(2):598–9.
23. Cahan P, Li H, Morris SA, Lummertz da Rocha E, Daley GQ, Collins JJ. CellNet: Network Biology Applied to Stem Cell Engineering. Cell. 2014 Aug 14;158(4):903–15.
24. Rackham OJL, Firas J, Fang H, Oates ME, Holmes ML, Knaupp AS, et al. A predictive computational framework for direct reprogramming between human cell types. Nat Genet. 2016 Mar;48(3):331–5.
25. Morris SA, Cahan P, Li H, Zhao AM, San Roman AK, Shivdasani RA, et al. Dissecting Engineered Cell Types and Enhancing Cell Fate Conversion via CellNet. Cell. 2014 Aug 14;158(4):889–902.
Related topics
Big Data, Cell Therapy, Chimeric Antigen Receptors (CARs), Drug Development, Induced Pluripotent Stem Cells (iPSCs), Regenerative Medicine, Research & Development, Stem Cells, Therapeutics
Related conditions
Cancer, Cystic fibrosis, Diabetes, Inflammatory bowel disease (IBD), Macular degeneration, myocardial infarction
Related organisations
Cell and Gene Therapy Catapult, Human Cell Atlas