How data science is driving genomics in the pharmaceutical industry
Posted: 7 January 2021 | Ramya Sriram (Kolabtree) | No comments yet
In this article, Ramya Sriram describes how data science is driving innovations in medical biotechnology and genomics.
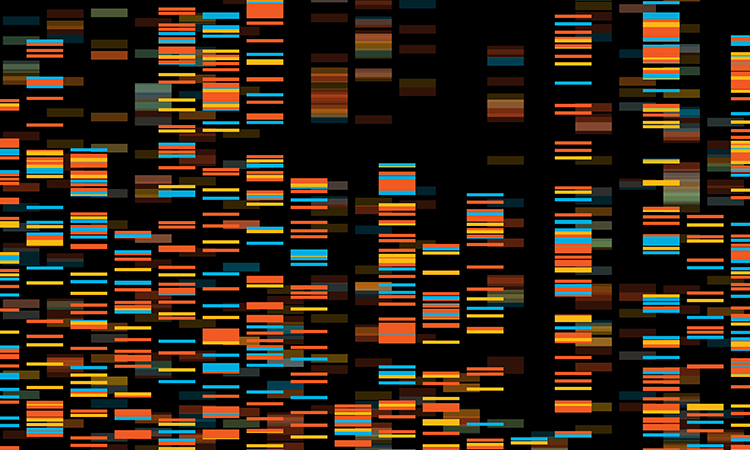
The human body contains a plethora of genomics data. Not only is our DNA made up of about three billion genome bases, if you laid out all the DNA in the human body, it would stretch to twice the diameter of the Solar System and each cell’s DNA would be three meters long.
Biotechnology, the use of living organisms or biological systems and their derivatives to make products, is propelled forward by data, information and statistics. In 2014, according to Science, bioinformatics became a discipline in its own right, rather than a tool in a biologist or biotechnologist’s armoury. Business intelligence, data analytics and technological advances are crucial to the development of new technologies and treatments and to overcome current challenges. By making sense of big data, from genomics or from sensors, we can identify potential drug targets, improve processes, bring new drugs to market and reduce errors in clinical trials.
Genomics
If you think of big data in the context of biotechnology, your first thought likely relates to genome sequencing. The Human Genome Project, which ran from 1990 to 2003, was a pioneering effort that gave us access to three billion bases of data, opening the door to information on mutations, genes and more. We now live in a world where genome data is at our fingertips; it can be sequenced in a few hours for under £1,000.
By making sense of big data, from genomics or from sensors, we can identify potential drug targets”
The data now available provides researchers with the ability to obtain valuable insights in the field of medicine, crime scene investigations, etc. To work with it effectively, data scientists use frameworks and tools to store, track, receive, analyse and interpret data. Tools are now being built to automatically annotate specific genes and software companies like DNAnexus, Knome and NextBio have begun to tackle genome interpretation. Interestingly, NextBio has even worked with Intel to improve Hadoop for genomic big data analysis. The pharmaceutical and healthcare industries can use this insight to improve diagnostics, aid drug discovery or develop personalised medicine strategies.
Drug discovery, development and genomics
Bringing a new pharmaceutical product to market is a long, arduous process with many bottlenecks. Trials regularly fail to meet their objectives, for example in terms of enrolment, which can add further delay and therefore increase the costs of an already expensive process. However before issues from recruiting patients for a clinical trial can take place, scientists first need to identify a drug candidate, which still includes numerous data points, experiments and risk/benefit analyses.
We can now use automated software to screen millions of compounds to identify drug candidates for a clinical trial. Pharmaceutical professionals can let artificial intelligence (AI) do the hard work of sifting through a huge library of potential drugs, assessing what is likely to work against the trial’s specific criteria.
Biotechnology company Numerate, for example, builds predictive models to help with small molecule drug design, making predictions on toxicity, metabolism, absorption, distribution and more. AI can also be used to invent new combinations of compounds. Pharmaceutical companies can therefore screen drug candidates and pick the ones most likely to succeed in clinical trials.
Big data in biotechnology is not only about genomics — the data may also be collected by sensors. Wearable, ingestible or implantable sensors can provide a continuous data stream for clinical trials. This data can reduce the gap between measurements taken at appointments, mitigate for human error, identify reasons for dropout and may allow patients to go about their normal lives more easily. Any improvement in the drug discovery or clinical trial process can save millions of dollars in development costs and therefore speed up the time it takes to bring a potentially life-saving drug to market.
The keys to the future
Data scientists hold the keys to the future of data analytics for medical biotechnology in their hands. For innovation to take place, the industry needs trained data scientists and biotechnicians with skills in programming and coding languages including Python, R, C++ and SQL among others. They also require an underlying knowledge of data collection, storage, algorithms, validation and visualisation to generate meaning from biological data.
The knowledge and skills needed to write in these languages are primarily held by data scientists with advanced degrees, meaning it is important to invest in either freelancers or training for in house talent to make full use of genomic data.
About the author
Ramya Sriram manages digital content and communications at Kolabtree, a freelancing platform for scientists. She has over a decade of experience in publishing, advertising and digital content creation.
Related topics
Artificial Intelligence, Drug Discovery, Genetic Analysis, Genomics, Informatics, Screening
Related organisations
DNAnexus, Human Genome Project, Knome, NextBio, Numerate