Accelerating drug discovery with disruptive computational methods
Posted: 22 September 2021 | Sheraz Gul (Fraunhofer Institute) | No comments yet
Sheraz Gul explores how machine learning and artificial intelligence represent an exciting opportunity for the drug discovery industry, with the potential to develop highly optimised small molecules.
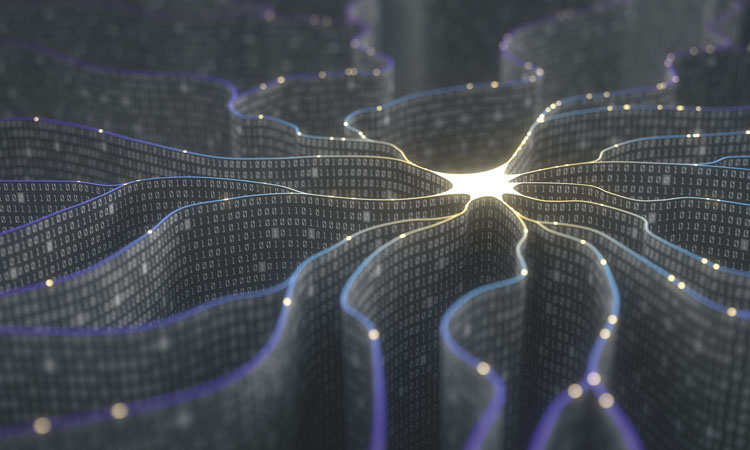
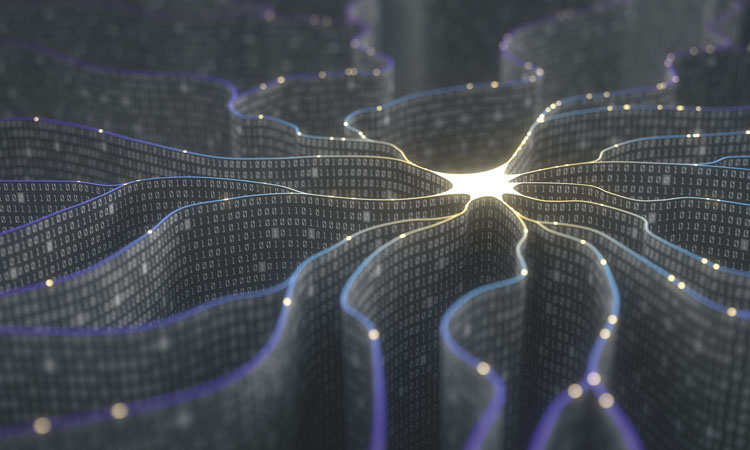
Discovering drugs has all too often been described as a risky, expensive and lengthy process. This is evidenced by the fact that (a) less than a third of new chemical entities entering Phase I clinical trials attain regulatory approval, (b) the average cost for launching a drug is in excess of $1 billion and (c) it typical takes well over 10 years of research, development and clinical trials per drug.1 The latest views from pharmaceutical industry analysts suggest that despite these challenges, a number of disruptive technologies are maturing that are expected to help accelerate drug discovery. These include the development of highly sensitive in vitro bioassay systems that more closely resemble the natural environment of protein drug targets implicated in disease processes, which if used appropriately will ensure experimental findings are more likely to translate in vivo. These systems are more predictive and can be personalised, especially if biomaterials of human origin are employed rather than the commonly utilised cellular systems originating from cancer cell lines.
A newer approach to accelerate drug discovery is the accessibility of advanced computational methods. These methods have been utilised extensively to complement in vitro techniques to progress small molecule drug discovery projects. For example, they can be used for rationalising structure‑activity relationships (SARs) of molecules by modelling and docking studies in protein structures deposited in protein data banks.
One novel aspect of computational methods include machine learning and artificial intelligence (AI) techniques which have successfully designed small molecules that act as modulators of relevant proteins involved in disease processes from scratch. These methods are being further exploited to ensure that the molecules have optimised pharmacokinetics, chemical and pharmacological properties, as well as safety and toxicity profiles.2 This ensures that small molecules are designed as lead candidates very early in the process of drug development. In one particular case, AI has successfully designed a clinic‑ready molecule that targets a specific G protein‑coupled receptor (GPCR), which belongs to the most successful protein target-class in drug discovery with around 25 percent of all approved drugs acting on these proteins. The clinical trial of the molecule is a three-part study to assess its safety, tolerability, pharmacokinetics and pharmacodynamics.3 We eagerly await the readout of the clinical study, which is expected very soon.
About the author
Sheraz Gul is the Head of Assay Development and Drug Repurposing at the Fraunhofer Institute, Germany. He has professional experience in the field of drug discovery, assay development and screening gained whilst employed in academia. He has co-authored more than 80 peer-reviewed papers, book chapters and patents including the Enzyme Assays: Essential Data handbook. He also has an interest in education and thus far has organised 46 drug discovery workshops since 2011 across the globe and trained 880 scientists.
References
- Estimated Research and Development Investment Needed to Bring a New Medicine to Market, 2009-2018. Wouters OJ, et al. JAMA. 2020, 323, 844-853.
- Gul S, Ed. Jayaprakash V, Castagnolo D, Özkay Y. Key concepts in assay development, screening and the properties of Lead and Candidate compounds, in Medicinal Chemistry of Neglected Tropical Diseases. Advances in The Design and Synthesis of Antimicrobial Agents. 2019, Chapter 1, p. 1–18, CRC Press, Taylor & Francis Group, U.S.A.
- 3-part Study to Assess Safety, Tolerability, Pharmacokinetics and Pharmacodynamics of EXS21546 – Full Text View – ClinicalTrials.gov [Internet]. Clinicaltrials.gov. 2021 [cited 9 September 2021]. Available from: https://clinicaltrials.gov/ct2/show/NCT04727138?term=exscientia&draw=2&rank=1
Related topics
Analysis, Artificial Intelligence, Drug Discovery, Informatics, Technology