A revolutionary deep learning model to predict undesired drug-drug interactions
Posted: 5 May 2022 | Ria Kakkad (Drug Target Review) | No comments yet
Scientists have developed a deep learning-based model trained on drug-induced gene expression signatures to predict drug-drug interactions.
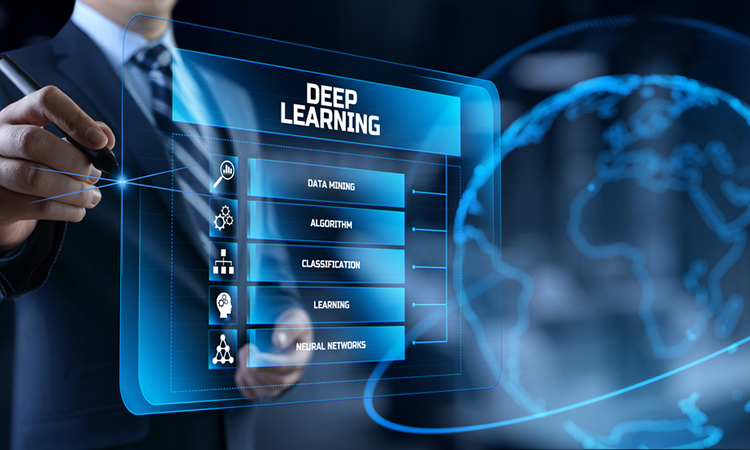
Using gene expression data, scientists from the Gwangju Institute of Science and Technology, South Korea have developed a deep learning model to predict how some drug-drug interactions (DDIs) can lead to adverse effects in the human body. The study was recently published in the Journal of Cheminformatics
Currently, computational models and neural network-based algorithms examine prior records of known drug interactions and identify the structures and side effects they are associated with. These approaches assume that similar drugs have similar interactions and identify drug combinations associated with similar adverse effects.
Although understanding the mechanisms of DDIs at a molecular level is essential to predict their undesirable effects, current models rely on structures and properties of drugs, with predictive range limited to previously observed interactions. They do not consider the effect of DDIs on genes and cell functionality.
The model consists of two parts: a feature generation model and a DDI prediction model. The feature generation model predicts a drug’s effect on gene expression by considering both the structure and properties of the drug while the DDI prediction model predicts various side effects resulting from drug combinations.
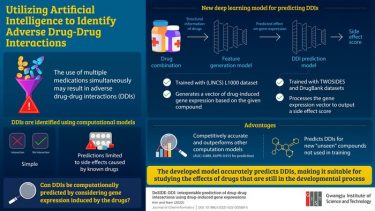
Researchers at the GIST Develop Deep Learning Model to Predict Adverse Drug-Drug Interactions. Using gene expression data, the new model can predict how some drug-drug interactions can lead to adverse effects in the human body
[Credit: Gwangju Institute of Science and Technology].
To explain the key features of this model Associate Professor Hojung Nam explained, “our model considers the effects of drugs on genes by utilising gene expression data, providing an explanation for why a certain pair of drugs cause DDIs. It can predict DDIs for currently approved drugs as well as for novel compounds. This way, the threats of polypharmacy can be resolved before new drugs are made available to the public.”
Furthermore, as all compounds do not have drug-treated gene expression signatures, this model uses a pre-trained compound generation model to generate expected drug-treated gene expressions.
“This model can discern potentially dangerous drug pairs, acting as a drug safety monitoring system. It can help researchers define the correct usage of the drug in the drug development phase,” concluded Nam.
Related topics
Artificial Intelligence, Bioinformatics, Drug Development, Drug Discovery, Drug Discovery Processes, Technology
Related organisations
the Gwangju Institute of Science and Technology
Related people
Associate Professor Hojung Nam