Novel machine learning technique to develop new drugs
Posted: 25 May 2022 | Ria Kakkad (Drug Target Review) | No comments yet
New machine learning models could propose new molecules that have specific properties which could fight certain diseases, doing in minutes what might take humans months to achieve manually.
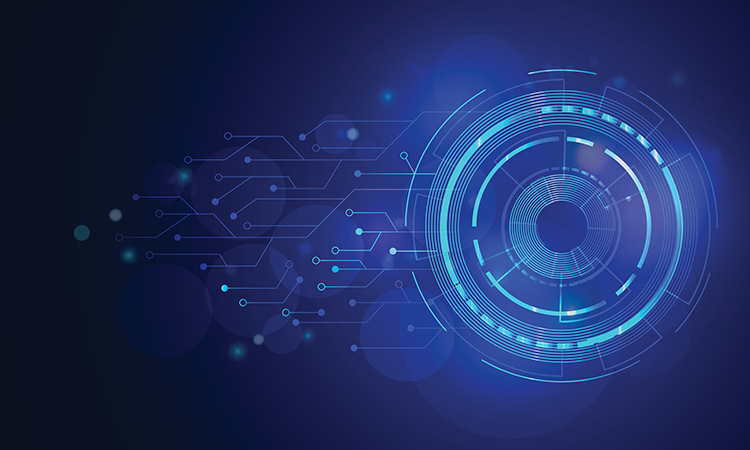
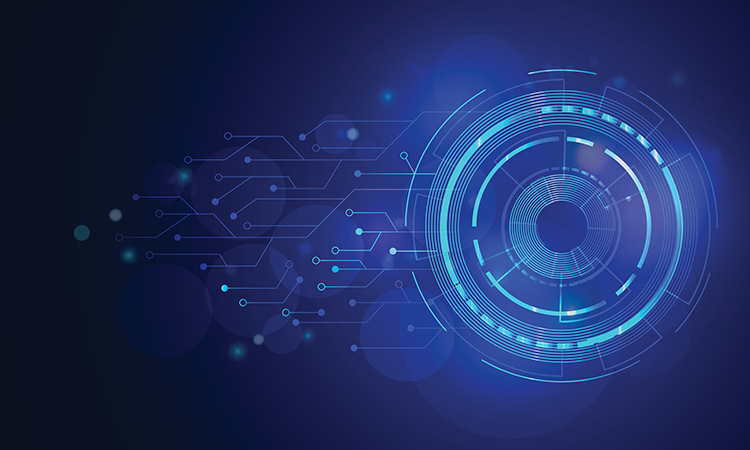
Pharmaceutical companies are using artificial intelligence (AI) to streamline the process of discovering new medicines. Machine learning models can propose new molecules that have specific properties which could fight certain diseases, doing in minutes what might take humans months to achieve manually. However, the models often suggest new molecular structures that are difficult or impossible to produce in a laboratory.
Researchers from Massachusetts Institute of Technology (MIT), US, have developed a new approach that constrains a machine learning model so it only suggests molecular structures that can be synthesised. The method guarantees that molecules are composed of materials that can be purchased and that the chemical reactions that occur between those materials follow the laws of chemistry.
When compared to other methods, their model proposed molecular structures that scored as high and sometimes better using popular evaluations but were guaranteed to be synthesisable. Their system also takes less than one second to propose a synthetic pathway, while other methods that separately propose molecules and then evaluate their synthesisability can take several minutes.
To create a molecular structure, the model simulates the process of synthesizing a molecule to ensure it can be produced. The model is given a set of viable building blocks, which are chemicals that can be purchased, and a list of valid chemical reactions to work with. These chemical reaction templates are hand-made by experts. Controlling these inputs by only allowing certain chemicals or specific reactions enables the researchers to limit how large the search space can be for a new molecule.
The machine learning model uses these inputs to build a tree by selecting building blocks and linking them through chemical reactions, one at a time, to build the final molecule. At each step, the molecule becomes more complex as additional chemicals and reactions are added. It also outputs both the final molecular structure and the tree of chemicals and reactions that would synthesis it.
To train their model, the researchers input a complete molecular structure and a set of building blocks and chemical reactions, and the model learns to create a tree that synthesises the molecule. After seeing hundreds of thousands of examples, the model learns to come up with these synthetic pathways on its own.
The trained model can be used for optimisation. Researchers define certain properties they want to achieve in a final molecule, given certain building blocks and chemical reaction templates, and the model proposes a synthesisable molecular structure.
They tested the model by evaluating how well it could reconstruct synthesisable molecules. It was able to reproduce 51 percent of these molecules and took less than a second to recreate each one.
When they used their model to propose molecules with specific properties, their method suggested higher quality molecular structures that had stronger binding affinities than those from other methods. This means the molecules would be better able to attach to a protein and block a certain activity, like stopping a virus from replicating.
Now that they have validated their approach, the team plans to continue improving the chemical reaction templates to further enhance the model’s performance. With additional templates, they can run more tests on certain disease targets and, eventually, apply the model to the drug discovery process.
Related topics
Artificial Intelligence, Bioinformatics, Disease Research, Lab Automation
Related organisations
Massachusetts Institute of Technology (MIT)