New machine learning technique to accelerate the process of drug screening
Posted: 15 August 2022 | Ria Kakkad (Drug Target Review) | No comments yet
Researchers have developed a machine learning method to quantitatively analyse and compare microscopy images of proteins.
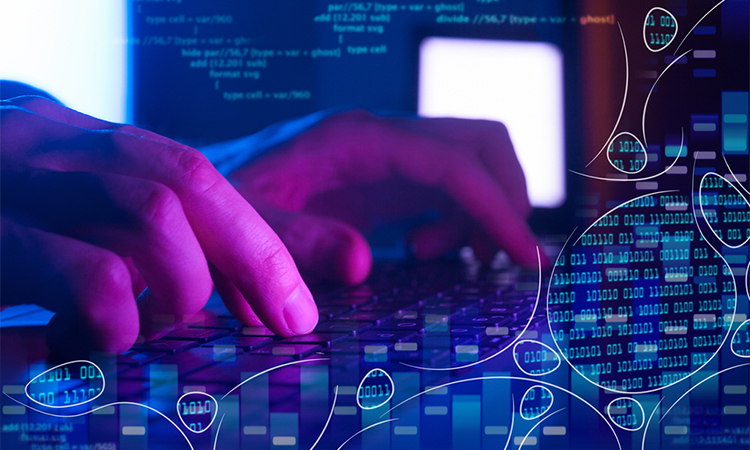
Scientists at Chan Zuckerberg Biohub, US have developed a machine learning method to quantitatively analyse and compare microscopy images of proteins. Their algorithm, dubbed “cytoself”, provides information on protein location and function within a cell. The findings, which were recently reported in Nature Methods, could quicken research time for cell biologists and eventually be used to accelerate the process of drug discovery and drug screening.
Cytoself is an example of self-supervised learning, meaning that humans do not teach the algorithm anything about the protein images, as is the case in supervised learning. “In supervised learning you have to teach the machine one by one with examples; it is a lot of work and very tedious,” said Hirofumi Kobayashi, lead author of the study. He also explained that if the machine is limited to the categories that humans teach it, it can introduce bias into the system.
“The machine transforms each protein image into a mathematical vector. So then you can start ranking images that look the same. We realised that by doing that we could predict, with high specificity, proteins that work together in the cell just by comparing their images, which was kind of surprising,” explained said Manuel Leonetti, a co-corresponding author of the study.
The researchers described that while there has been some previous work on protein images using self-supervised or unsupervised models, never has self-supervised learning been used so successfully on such a large dataset of over 1 million images covering over 1,300 proteins measured from live human cells.
“The question of what are all the possible ways a protein can localise in a cell – all the places it can be and all the kinds of combinations of places – is fundamental,” said Loic Royer, another co-corresponding author of the study. “Biologists have tried to establish all the possible places it can be, over decades, and all the possible structures within a cell. But that has always been done by humans looking at the data. The question is, how much have human limitations and biases made this process imperfect?”
Royer added: “As we have shown, machines can do it better than humans can do. They can find finer categories and see distinctions in the images that are extremely fine.”
The team’s next goal for cytoself is to track how small changes in protein localisation can be used to recognise different cellular states, for example, a normal cell versus a cancerous cell. This might hold the key to better understanding of many diseases and facilitate drug discovery.
“Drug screening is basically trial and error,” Kobayashi concluded. “But with cytoself, this is a big jump because you will not need to do experiments one-by-one with thousands of proteins. It is a low-cost method that could increase research speed by a lot.”
Related topics
Bioinformatics, Drug Discovery Processes, Drug Targets, Imaging
Related organisations
Chan Zuckerberg Biohub
Related people
Hirofumi Kobayashi, Loic Royer, Manuel Leonetti