Artificial intelligence model finds potential drug molecules a thousand times faster
Posted: 27 September 2022 | Ria Kakkad (Drug Target Review) | No comments yet
A geometric deep-learning model is faster and more accurate than state-of-the-art computational models, reducing the chances and costs of drug trial failures.
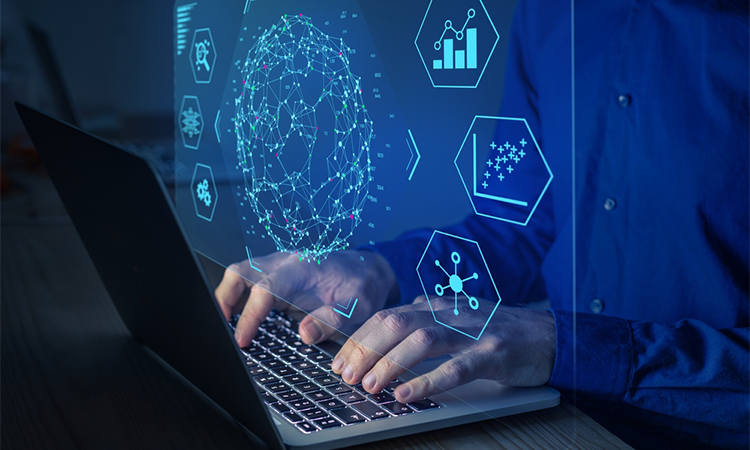
Researchers from the Massachusetts Institute of Technology (MIT), US, have developed a geometric deep-learning model called EquiBind that is 1,200 times faster than one of the fastest existing computational molecular docking models, QuickVina2-W, in successfully binding drug-like molecules to proteins. The paper was recently presented at the International Conference on Machine Learning (ICML).
Before drug development can even take place, drug researchers must find promising drug-like molecules that can bind or “dock” properly onto certain protein targets in a process known as drug discovery. After successfully docking to the protein, the binding drug, also known as the ligand, can stop a protein from functioning. If this happens to an essential protein of a bacterium, it can kill the bacterium, conferring protection to the human body.
However, the process of drug discovery can be costly both financially and computationally, with billions of dollars poured into the process and over a decade of development and testing before final approval from the US Food and Drug Administration.
The current computational process for finding promising drug candidate molecules goes like this: most state-of-the-art computational models rely upon heavy candidate sampling coupled with methods like scoring, ranking, and fine-tuning to get the best “fit” between the ligand and the protein.
Hannes Stärk, lead author of the paper, likens typical ligand-to-protein binding methodologies to “trying to fit a key into a lock with a lot of keyholes.” Typical models time-consumingly score each “fit” before choosing the best one. In contrast, EquiBind directly predicts the precise key location in a single step without prior knowledge of the protein’s target pocket, which is known as “blind docking.”
Unlike most models that require several attempts to find a favourable position for the ligand in the protein, EquiBind already has built-in geometric reasoning that helps the model learn the underlying physics of molecules and successfully generalise to make better predictions when encountering new, unseen data.
The researchers now hope to discuss ways to further improve the model.
Related topics
Artificial Intelligence, Bioinformatics, Drug Discovery
Related organisations
Massachusetts Institute of Technology (MIT), US Food and Drug Administration (FDA)
Related people
Hannes Stärk