Harnessing machine learning for early cancer detection in primary care
Posted: 25 July 2023 | Izzy Wood (Drug Target Review) | No comments yet
Machine learning (ML) presents a promising opportunity to revolutionize early cancer detection in primary care, addressing the challenges associated with diagnostic errors and improving patient outcomes. The potential of ML in this field is highlighted in a recent paper published in Oncoscience
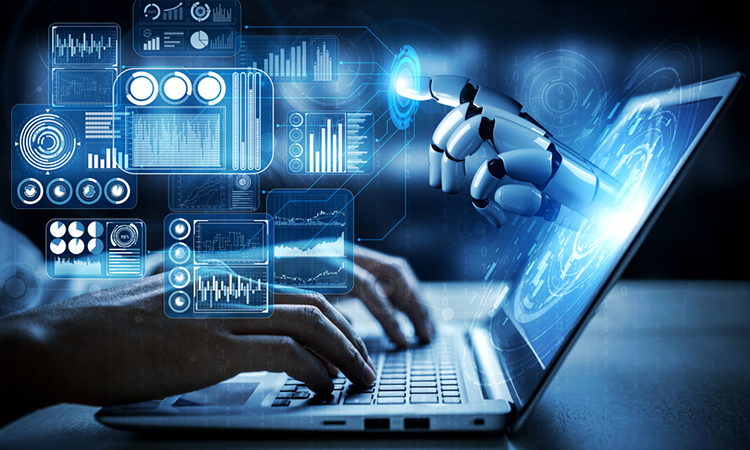
“Machine learning has the potential to transform early cancer detection in primary care.”
Cancer remains a significant global health burden, and early detection plays a crucial role in improving patient outcomes. Primary care settings serve as frontline gatekeepers, providing an opportunity for early detection through symptom assessment and targeted screening. However, detecting early-stage cancer and identifying individuals at high risk can be challenging due to the complexity and subtlety of symptoms.
In recent years, the emergence of machine learning (ML) techniques has shown promise in revolutionizing early detection efforts.
A new paper was published in Oncoscience (Volume 10) entitled: Transforming early cancer detection in primary care: harnessing the power of machine learning, outlines potential of ML in enhancing early cancer detection in primary care. Researchers Elinor Nemlander, Marcela Ewing, Axel C Carlsson and Andreas Rosenblad from Karolinska Institutet and the Academic Primary Health Care Centre at Region Stockholm explore this potential.
The challenging nature of early detection is revealed by diagnostic errors in primary care, with cancer being one of the most frequently missed or delayed diagnoses.
While ML holds big potential, several challenges need to be addressed. Data privacy, algorithm transparency, and the potential for biases in algorithm development are critical concerns. It is essential to validate algorithms across diverse populations and collaborate between researchers, clinicians, and policymakers to establish standardized protocols and ensure equitable access to these innovative tools. Additionally, the choice of the appropriate method, whether ML or traditional methods, should be determined by the specific task at hand, as no single approach is universally superior
“ML has the potential to transform early cancer detection in primary care by leveraging extensive patient data and improving risk stratification and pre-diagnostic accuracy, hopefully saving lives. However, responsible and equitable implementation of ML models requires careful attention to ethical considerations, collaboration, and validation across diverse populations.”
Related topics
Bioinformatics, Machine learning, Oncology
Related conditions
Cancer
Related organisations
Academic Primary Health Care Centre at Region Stockholm, Karolinska Institutet, Oncoscience
Related people
Andreas Rosenblad, Axel C. Carlsson, Elinor Nemlander, Marcela Ewing