Novel prediction tool accurately determines disease stage
Posted: 29 January 2024 | Drug Target Review | No comments yet
A new learning-based framework enables patients and caregivers to predict the timing of any of the five clinical groups of AD development.
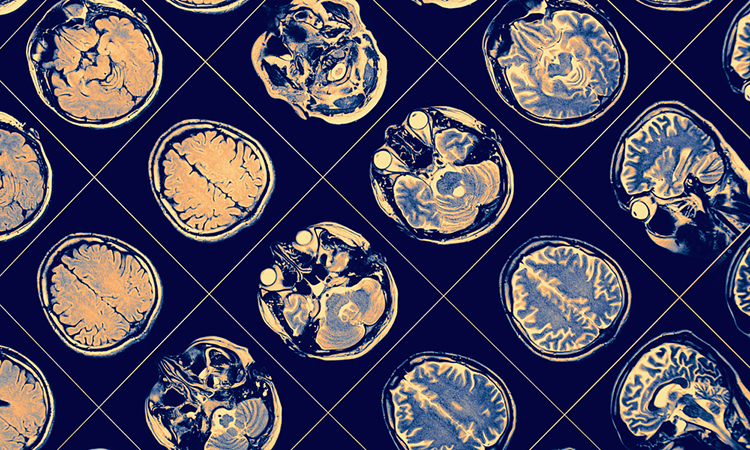
Scientists at The University of Texas at Arlington (UTA) have created a new learning-based framework to help Alzheimer’s patients accurately determine at what stage they are within the disease-development spectrum. This will enable them to predict the timing of the later stages, facilitating a plan for future care.
According to the World Health Organisation, about 55 million people are living with dementia globally. Its most common form is Alzheimer’s disease (AD), causing not only devastating physical effects, but social and economic ones too. Its symptoms worsen over time so it is crucial that patients and caregivers can prepare for extra support as the disease progresses.
Dr Dajiang Zhu, an associate professor in computer science and engineering at UTA, explained: “For decades, a variety of predictive approaches have been proposed and evaluated in terms of the predictive capability for Alzheimer’s disease and its precursor, mild cognitive impairment…Many of these earlier prediction tools overlooked the continuous nature of how Alzheimer’s disease develops and the transition stages of the disease.”
Dr Zhu’s Medical Imaging and Neuroscientific Discovery research lab and Dr Li Wang, UTA associate professor in mathematics, developed a novel learning-based embedding framework. It codes the various stages of AD development in a process they named a “disease-embedding tree” (DETree).
The DETree predicts any of the five clinical groups of AD development efficiently and accurately and can provide more in-depth status information by projecting where within the patient it will be as the disease progresses.
The researchers used data from 266 individuals with AD from the multicentre Alzheimer’s Disease Neuroimaging Initiative to test their DETree framework. Then, the DETree strategy results were compared with other widely used methods for predicting Alzheimer’s disease progression, and the experiment was repeated several times using machine learning (ML)-methods to validate the technique.
“We know individuals living with Alzheimer’s disease often develop worsening symptoms at very different rates,” Dr Zhu said. “We’re heartened that our new framework is more accurate than the other prediction models available, which we hope will help patients and their families better plan for the uncertainties of this complicated and devastating disease.”
The team believes that the DETree framework has the potential to help predict the progression of other diseases that have multiple clinical stages of development, such as Parkinson’s disease (PD), Huntington’s disease (HD), and Creutzfeldt-Jakob disease (CJD).
This work was supported by over $2 million in grants from the National Institutes of Health (NIH) and the National Institute on Aging (NIA).
The study was published in Pharmacological Research.
Related topics
Informatics, Neurosciences
Related conditions
Alzheimer's disease (AD), Creutzfeldt-Jakob disease (CJD), Huntington's disease (HD), Parkinson's disease (PD)
Related organisations
The University of Texas at Arlington (UTA)
Related people
Dr Dajiang Zhu (UTA), Dr Li Wang (UTA)