Discovery of statistical method identifies cancer predisposition
Posted: 12 July 2018 | Drug Target Review | No comments yet
New statistical method, ALFRED, could identify predisposition for cancers by using cancer genome data sets to identify risk variants.
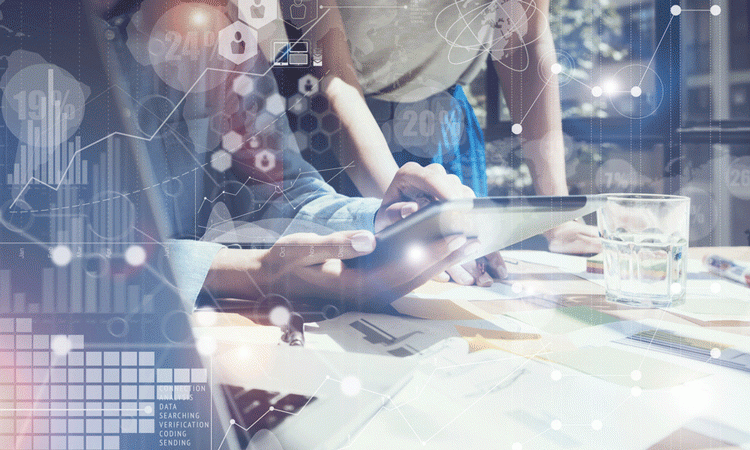
Researchers at the Centre for Genomic Regulation (CRG) in Barcelona developed a statistical method to identify cancer predisposition genes from tumour sequencing data. This developed statistical method, ALFRED, allows researchers to find risk variants without control groups, and as such studies may no longer need healthy groups of individuals as comparisons for cancer patients. The team also identified 13 possible cancer predisposition genes, with 10 of them being new.
Solip Park, the first author, said, “Our computational method uses an old idea that cancer genes often require ‘two hits’ before they cause cancer. We developed a method that allows us to systematically identify these genes from existing cancer genome datasets.”
“We applied our method to the genome sequences of more than 10,000 cancer patients with 30 different tumour types and identified known and new possible cancer predisposition genes that have the potential to contribute substantially to cancer risk,” said Professor Ben Lehner, study lead.
“Our results show that the new cancer predisposition genes may have an important role in many types of cancer. For example, they were associated with 14 percent of ovarian tumours, 7 percent of breast tumours and to about 1 in 50 of all cancers. For example, inherited variants in one of the newly-proposed risk genes – NSD1 – may be implicated in at least 3 out of 1,000 cancer patients”, clarifies Frank Supek, group leader of the Genome Data Science laboratory at the Institute for Research in Biomedicine (IRB Barcelona).
“We managed to develop and test a new statistical method that hopefully will improve our understanding of cancer genomics and will contribute to cancer research, diagnostics and prevention just by using public data,” states Park.
He added, “now we have a powerful tool to detect new cancer predisposition genes and, consequently, to contribute to improving cancer diagnosis and prevention in the future.”
The team worked with data from several genome studies around the world, including The Cancer Genome Atlas (TCGA) project.
Prof Lehner said, “Our work highlights how important it is to share genomic data. It is a success story for how being open is far more efficient and has a multiplier effect. We combined data from many different projects and by applying a new computational method were able to identify important cancer genes that were not identified by the original studies. Many patient groups lobby for better sharing of genomic data because it is only by comparing data across hospitals, countries and diseases that we can obtain a deep understanding of many rare and common diseases. Unfortunately, many researchers still do not share their data and this is something we need to actively change as a society”.
The study on this statistical method was published in Nature Communications.
Related topics
Analytical Techniques, Artificial Intelligence, Informatics
Related conditions
Cancer
Related organisations
Centre for Genomic Regulation, Institute for Research in Biomedicine
Related people
Fran Supek, Juan de la Cierva, Professor Ben Lehner, Solip Park