Imaging and machine learning system created to discover tuberculosis drugs
Posted: 24 July 2020 | Victoria Rees (Drug Target Review) | No comments yet
Researchers have developed MorphEUS, a technology to identify new drugs that combat M. tuberculosis by revealing how compounds destroy the bacteria.
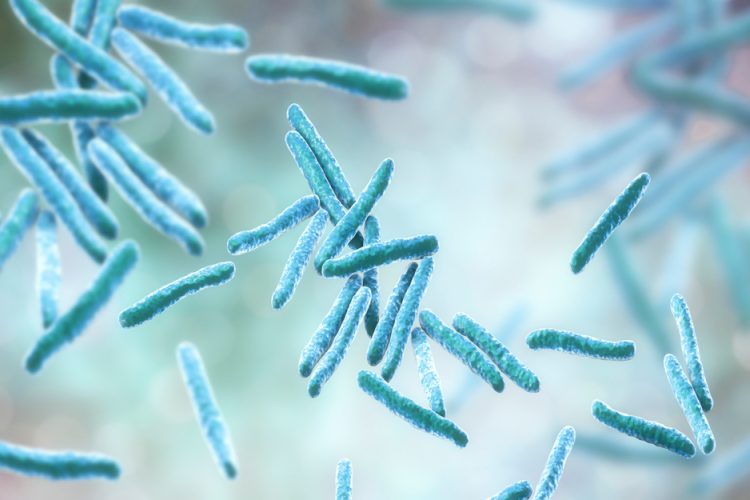
A new technology that combines high throughput imaging and machine learning has been developed, which the researchers say could speed up the discovery of drugs to fight tuberculosis (TB).
Conducted at Tufts University, US, the study unveils the new technology named Morphological Evaluation and Understanding of drug Stress (MorphEUS). According to the researchers, MorphEUS provides a rapid, efficient and cost-effective way to determine how specific compounds act to destroy Mycobcterium tuberculosis, the bacterium that causes tuberculosis.
“We urgently need shorter, more effective TB therapies and MorphEUS enables us to screen through drug candidates, see how they actually affect the cell and learn which drugs have unique ways to kill the M. tuberculosis,” said Bree Aldridge, associate professor of molecular biology and microbiology at Tufts University School of Medicine and senior author of the paper.
Aldridge and colleagues applied MorphEUS to 34 currently available antibiotics for which modes of action were already established and three non-commercial compounds. They found that MorphEUS categorised the drugs correctly 94 percent of the time. In the remaining instances, MorphEUS identified previously unknown target pathways.
“We found that conventional morphological profiling approaches did not work with M. tuberculosis, because the bacterium’s inherent response to treatment was extremely variable and changes in morphology were much less obvious than in bacteria like E. coli,” said Trever Smith, co-first author on the paper and a postdoctoral researcher in the Aldridge laboratory.
The researchers explained that MorphEUS harnesses this variation by incorporating measurements of heterogeneity itself into morphological profiles and combining this enhanced feature set with machine learning and other complex analytical tools. Network webs and matrices visualise the data analysis. For example, much of the heterogeneity in staining patterns in M. tuberculosis is due to its thick, complex cell wall. There is increased staining and less variation in staining patterns when M. tuberculosis is treated with cell-wall targeting antibiotics compared with other classes of antibiotics.
“With MorphEUS, we used the distribution of staining across a large number of bacilli to learn how each drug acts on M. tuberculosis,” said Aldridge. “Similarly, we looked at staining intensity and the spread of that brightness across thousands of cells to identify more subtle patterns.”
MorphEUS, like all cytological profiling techniques, is data-driven and based on classification among a pool of other profiles. It requires multiple representative profiles from M. tuberculosis treated with compounds known to target the same broad cellular target. As the drug set expands, the accuracy and resolution of MorphEUS will improve, the researchers say.
MorphEUS can also determine if drugs have off-target or secondary effects that are otherwise hard to identify. Such complex mechanisms of drug action can be key in designing multidrug therapies.
“We expect that the success of MorphEUS in profiling drug action in an organism like M. tuberculosis with significant inherent heterogeneity and subtle cytological responsiveness will make it useful in other pathogens and cell types,” said Aldridge.
However, the researchers highlight that MorphEUS is limited in its ability to identify target pathways of compounds with novel mechanisms of action that are unlike the other profiled drugs in the reference set.
The results can be found in Proceedings of the National Academies of Sciences.
Related topics
Drug Discovery, Drug Discovery Processes, Informatics, Microbiology, Screening, Target Validation, Technology
Related conditions
M. tuberculosis, Tuberculosis
Related organisations
Tufts University
Related people
Bree Aldridge